What’s New¶
These are new features and improvements of note in each release.
v0.17.1 (November 21, 2015)¶
Note
We are proud to announce that pandas has become a sponsored project of the (NUMFocus organization). This will help ensure the success of development of pandas as a world-class open-source project.
This is a minor bug-fix release from 0.17.0 and includes a large number of bug fixes along several new features, enhancements, and performance improvements. We recommend that all users upgrade to this version.
Highlights include:
- Support for Conditional HTML Formatting, see here
- Releasing the GIL on the csv reader & other ops, see here
- Fixed regression in DataFrame.drop_duplicates from 0.16.2, causing incorrect results on integer values (GH11376)
What’s new in v0.17.1
New features¶
Conditional HTML Formatting¶
Warning
This is a new feature and is under active development. We’ll be adding features an possibly making breaking changes in future releases. Feedback is welcome.
We’ve added experimental support for conditional HTML formatting: the visual styling of a DataFrame based on the data. The styling is accomplished with HTML and CSS. Acesses the styler class with the pandas.DataFrame.style, attribute, an instance of Styler with your data attached.
Here’s a quick example:
In [1]: np.random.seed(123) In [2]: df = DataFrame(np.random.randn(10, 5), columns=list('abcde')) In [3]: html = df.style.background_gradient(cmap='viridis', low=.5)
We can render the HTML to get the following table.
a | b | c | d | e | |
---|---|---|---|---|---|
0 | -1.085631 | 0.997345 | 0.282978 | -1.506295 | -0.5786 |
1 | 1.651437 | -2.426679 | -0.428913 | 1.265936 | -0.86674 |
2 | -0.678886 | -0.094709 | 1.49139 | -0.638902 | -0.443982 |
3 | -0.434351 | 2.20593 | 2.186786 | 1.004054 | 0.386186 |
4 | 0.737369 | 1.490732 | -0.935834 | 1.175829 | -1.253881 |
5 | -0.637752 | 0.907105 | -1.428681 | -0.140069 | -0.861755 |
6 | -0.255619 | -2.798589 | -1.771533 | -0.699877 | 0.927462 |
7 | -0.173636 | 0.002846 | 0.688223 | -0.879536 | 0.283627 |
8 | -0.805367 | -1.727669 | -0.3909 | 0.573806 | 0.338589 |
9 | -0.01183 | 2.392365 | 0.412912 | 0.978736 | 2.238143 |
Styler interacts nicely with the Jupyter Notebook. See the documentation for more.
Enhancements¶
DatetimeIndex now supports conversion to strings with astype(str) (GH10442)
Support for compression (gzip/bz2) in pandas.DataFrame.to_csv() (GH7615)
pd.read_* functions can now also accept pathlib.Path, or py._path.local.LocalPath objects for the filepath_or_buffer argument. (GH11033) - The DataFrame and Series functions .to_csv(), .to_html() and .to_latex() can now handle paths beginning with tildes (e.g. ~/Documents/) (GH11438)
DataFrame now uses the fields of a namedtuple as columns, if columns are not supplied (GH11181)
DataFrame.itertuples() now returns namedtuple objects, when possible. (GH11269, GH11625)
Added axvlines_kwds to parallel coordinates plot (GH10709)
Option to .info() and .memory_usage() to provide for deep introspection of memory consumption. Note that this can be expensive to compute and therefore is an optional parameter. (GH11595)
In [4]: df = DataFrame({'A' : ['foo']*1000}) In [5]: df['B'] = df['A'].astype('category') # shows the '+' as we have object dtypes In [6]: df.info() <class 'pandas.core.frame.DataFrame'> Int64Index: 1000 entries, 0 to 999 Data columns (total 2 columns): A 1000 non-null object B 1000 non-null category dtypes: category(1), object(1) memory usage: 12.7+ KB # we have an accurate memory assessment (but can be expensive to compute this) In [7]: df.info(memory_usage='deep') <class 'pandas.core.frame.DataFrame'> Int64Index: 1000 entries, 0 to 999 Data columns (total 2 columns): A 1000 non-null object B 1000 non-null category dtypes: category(1), object(1) memory usage: 36.2 KB
Index now has a fillna method (GH10089)
In [8]: pd.Index([1, np.nan, 3]).fillna(2) Out[8]: Float64Index([1.0, 2.0, 3.0], dtype='float64')
Series of type category now make .str.<...> and .dt.<...> accessor methods / properties available, if the categories are of that type. (GH10661)
In [9]: s = pd.Series(list('aabb')).astype('category') In [10]: s Out[10]: 0 a 1 a 2 b 3 b dtype: category Categories (2, object): [a, b] In [11]: s.str.contains("a") Out[11]: 0 True 1 True 2 False 3 False dtype: bool In [12]: date = pd.Series(pd.date_range('1/1/2015', periods=5)).astype('category') In [13]: date Out[13]: 0 2015-01-01 1 2015-01-02 2 2015-01-03 3 2015-01-04 4 2015-01-05 dtype: category Categories (5, datetime64[ns]): [2015-01-01, 2015-01-02, 2015-01-03, 2015-01-04, 2015-01-05] In [14]: date.dt.day Out[14]: 0 1 1 2 2 3 3 4 4 5 dtype: int64
pivot_table now has a margins_name argument so you can use something other than the default of ‘All’ (GH3335)
Implement export of datetime64[ns, tz] dtypes with a fixed HDF5 store (GH11411)
Pretty printing sets (e.g. in DataFrame cells) now uses set literal syntax ({x, y}) instead of Legacy Python syntax (set([x, y])) (GH11215)
Improve the error message in pandas.io.gbq.to_gbq() when a streaming insert fails (GH11285) and when the DataFrame does not match the schema of the destination table (GH11359)
API changes¶
- raise NotImplementedError in Index.shift for non-supported index types (GH8038)
- min and max reductions on datetime64 and timedelta64 dtyped series now result in NaT and not nan (GH11245).
- Indexing with a null key will raise a TypeError, instead of a ValueError (GH11356)
- Series.ptp will now ignore missing values by default (GH11163)
Performance Improvements¶
- Checking monotonic-ness before sorting on an index (GH11080)
- Series.dropna performance improvement when its dtype can’t contain NaN (GH11159)
- Release the GIL on most datetime field operations (e.g. DatetimeIndex.year, Series.dt.year), normalization, and conversion to and from Period, DatetimeIndex.to_period and PeriodIndex.to_timestamp (GH11263)
- Release the GIL on some rolling algos: rolling_median, rolling_mean, rolling_max, rolling_min, rolling_var, rolling_kurt, rolling_skew (GH11450)
- Release the GIL when reading and parsing text files in read_csv, read_table (GH11272)
- Improved performance of rolling_median (GH11450)
- Improved performance of to_excel (GH11352)
- Performance bug in repr of Categorical categories, which was rendering the strings before chopping them for display (GH11305)
- Performance improvement in Categorical.remove_unused_categories, (GH11643).
- Improved performance of Series constructor with no data and DatetimeIndex (GH11433)
- Improved performance of shift, cumprod, and cumsum with groupby (GH4095)
Bug Fixes¶
- SparseArray.__iter__() now does not cause PendingDeprecationWarning in Python 3.5 (GH11622)
- Regression from 0.16.2 for output formatting of long floats/nan, restored in (GH11302)
- Series.sort_index() now correctly handles the inplace option (GH11402)
- Incorrectly distributed .c file in the build on PyPi when reading a csv of floats and passing na_values=<a scalar> would show an exception (GH11374)
- Bug in .to_latex() output broken when the index has a name (GH10660)
- Bug in HDFStore.append with strings whose encoded length exceded the max unencoded length (GH11234)
- Bug in merging datetime64[ns, tz] dtypes (GH11405)
- Bug in HDFStore.select when comparing with a numpy scalar in a where clause (GH11283)
- Bug in using DataFrame.ix with a multi-index indexer (GH11372)
- Bug in date_range with ambigous endpoints (GH11626)
- Prevent adding new attributes to the accessors .str, .dt and .cat. Retrieving such a value was not possible, so error out on setting it. (GH10673)
- Bug in tz-conversions with an ambiguous time and .dt accessors (GH11295)
- Bug in output formatting when using an index of ambiguous times (GH11619)
- Bug in comparisons of Series vs list-likes (GH11339)
- Bug in DataFrame.replace with a datetime64[ns, tz] and a non-compat to_replace (GH11326, GH11153)
- Bug in isnull where numpy.datetime64('NaT') in a numpy.array was not determined to be null(GH11206)
- Bug in list-like indexing with a mixed-integer Index (GH11320)
- Bug in pivot_table with margins=True when indexes are of Categorical dtype (GH10993)
- Bug in DataFrame.plot cannot use hex strings colors (GH10299)
- Regression in DataFrame.drop_duplicates from 0.16.2, causing incorrect results on integer values (GH11376)
- Bug in pd.eval where unary ops in a list error (GH11235)
- Bug in squeeze() with zero length arrays (GH11230, GH8999)
- Bug in describe() dropping column names for hierarchical indexes (GH11517)
- Bug in DataFrame.pct_change() not propagating axis keyword on .fillna method (GH11150)
- Bug in .to_csv() when a mix of integer and string column names are passed as the columns parameter (GH11637)
- Bug in indexing with a range, (GH11652)
- Bug in inference of numpy scalars and preserving dtype when setting columns (GH11638)
- Bug in to_sql using unicode column names giving UnicodeEncodeError with (GH11431).
- Fix regression in setting of xticks in plot (GH11529).
- Bug in holiday.dates where observance rules could not be applied to holiday and doc enhancement (GH11477, GH11533)
- Fix plotting issues when having plain Axes instances instead of SubplotAxes (GH11520, GH11556).
- Bug in DataFrame.to_latex() produces an extra rule when header=False (GH7124)
- Bug in df.groupby(...).apply(func) when a func returns a Series containing a new datetimelike column (GH11324)
- Bug in pandas.json when file to load is big (GH11344)
- Bugs in to_excel with duplicate columns (GH11007, GH10982, GH10970)
- Fixed a bug that prevented the construction of an empty series of dtype datetime64[ns, tz] (GH11245).
- Bug in read_excel with multi-index containing integers (GH11317)
- Bug in to_excel with openpyxl 2.2+ and merging (GH11408)
- Bug in DataFrame.to_dict() produces a np.datetime64 object instead of Timestamp when only datetime is present in data (GH11327)
- Bug in DataFrame.corr() raises exception when computes Kendall correlation for DataFrames with boolean and not boolean columns (GH11560)
- Bug in the link-time error caused by C inline functions on FreeBSD 10+ (with clang) (GH10510)
- Bug in DataFrame.to_csv in passing through arguments for formatting MultiIndexes, including date_format (GH7791)
- Bug in DataFrame.join() with how='right' producing a TypeError (GH11519)
- Bug in Series.quantile with empty list results has Index with object dtype (GH11588)
- Bug in pd.merge results in empty Int64Index rather than Index(dtype=object) when the merge result is empty (GH11588)
- Bug in Categorical.remove_unused_categories when having NaN values (GH11599)
- Bug in DataFrame.to_sparse() loses column names for MultiIndexes (GH11600)
- Bug in DataFrame.round() with non-unique column index producing a Fatal Python error (GH11611)
- Bug in DataFrame.round() with decimals being a non-unique indexed Series producing extra columns (GH11618)
v0.17.0 (October 9, 2015)¶
This is a major release from 0.16.2 and includes a small number of API changes, several new features, enhancements, and performance improvements along with a large number of bug fixes. We recommend that all users upgrade to this version.
Warning
pandas >= 0.17.0 will no longer support compatibility with Python version 3.2 (GH9118)
Warning
The pandas.io.data package is deprecated and will be replaced by the pandas-datareader package. This will allow the data modules to be independently updated to your pandas installation. The API for pandas-datareader v0.1.1 is exactly the same as in pandas v0.17.0 (GH8961, GH10861).
After installing pandas-datareader, you can easily change your imports:
from pandas.io import data, wb
becomes
from pandas_datareader import data, wb
Highlights include:
- Release the Global Interpreter Lock (GIL) on some cython operations, see here
- Plotting methods are now available as attributes of the .plot accessor, see here
- The sorting API has been revamped to remove some long-time inconsistencies, see here
- Support for a datetime64[ns] with timezones as a first-class dtype, see here
- The default for to_datetime will now be to raise when presented with unparseable formats, previously this would return the original input. Also, date parse functions now return consistent results. See here
- The default for dropna in HDFStore has changed to False, to store by default all rows even if they are all NaN, see here
- Datetime accessor (dt) now supports Series.dt.strftime to generate formatted strings for datetime-likes, and Series.dt.total_seconds to generate each duration of the timedelta in seconds. See here
- Period and PeriodIndex can handle multiplied freq like 3D, which corresponding to 3 days span. See here
- Development installed versions of pandas will now have PEP440 compliant version strings (GH9518)
- Development support for benchmarking with the Air Speed Velocity library (GH8361)
- Support for reading SAS xport files, see here
- Documentation comparing SAS to pandas, see here
- Removal of the automatic TimeSeries broadcasting, deprecated since 0.8.0, see here
- Display format with plain text can optionally align with Unicode East Asian Width, see here
- Compatibility with Python 3.5 (GH11097)
- Compatibility with matplotlib 1.5.0 (GH11111)
Check the API Changes and deprecations before updating.
What’s new in v0.17.0
- New features
- Datetime with TZ
- Releasing the GIL
- Plot submethods
- Additional methods for dt accessor
- Period Frequency Enhancement
- Support for SAS XPORT files
- Support for Math Functions in .eval()
- Changes to Excel with MultiIndex
- Google BigQuery Enhancements
- Display Alignment with Unicode East Asian Width
- Other enhancements
- Backwards incompatible API changes
- Changes to sorting API
- Changes to to_datetime and to_timedelta
- Changes to Index Comparisons
- Changes to Boolean Comparisons vs. None
- HDFStore dropna behavior
- Changes to display.precision option
- Changes to Categorical.unique
- Changes to bool passed as header in Parsers
- Other API Changes
- Deprecations
- Removal of prior version deprecations/changes
- Performance Improvements
- Bug Fixes
New features¶
Datetime with TZ¶
We are adding an implementation that natively supports datetime with timezones. A Series or a DataFrame column previously could be assigned a datetime with timezones, and would work as an object dtype. This had performance issues with a large number rows. See the docs for more details. (GH8260, GH10763, GH11034).
The new implementation allows for having a single-timezone across all rows, with operations in a performant manner.
In [1]: df = DataFrame({'A' : date_range('20130101',periods=3),
...: 'B' : date_range('20130101',periods=3,tz='US/Eastern'),
...: 'C' : date_range('20130101',periods=3,tz='CET')})
...:
In [2]: df
Out[2]:
A B C
0 2013-01-01 2013-01-01 00:00:00-05:00 2013-01-01 00:00:00+01:00
1 2013-01-02 2013-01-02 00:00:00-05:00 2013-01-02 00:00:00+01:00
2 2013-01-03 2013-01-03 00:00:00-05:00 2013-01-03 00:00:00+01:00
In [3]: df.dtypes
Out[3]:
A datetime64[ns]
B datetime64[ns, US/Eastern]
C datetime64[ns, CET]
dtype: object
In [4]: df.B
Out[4]:
0 2013-01-01 00:00:00-05:00
1 2013-01-02 00:00:00-05:00
2 2013-01-03 00:00:00-05:00
Name: B, dtype: datetime64[ns, US/Eastern]
In [5]: df.B.dt.tz_localize(None)
Out[5]:
0 2013-01-01
1 2013-01-02
2 2013-01-03
Name: B, dtype: datetime64[ns]
This uses a new-dtype representation as well, that is very similar in look-and-feel to its numpy cousin datetime64[ns]
In [6]: df['B'].dtype
Out[6]: datetime64[ns, US/Eastern]
In [7]: type(df['B'].dtype)
Out[7]: pandas.core.dtypes.DatetimeTZDtype
Note
There is a slightly different string repr for the underlying DatetimeIndex as a result of the dtype changes, but functionally these are the same.
Previous Behavior:
In [1]: pd.date_range('20130101',periods=3,tz='US/Eastern')
Out[1]: DatetimeIndex(['2013-01-01 00:00:00-05:00', '2013-01-02 00:00:00-05:00',
'2013-01-03 00:00:00-05:00'],
dtype='datetime64[ns]', freq='D', tz='US/Eastern')
In [2]: pd.date_range('20130101',periods=3,tz='US/Eastern').dtype
Out[2]: dtype('<M8[ns]')
New Behavior:
In [8]: pd.date_range('20130101',periods=3,tz='US/Eastern')
Out[8]:
DatetimeIndex(['2013-01-01 00:00:00-05:00', '2013-01-02 00:00:00-05:00',
'2013-01-03 00:00:00-05:00'],
dtype='datetime64[ns, US/Eastern]', freq='D')
In [9]: pd.date_range('20130101',periods=3,tz='US/Eastern').dtype
Out[9]: datetime64[ns, US/Eastern]
Releasing the GIL¶
We are releasing the global-interpreter-lock (GIL) on some cython operations. This will allow other threads to run simultaneously during computation, potentially allowing performance improvements from multi-threading. Notably groupby, nsmallest, value_counts and some indexing operations benefit from this. (GH8882)
For example the groupby expression in the following code will have the GIL released during the factorization step, e.g. df.groupby('key') as well as the .sum() operation.
N = 1000000
ngroups = 10
df = DataFrame({'key' : np.random.randint(0,ngroups,size=N),
'data' : np.random.randn(N) })
df.groupby('key')['data'].sum()
Releasing of the GIL could benefit an application that uses threads for user interactions (e.g. QT), or performing multi-threaded computations. A nice example of a library that can handle these types of computation-in-parallel is the dask library.
Plot submethods¶
The Series and DataFrame .plot() method allows for customizing plot types by supplying the kind keyword arguments. Unfortunately, many of these kinds of plots use different required and optional keyword arguments, which makes it difficult to discover what any given plot kind uses out of the dozens of possible arguments.
To alleviate this issue, we have added a new, optional plotting interface, which exposes each kind of plot as a method of the .plot attribute. Instead of writing series.plot(kind=<kind>, ...), you can now also use series.plot.<kind>(...):
In [10]: df = pd.DataFrame(np.random.rand(10, 2), columns=['a', 'b'])
In [11]: df.plot.bar()
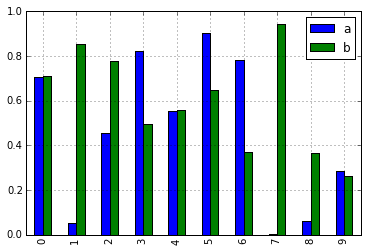
As a result of this change, these methods are now all discoverable via tab-completion:
In [12]: df.plot.<TAB>
df.plot.area df.plot.barh df.plot.density df.plot.hist df.plot.line df.plot.scatter
df.plot.bar df.plot.box df.plot.hexbin df.plot.kde df.plot.pie
Each method signature only includes relevant arguments. Currently, these are limited to required arguments, but in the future these will include optional arguments, as well. For an overview, see the new Plotting API documentation.
Additional methods for dt accessor¶
strftime¶
We are now supporting a Series.dt.strftime method for datetime-likes to generate a formatted string (GH10110). Examples:
# DatetimeIndex
In [13]: s = pd.Series(pd.date_range('20130101', periods=4))
In [14]: s
Out[14]:
0 2013-01-01
1 2013-01-02
2 2013-01-03
3 2013-01-04
dtype: datetime64[ns]
In [15]: s.dt.strftime('%Y/%m/%d')
Out[15]:
0 2013/01/01
1 2013/01/02
2 2013/01/03
3 2013/01/04
dtype: object
# PeriodIndex
In [16]: s = pd.Series(pd.period_range('20130101', periods=4))
In [17]: s
Out[17]:
0 2013-01-01
1 2013-01-02
2 2013-01-03
3 2013-01-04
dtype: object
In [18]: s.dt.strftime('%Y/%m/%d')
Out[18]:
0 2013/01/01
1 2013/01/02
2 2013/01/03
3 2013/01/04
dtype: object
The string format is as the python standard library and details can be found here
total_seconds¶
pd.Series of type timedelta64 has new method .dt.total_seconds() returning the duration of the timedelta in seconds (GH10817)
# TimedeltaIndex
In [19]: s = pd.Series(pd.timedelta_range('1 minutes', periods=4))
In [20]: s
Out[20]:
0 0 days 00:01:00
1 1 days 00:01:00
2 2 days 00:01:00
3 3 days 00:01:00
dtype: timedelta64[ns]
In [21]: s.dt.total_seconds()
Out[21]:
0 60
1 86460
2 172860
3 259260
dtype: float64
Period Frequency Enhancement¶
Period, PeriodIndex and period_range can now accept multiplied freq. Also, Period.freq and PeriodIndex.freq are now stored as a DateOffset instance like DatetimeIndex, and not as str (GH7811)
A multiplied freq represents a span of corresponding length. The example below creates a period of 3 days. Addition and subtraction will shift the period by its span.
In [22]: p = pd.Period('2015-08-01', freq='3D')
In [23]: p
Out[23]: Period('2015-08-01', '3D')
In [24]: p + 1
Out[24]: Period('2015-08-04', '3D')
In [25]: p - 2
Out[25]: Period('2015-07-26', '3D')
In [26]: p.to_timestamp()
Out[26]: Timestamp('2015-08-01 00:00:00')
In [27]: p.to_timestamp(how='E')
Out[27]: Timestamp('2015-08-03 00:00:00')
You can use the multiplied freq in PeriodIndex and period_range.
In [28]: idx = pd.period_range('2015-08-01', periods=4, freq='2D')
In [29]: idx
Out[29]: PeriodIndex(['2015-08-01', '2015-08-03', '2015-08-05', '2015-08-07'], dtype='int64', freq='2D')
In [30]: idx + 1
Out[30]: PeriodIndex(['2015-08-03', '2015-08-05', '2015-08-07', '2015-08-09'], dtype='int64', freq='2D')
Support for SAS XPORT files¶
read_sas() provides support for reading SAS XPORT format files. (GH4052).
df = pd.read_sas('sas_xport.xpt')
It is also possible to obtain an iterator and read an XPORT file incrementally.
for df in pd.read_sas('sas_xport.xpt', chunksize=10000)
do_something(df)
See the docs for more details.
Support for Math Functions in .eval()¶
eval() now supports calling math functions (GH4893)
df = pd.DataFrame({'a': np.random.randn(10)})
df.eval("b = sin(a)")
The support math functions are sin, cos, exp, log, expm1, log1p, sqrt, sinh, cosh, tanh, arcsin, arccos, arctan, arccosh, arcsinh, arctanh, abs and arctan2.
These functions map to the intrinsics for the NumExpr engine. For the Python engine, they are mapped to NumPy calls.
Changes to Excel with MultiIndex¶
In version 0.16.2 a DataFrame with MultiIndex columns could not be written to Excel via to_excel. That functionality has been added (GH10564), along with updating read_excel so that the data can be read back with, no loss of information, by specifying which columns/rows make up the MultiIndex in the header and index_col parameters (GH4679)
See the documentation for more details.
In [31]: df = pd.DataFrame([[1,2,3,4], [5,6,7,8]],
....: columns = pd.MultiIndex.from_product([['foo','bar'],['a','b']],
....: names = ['col1', 'col2']),
....: index = pd.MultiIndex.from_product([['j'], ['l', 'k']],
....: names = ['i1', 'i2']))
....:
In [32]: df
Out[32]:
col1 foo bar
col2 a b a b
i1 i2
j l 1 2 3 4
k 5 6 7 8
In [33]: df.to_excel('test.xlsx')
In [34]: df = pd.read_excel('test.xlsx', header=[0,1], index_col=[0,1])
In [35]: df
Out[35]:
col1 foo bar
col2 a b a b
i1 i2
j l 1 2 3 4
k 5 6 7 8
Previously, it was necessary to specify the has_index_names argument in read_excel, if the serialized data had index names. For version 0.17.0 the ouptput format of to_excel has been changed to make this keyword unnecessary - the change is shown below.
Old
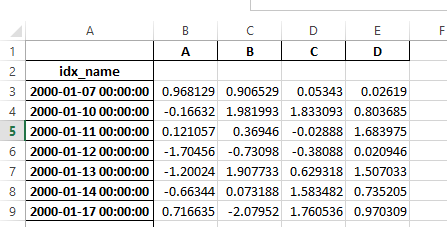
New
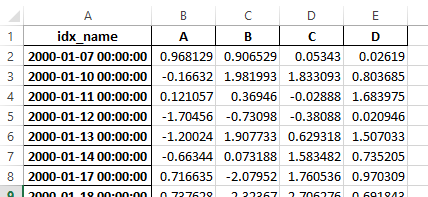
Warning
Excel files saved in version 0.16.2 or prior that had index names will still able to be read in, but the has_index_names argument must specified to True.
Google BigQuery Enhancements¶
- Added ability to automatically create a table/dataset using the pandas.io.gbq.to_gbq() function if the destination table/dataset does not exist. (GH8325, GH11121).
- Added ability to replace an existing table and schema when calling the pandas.io.gbq.to_gbq() function via the if_exists argument. See the docs for more details (GH8325).
- InvalidColumnOrder and InvalidPageToken in the gbq module will raise ValueError instead of IOError.
- The generate_bq_schema() function is now deprecated and will be removed in a future version (GH11121)
- The gbq module will now support Python 3 (GH11094).
Display Alignment with Unicode East Asian Width¶
Warning
Enabling this option will affect the performance for printing of DataFrame and Series (about 2 times slower). Use only when it is actually required.
Some East Asian countries use Unicode characters its width is corresponding to 2 alphabets. If a DataFrame or Series contains these characters, the default output cannot be aligned properly. The following options are added to enable precise handling for these characters.
- display.unicode.east_asian_width: Whether to use the Unicode East Asian Width to calculate the display text width. (GH2612)
- display.unicode.ambiguous_as_wide: Whether to handle Unicode characters belong to Ambiguous as Wide. (GH11102)
In [36]: df = pd.DataFrame({u'国籍': ['UK', u'日本'], u'名前': ['Alice', u'しのぶ']})
In [37]: df;

In [38]: pd.set_option('display.unicode.east_asian_width', True)
In [39]: df;

For further details, see here
Other enhancements¶
Support for openpyxl >= 2.2. The API for style support is now stable (GH10125)
merge now accepts the argument indicator which adds a Categorical-type column (by default called _merge) to the output object that takes on the values (GH8790)
Observation Origin _merge value Merge key only in 'left' frame left_only Merge key only in 'right' frame right_only Merge key in both frames both In [40]: df1 = pd.DataFrame({'col1':[0,1], 'col_left':['a','b']}) In [41]: df2 = pd.DataFrame({'col1':[1,2,2],'col_right':[2,2,2]}) In [42]: pd.merge(df1, df2, on='col1', how='outer', indicator=True) Out[42]: col1 col_left col_right _merge 0 0 a NaN left_only 1 1 b 2 both 2 2 NaN 2 right_only 3 2 NaN 2 right_only
For more, see the updated docs
pd.to_numeric is a new function to coerce strings to numbers (possibly with coercion) (GH11133)
pd.merge will now allow duplicate column names if they are not merged upon (GH10639).
pd.pivot will now allow passing index as None (GH3962).
pd.concat will now use existing Series names if provided (GH10698).
In [43]: foo = pd.Series([1,2], name='foo') In [44]: bar = pd.Series([1,2]) In [45]: baz = pd.Series([4,5])
Previous Behavior:
In [1] pd.concat([foo, bar, baz], 1) Out[1]: 0 1 2 0 1 1 4 1 2 2 5
New Behavior:
In [46]: pd.concat([foo, bar, baz], 1) Out[46]: foo 0 1 0 1 1 4 1 2 2 5
DataFrame has gained the nlargest and nsmallest methods (GH10393)
Add a limit_direction keyword argument that works with limit to enable interpolate to fill NaN values forward, backward, or both (GH9218, GH10420, GH11115)
In [47]: ser = pd.Series([np.nan, np.nan, 5, np.nan, np.nan, np.nan, 13]) In [48]: ser.interpolate(limit=1, limit_direction='both') Out[48]: 0 NaN 1 5 2 5 3 7 4 NaN 5 11 6 13 dtype: float64
Added a DataFrame.round method to round the values to a variable number of decimal places (GH10568).
In [49]: df = pd.DataFrame(np.random.random([3, 3]), columns=['A', 'B', 'C'], ....: index=['first', 'second', 'third']) ....: In [50]: df Out[50]: A B C first 0.342764 0.304121 0.417022 second 0.681301 0.875457 0.510422 third 0.669314 0.585937 0.624904 In [51]: df.round(2) Out[51]: A B C first 0.34 0.30 0.42 second 0.68 0.88 0.51 third 0.67 0.59 0.62 In [52]: df.round({'A': 0, 'C': 2}) Out[52]: A B C first 0 0.304121 0.42 second 1 0.875457 0.51 third 1 0.585937 0.62
drop_duplicates and duplicated now accept a keep keyword to target first, last, and all duplicates. The take_last keyword is deprecated, see here (GH6511, GH8505)
In [53]: s = pd.Series(['A', 'B', 'C', 'A', 'B', 'D']) In [54]: s.drop_duplicates() Out[54]: 0 A 1 B 2 C 5 D dtype: object In [55]: s.drop_duplicates(keep='last') Out[55]: 2 C 3 A 4 B 5 D dtype: object In [56]: s.drop_duplicates(keep=False) Out[56]: 2 C 5 D dtype: object
Reindex now has a tolerance argument that allows for finer control of Limits on filling while reindexing (GH10411):
In [57]: df = pd.DataFrame({'x': range(5), ....: 't': pd.date_range('2000-01-01', periods=5)}) ....: In [58]: df.reindex([0.1, 1.9, 3.5], ....: method='nearest', ....: tolerance=0.2) ....: Out[58]: t x 0.1 2000-01-01 0 1.9 2000-01-03 2 3.5 NaT NaN
When used on a DatetimeIndex, TimedeltaIndex or PeriodIndex, tolerance will coerced into a Timedelta if possible. This allows you to specify tolerance with a string:
In [59]: df = df.set_index('t') In [60]: df.reindex(pd.to_datetime(['1999-12-31']), ....: method='nearest', ....: tolerance='1 day') ....: Out[60]: x 1999-12-31 0
tolerance is also exposed by the lower level Index.get_indexer and Index.get_loc methods.
Added functionality to use the base argument when resampling a TimeDeltaIndex (GH10530)
DatetimeIndex can be instantiated using strings contains NaT (GH7599)
to_datetime can now accept the yearfirst keyword (GH7599)
pandas.tseries.offsets larger than the Day offset can now be used with a Series for addition/subtraction (GH10699). See the docs for more details.
pd.Timedelta.total_seconds() now returns Timedelta duration to ns precision (previously microsecond precision) (GH10939)
PeriodIndex now supports arithmetic with np.ndarray (GH10638)
Support pickling of Period objects (GH10439)
.as_blocks will now take a copy optional argument to return a copy of the data, default is to copy (no change in behavior from prior versions), (GH9607)
regex argument to DataFrame.filter now handles numeric column names instead of raising ValueError (GH10384).
Enable reading gzip compressed files via URL, either by explicitly setting the compression parameter or by inferring from the presence of the HTTP Content-Encoding header in the response (GH8685)
Enable writing Excel files in memory using StringIO/BytesIO (GH7074)
Enable serialization of lists and dicts to strings in ExcelWriter (GH8188)
SQL io functions now accept a SQLAlchemy connectable. (GH7877)
pd.read_sql and to_sql can accept database URI as con parameter (GH10214)
read_sql_table will now allow reading from views (GH10750).
Enable writing complex values to HDFStores when using the table format (GH10447)
Enable pd.read_hdf to be used without specifying a key when the HDF file contains a single dataset (GH10443)
pd.read_stata will now read Stata 118 type files. (GH9882)
msgpack submodule has been updated to 0.4.6 with backward compatibility (GH10581)
DataFrame.to_dict now accepts orient='index' keyword argument (GH10844).
DataFrame.apply will return a Series of dicts if the passed function returns a dict and reduce=True (GH8735).
Allow passing kwargs to the interpolation methods (GH10378).
Improved error message when concatenating an empty iterable of Dataframe objects (GH9157)
pd.read_csv can now read bz2-compressed files incrementally, and the C parser can read bz2-compressed files from AWS S3 (GH11070, GH11072).
In pd.read_csv, recognize s3n:// and s3a:// URLs as designating S3 file storage (GH11070, GH11071).
Read CSV files from AWS S3 incrementally, instead of first downloading the entire file. (Full file download still required for compressed files in Python 2.) (GH11070, GH11073)
pd.read_csv is now able to infer compression type for files read from AWS S3 storage (GH11070, GH11074).
Backwards incompatible API changes¶
Changes to sorting API¶
The sorting API has had some longtime inconsistencies. (GH9816, GH8239).
Here is a summary of the API PRIOR to 0.17.0:
- Series.sort is INPLACE while DataFrame.sort returns a new object.
- Series.order returns a new object
- It was possible to use Series/DataFrame.sort_index to sort by values by passing the by keyword.
- Series/DataFrame.sortlevel worked only on a MultiIndex for sorting by index.
To address these issues, we have revamped the API:
- We have introduced a new method, DataFrame.sort_values(), which is the merger of DataFrame.sort(), Series.sort(), and Series.order(), to handle sorting of values.
- The existing methods Series.sort(), Series.order(), and DataFrame.sort() have been deprecated and will be removed in a future version.
- The by argument of DataFrame.sort_index() has been deprecated and will be removed in a future version.
- The existing method .sort_index() will gain the level keyword to enable level sorting.
We now have two distinct and non-overlapping methods of sorting. A * marks items that will show a FutureWarning.
To sort by the values:
Previous | Replacement |
---|---|
* Series.order() | Series.sort_values() |
* Series.sort() | Series.sort_values(inplace=True) |
* DataFrame.sort(columns=...) | DataFrame.sort_values(by=...) |
To sort by the index:
Previous | Replacement |
---|---|
Series.sort_index() | Series.sort_index() |
Series.sortlevel(level=...) | Series.sort_index(level=...) |
DataFrame.sort_index() | DataFrame.sort_index() |
DataFrame.sortlevel(level=...) | DataFrame.sort_index(level=...) |
* DataFrame.sort() | DataFrame.sort_index() |
We have also deprecated and changed similar methods in two Series-like classes, Index and Categorical.
Previous | Replacement |
---|---|
* Index.order() | Index.sort_values() |
* Categorical.order() | Categorical.sort_values() |
Changes to to_datetime and to_timedelta¶
Error handling¶
The default for pd.to_datetime error handling has changed to errors='raise'. In prior versions it was errors='ignore'. Furthermore, the coerce argument has been deprecated in favor of errors='coerce'. This means that invalid parsing will raise rather that return the original input as in previous versions. (GH10636)
Previous Behavior:
In [2]: pd.to_datetime(['2009-07-31', 'asd'])
Out[2]: array(['2009-07-31', 'asd'], dtype=object)
New Behavior:
In [3]: pd.to_datetime(['2009-07-31', 'asd'])
ValueError: Unknown string format
Of course you can coerce this as well.
In [61]: to_datetime(['2009-07-31', 'asd'], errors='coerce')
Out[61]: DatetimeIndex(['2009-07-31', 'NaT'], dtype='datetime64[ns]', freq=None)
To keep the previous behavior, you can use errors='ignore':
In [62]: to_datetime(['2009-07-31', 'asd'], errors='ignore')
Out[62]: array(['2009-07-31', 'asd'], dtype=object)
Furthermore, pd.to_timedelta has gained a similar API, of errors='raise'|'ignore'|'coerce', and the coerce keyword has been deprecated in favor of errors='coerce'.
Consistent Parsing¶
The string parsing of to_datetime, Timestamp and DatetimeIndex has been made consistent. (GH7599)
Prior to v0.17.0, Timestamp and to_datetime may parse year-only datetime-string incorrectly using today’s date, otherwise DatetimeIndex uses the beginning of the year. Timestamp and to_datetime may raise ValueError in some types of datetime-string which DatetimeIndex can parse, such as a quarterly string.
Previous Behavior:
In [1]: Timestamp('2012Q2')
Traceback
...
ValueError: Unable to parse 2012Q2
# Results in today's date.
In [2]: Timestamp('2014')
Out [2]: 2014-08-12 00:00:00
v0.17.0 can parse them as below. It works on DatetimeIndex also.
New Behavior:
In [63]: Timestamp('2012Q2')
Out[63]: Timestamp('2012-04-01 00:00:00')
In [64]: Timestamp('2014')
Out[64]: Timestamp('2014-01-01 00:00:00')
In [65]: DatetimeIndex(['2012Q2', '2014'])
Out[65]: DatetimeIndex(['2012-04-01', '2014-01-01'], dtype='datetime64[ns]', freq=None)
Note
If you want to perform calculations based on today’s date, use Timestamp.now() and pandas.tseries.offsets.
In [66]: import pandas.tseries.offsets as offsets
In [67]: Timestamp.now()
Out[67]: Timestamp('2015-11-21 02:38:37.772350')
In [68]: Timestamp.now() + offsets.DateOffset(years=1)
Out[68]: Timestamp('2016-11-21 02:38:37.906963')
Changes to Index Comparisons¶
Operator equal on Index should behavior similarly to Series (GH9947, GH10637)
Starting in v0.17.0, comparing Index objects of different lengths will raise a ValueError. This is to be consistent with the behavior of Series.
Previous Behavior:
In [2]: pd.Index([1, 2, 3]) == pd.Index([1, 4, 5])
Out[2]: array([ True, False, False], dtype=bool)
In [3]: pd.Index([1, 2, 3]) == pd.Index([2])
Out[3]: array([False, True, False], dtype=bool)
In [4]: pd.Index([1, 2, 3]) == pd.Index([1, 2])
Out[4]: False
New Behavior:
In [8]: pd.Index([1, 2, 3]) == pd.Index([1, 4, 5])
Out[8]: array([ True, False, False], dtype=bool)
In [9]: pd.Index([1, 2, 3]) == pd.Index([2])
ValueError: Lengths must match to compare
In [10]: pd.Index([1, 2, 3]) == pd.Index([1, 2])
ValueError: Lengths must match to compare
Note that this is different from the numpy behavior where a comparison can be broadcast:
In [69]: np.array([1, 2, 3]) == np.array([1])
Out[69]: array([ True, False, False], dtype=bool)
or it can return False if broadcasting can not be done:
In [70]: np.array([1, 2, 3]) == np.array([1, 2])
Out[70]: False
Changes to Boolean Comparisons vs. None¶
Boolean comparisons of a Series vs None will now be equivalent to comparing with np.nan, rather than raise TypeError. (GH1079).
In [71]: s = Series(range(3))
In [72]: s.iloc[1] = None
In [73]: s
Out[73]:
0 0
1 NaN
2 2
dtype: float64
Previous Behavior:
In [5]: s==None
TypeError: Could not compare <type 'NoneType'> type with Series
New Behavior:
In [74]: s==None
Out[74]:
0 False
1 False
2 False
dtype: bool
Usually you simply want to know which values are null.
In [75]: s.isnull()
Out[75]:
0 False
1 True
2 False
dtype: bool
Warning
You generally will want to use isnull/notnull for these types of comparisons, as isnull/notnull tells you which elements are null. One has to be mindful that nan's don’t compare equal, but None's do. Note that Pandas/numpy uses the fact that np.nan != np.nan, and treats None like np.nan.
In [76]: None == None
Out[76]: True
In [77]: np.nan == np.nan
Out[77]: False
HDFStore dropna behavior¶
The default behavior for HDFStore write functions with format='table' is now to keep rows that are all missing. Previously, the behavior was to drop rows that were all missing save the index. The previous behavior can be replicated using the dropna=True option. (GH9382)
Previous Behavior:
In [78]: df_with_missing = pd.DataFrame({'col1':[0, np.nan, 2],
....: 'col2':[1, np.nan, np.nan]})
....:
In [79]: df_with_missing
Out[79]:
col1 col2
0 0 1
1 NaN NaN
2 2 NaN
In [28]:
df_with_missing.to_hdf('file.h5',
'df_with_missing',
format='table',
mode='w')
pd.read_hdf('file.h5', 'df_with_missing')
Out [28]:
col1 col2
0 0 1
2 2 NaN
New Behavior:
In [80]: df_with_missing.to_hdf('file.h5',
....: 'df_with_missing',
....: format='table',
....: mode='w')
....:
In [81]: pd.read_hdf('file.h5', 'df_with_missing')
Out[81]:
col1 col2
0 0 1
1 NaN NaN
2 2 NaN
See the docs for more details.
Changes to display.precision option¶
The display.precision option has been clarified to refer to decimal places (GH10451).
Earlier versions of pandas would format floating point numbers to have one less decimal place than the value in display.precision.
In [1]: pd.set_option('display.precision', 2)
In [2]: pd.DataFrame({'x': [123.456789]})
Out[2]:
x
0 123.5
If interpreting precision as “significant figures” this did work for scientific notation but that same interpretation did not work for values with standard formatting. It was also out of step with how numpy handles formatting.
Going forward the value of display.precision will directly control the number of places after the decimal, for regular formatting as well as scientific notation, similar to how numpy’s precision print option works.
In [82]: pd.set_option('display.precision', 2)
In [83]: pd.DataFrame({'x': [123.456789]})
Out[83]:
x
0 123.46
To preserve output behavior with prior versions the default value of display.precision has been reduced to 6 from 7.
Changes to Categorical.unique¶
Categorical.unique now returns new Categoricals with categories and codes that are unique, rather than returning np.array (GH10508)
- unordered category: values and categories are sorted by appearance order.
- ordered category: values are sorted by appearance order, categories keep existing order.
In [84]: cat = pd.Categorical(['C', 'A', 'B', 'C'],
....: categories=['A', 'B', 'C'],
....: ordered=True)
....:
In [85]: cat
Out[85]:
[C, A, B, C]
Categories (3, object): [A < B < C]
In [86]: cat.unique()
Out[86]:
[C, A, B]
Categories (3, object): [A < B < C]
In [87]: cat = pd.Categorical(['C', 'A', 'B', 'C'],
....: categories=['A', 'B', 'C'])
....:
In [88]: cat
Out[88]:
[C, A, B, C]
Categories (3, object): [A, B, C]
In [89]: cat.unique()
Out[89]:
[C, A, B]
Categories (3, object): [C, A, B]
Changes to bool passed as header in Parsers¶
In earlier versions of pandas, if a bool was passed the header argument of read_csv, read_excel, or read_html it was implicitly converted to an integer, resulting in header=0 for False and header=1 for True (GH6113)
A bool input to header will now raise a TypeError
In [29]: df = pd.read_csv('data.csv', header=False)
TypeError: Passing a bool to header is invalid. Use header=None for no header or
header=int or list-like of ints to specify the row(s) making up the column names
Other API Changes¶
Line and kde plot with subplots=True now uses default colors, not all black. Specify color='k' to draw all lines in black (GH9894)
Calling the .value_counts() method on a Series with a categorical dtype now returns a Series with a CategoricalIndex (GH10704)
The metadata properties of subclasses of pandas objects will now be serialized (GH10553).
groupby using Categorical follows the same rule as Categorical.unique described above (GH10508)
When constructing DataFrame with an array of complex64 dtype previously meant the corresponding column was automatically promoted to the complex128 dtype. Pandas will now preserve the itemsize of the input for complex data (GH10952)
some numeric reduction operators would return ValueError, rather than TypeError on object types that includes strings and numbers (GH11131)
Passing currently unsupported chunksize argument to read_excel or ExcelFile.parse will now raise NotImplementedError (GH8011)
Allow an ExcelFile object to be passed into read_excel (GH11198)
DatetimeIndex.union does not infer freq if self and the input have None as freq (GH11086)
NaT‘s methods now either raise ValueError, or return np.nan or NaT (GH9513)
Behavior Methods return np.nan weekday, isoweekday return NaT date, now, replace, to_datetime, today return np.datetime64('NaT') to_datetime64 (unchanged) raise ValueError All other public methods (names not beginning with underscores)
Deprecations¶
For Series the following indexing functions are deprecated (GH10177).
Deprecated Function Replacement .irow(i) .iloc[i] or .iat[i] .iget(i) .iloc[i] or .iat[i] .iget_value(i) .iloc[i] or .iat[i] For DataFrame the following indexing functions are deprecated (GH10177).
Deprecated Function Replacement .irow(i) .iloc[i] .iget_value(i, j) .iloc[i, j] or .iat[i, j] .icol(j) .iloc[:, j]
Note
These indexing function have been deprecated in the documentation since 0.11.0.
- Categorical.name was deprecated to make Categorical more numpy.ndarray like. Use Series(cat, name="whatever") instead (GH10482).
- Setting missing values (NaN) in a Categorical‘s categories will issue a warning (GH10748). You can still have missing values in the values.
- drop_duplicates and duplicated‘s take_last keyword was deprecated in favor of keep. (GH6511, GH8505)
- Series.nsmallest and nlargest‘s take_last keyword was deprecated in favor of keep. (GH10792)
- DataFrame.combineAdd and DataFrame.combineMult are deprecated. They can easily be replaced by using the add and mul methods: DataFrame.add(other, fill_value=0) and DataFrame.mul(other, fill_value=1.) (GH10735).
- TimeSeries deprecated in favor of Series (note that this has been an alias since 0.13.0), (GH10890)
- SparsePanel deprecated and will be removed in a future version (GH11157).
- Series.is_time_series deprecated in favor of Series.index.is_all_dates (GH11135)
- Legacy offsets (like 'A@JAN') listed in here are deprecated (note that this has been alias since 0.8.0), (GH10878)
- WidePanel deprecated in favor of Panel, LongPanel in favor of DataFrame (note these have been aliases since < 0.11.0), (GH10892)
- DataFrame.convert_objects has been deprecated in favor of type-specific functions pd.to_datetime, pd.to_timestamp and pd.to_numeric (new in 0.17.0) (GH11133).
Removal of prior version deprecations/changes¶
Removal of na_last parameters from Series.order() and Series.sort(), in favor of na_position. (GH5231)
Remove of percentile_width from .describe(), in favor of percentiles. (GH7088)
Removal of colSpace parameter from DataFrame.to_string(), in favor of col_space, circa 0.8.0 version.
Removal of automatic time-series broadcasting (GH2304)
In [90]: np.random.seed(1234) In [91]: df = DataFrame(np.random.randn(5,2),columns=list('AB'),index=date_range('20130101',periods=5)) In [92]: df Out[92]: A B 2013-01-01 0.471435 -1.190976 2013-01-02 1.432707 -0.312652 2013-01-03 -0.720589 0.887163 2013-01-04 0.859588 -0.636524 2013-01-05 0.015696 -2.242685
Previously
In [3]: df + df.A FutureWarning: TimeSeries broadcasting along DataFrame index by default is deprecated. Please use DataFrame.<op> to explicitly broadcast arithmetic operations along the index Out[3]: A B 2013-01-01 0.942870 -0.719541 2013-01-02 2.865414 1.120055 2013-01-03 -1.441177 0.166574 2013-01-04 1.719177 0.223065 2013-01-05 0.031393 -2.226989
Current
In [93]: df.add(df.A,axis='index') Out[93]: A B 2013-01-01 0.942870 -0.719541 2013-01-02 2.865414 1.120055 2013-01-03 -1.441177 0.166574 2013-01-04 1.719177 0.223065 2013-01-05 0.031393 -2.226989
Remove table keyword in HDFStore.put/append, in favor of using format= (GH4645)
Remove kind in read_excel/ExcelFile as its unused (GH4712)
Remove infer_type keyword from pd.read_html as its unused (GH4770, GH7032)
Remove offset and timeRule keywords from Series.tshift/shift, in favor of freq (GH4853, GH4864)
Remove pd.load/pd.save aliases in favor of pd.to_pickle/pd.read_pickle (GH3787)
Performance Improvements¶
- Development support for benchmarking with the Air Speed Velocity library (GH8361)
- Added vbench benchmarks for alternative ExcelWriter engines and reading Excel files (GH7171)
- Performance improvements in Categorical.value_counts (GH10804)
- Performance improvements in SeriesGroupBy.nunique and SeriesGroupBy.value_counts and SeriesGroupby.transform (GH10820, GH11077)
- Performance improvements in DataFrame.drop_duplicates with integer dtypes (GH10917)
- Performance improvements in DataFrame.duplicated with wide frames. (GH10161, GH11180)
- 4x improvement in timedelta string parsing (GH6755, GH10426)
- 8x improvement in timedelta64 and datetime64 ops (GH6755)
- Significantly improved performance of indexing MultiIndex with slicers (GH10287)
- 8x improvement in iloc using list-like input (GH10791)
- Improved performance of Series.isin for datetimelike/integer Series (GH10287)
- 20x improvement in concat of Categoricals when categories are identical (GH10587)
- Improved performance of to_datetime when specified format string is ISO8601 (GH10178)
- 2x improvement of Series.value_counts for float dtype (GH10821)
- Enable infer_datetime_format in to_datetime when date components do not have 0 padding (GH11142)
- Regression from 0.16.1 in constructing DataFrame from nested dictionary (GH11084)
- Performance improvements in addition/subtraction operations for DateOffset with Series or DatetimeIndex (GH10744, GH11205)
Bug Fixes¶
- Bug in incorrection computation of .mean() on timedelta64[ns] because of overflow (GH9442)
- Bug in .isin on older numpies (:issue: 11232)
- Bug in DataFrame.to_html(index=False) renders unnecessary name row (GH10344)
- Bug in DataFrame.to_latex() the column_format argument could not be passed (GH9402)
- Bug in DatetimeIndex when localizing with NaT (GH10477)
- Bug in Series.dt ops in preserving meta-data (GH10477)
- Bug in preserving NaT when passed in an otherwise invalid to_datetime construction (GH10477)
- Bug in DataFrame.apply when function returns categorical series. (GH9573)
- Bug in to_datetime with invalid dates and formats supplied (GH10154)
- Bug in Index.drop_duplicates dropping name(s) (GH10115)
- Bug in Series.quantile dropping name (GH10881)
- Bug in pd.Series when setting a value on an empty Series whose index has a frequency. (GH10193)
- Bug in pd.Series.interpolate with invalid order keyword values. (GH10633)
- Bug in DataFrame.plot raises ValueError when color name is specified by multiple characters (GH10387)
- Bug in Index construction with a mixed list of tuples (GH10697)
- Bug in DataFrame.reset_index when index contains NaT. (GH10388)
- Bug in ExcelReader when worksheet is empty (GH6403)
- Bug in BinGrouper.group_info where returned values are not compatible with base class (GH10914)
- Bug in clearing the cache on DataFrame.pop and a subsequent inplace op (GH10912)
- Bug in indexing with a mixed-integer Index causing an ImportError (GH10610)
- Bug in Series.count when index has nulls (GH10946)
- Bug in pickling of a non-regular freq DatetimeIndex (GH11002)
- Bug causing DataFrame.where to not respect the axis parameter when the frame has a symmetric shape. (GH9736)
- Bug in Table.select_column where name is not preserved (GH10392)
- Bug in offsets.generate_range where start and end have finer precision than offset (GH9907)
- Bug in pd.rolling_* where Series.name would be lost in the output (GH10565)
- Bug in stack when index or columns are not unique. (GH10417)
- Bug in setting a Panel when an axis has a multi-index (GH10360)
- Bug in USFederalHolidayCalendar where USMemorialDay and USMartinLutherKingJr were incorrect (GH10278 and GH9760 )
- Bug in .sample() where returned object, if set, gives unnecessary SettingWithCopyWarning (GH10738)
- Bug in .sample() where weights passed as Series were not aligned along axis before being treated positionally, potentially causing problems if weight indices were not aligned with sampled object. (GH10738)
- Regression fixed in (GH9311, GH6620, GH9345), where groupby with a datetime-like converting to float with certain aggregators (GH10979)
- Bug in DataFrame.interpolate with axis=1 and inplace=True (GH10395)
- Bug in io.sql.get_schema when specifying multiple columns as primary key (GH10385).
- Bug in groupby(sort=False) with datetime-like Categorical raises ValueError (GH10505)
- Bug in groupby(axis=1) with filter() throws IndexError (GH11041)
- Bug in test_categorical on big-endian builds (GH10425)
- Bug in Series.shift and DataFrame.shift not supporting categorical data (GH9416)
- Bug in Series.map using categorical Series raises AttributeError (GH10324)
- Bug in MultiIndex.get_level_values including Categorical raises AttributeError (GH10460)
- Bug in pd.get_dummies with sparse=True not returning SparseDataFrame (GH10531)
- Bug in Index subtypes (such as PeriodIndex) not returning their own type for .drop and .insert methods (GH10620)
- Bug in algos.outer_join_indexer when right array is empty (GH10618)
- Bug in filter (regression from 0.16.0) and transform when grouping on multiple keys, one of which is datetime-like (GH10114)
- Bug in to_datetime and to_timedelta causing Index name to be lost (GH10875)
- Bug in len(DataFrame.groupby) causing IndexError when there’s a column containing only NaNs (:issue: 11016)
- Bug that caused segfault when resampling an empty Series (GH10228)
- Bug in DatetimeIndex and PeriodIndex.value_counts resets name from its result, but retains in result’s Index. (GH10150)
- Bug in pd.eval using numexpr engine coerces 1 element numpy array to scalar (GH10546)
- Bug in pd.concat with axis=0 when column is of dtype category (GH10177)
- Bug in read_msgpack where input type is not always checked (GH10369, GH10630)
- Bug in pd.read_csv with kwargs index_col=False, index_col=['a', 'b'] or dtype (GH10413, GH10467, GH10577)
- Bug in Series.from_csv with header kwarg not setting the Series.name or the Series.index.name (GH10483)
- Bug in groupby.var which caused variance to be inaccurate for small float values (GH10448)
- Bug in Series.plot(kind='hist') Y Label not informative (GH10485)
- Bug in read_csv when using a converter which generates a uint8 type (GH9266)
- Bug causes memory leak in time-series line and area plot (GH9003)
- Bug when setting a Panel sliced along the major or minor axes when the right-hand side is a DataFrame (GH11014)
- Bug that returns None and does not raise NotImplementedError when operator functions (e.g. .add) of Panel are not implemented (GH7692)
- Bug in line and kde plot cannot accept multiple colors when subplots=True (GH9894)
- Bug in DataFrame.plot raises ValueError when color name is specified by multiple characters (GH10387)
- Bug in left and right align of Series with MultiIndex may be inverted (GH10665)
- Bug in left and right join of with MultiIndex may be inverted (GH10741)
- Bug in read_stata when reading a file with a different order set in columns (GH10757)
- Bug in Categorical may not representing properly when category contains tz or Period (GH10713)
- Bug in Categorical.__iter__ may not returning correct datetime and Period (GH10713)
- Bug in indexing with a PeriodIndex on an object with a PeriodIndex (GH4125)
- Bug in read_csv with engine='c': EOF preceded by a comment, blank line, etc. was not handled correctly (GH10728, GH10548)
- Reading “famafrench” data via DataReader results in HTTP 404 error because of the website url is changed (GH10591).
- Bug in read_msgpack where DataFrame to decode has duplicate column names (GH9618)
- Bug in io.common.get_filepath_or_buffer which caused reading of valid S3 files to fail if the bucket also contained keys for which the user does not have read permission (GH10604)
- Bug in vectorised setting of timestamp columns with python datetime.date and numpy datetime64 (GH10408, GH10412)
- Bug in Index.take may add unnecessary freq attribute (GH10791)
- Bug in merge with empty DataFrame may raise IndexError (GH10824)
- Bug in to_latex where unexpected keyword argument for some documented arguments (GH10888)
- Bug in indexing of large DataFrame where IndexError is uncaught (GH10645 and GH10692)
- Bug in read_csv when using the nrows or chunksize parameters if file contains only a header line (GH9535)
- Bug in serialization of category types in HDF5 in presence of alternate encodings. (GH10366)
- Bug in pd.DataFrame when constructing an empty DataFrame with a string dtype (GH9428)
- Bug in pd.DataFrame.diff when DataFrame is not consolidated (GH10907)
- Bug in pd.unique for arrays with the datetime64 or timedelta64 dtype that meant an array with object dtype was returned instead the original dtype (GH9431)
- Bug in Timedelta raising error when slicing from 0s (GH10583)
- Bug in DatetimeIndex.take and TimedeltaIndex.take may not raise IndexError against invalid index (GH10295)
- Bug in Series([np.nan]).astype('M8[ms]'), which now returns Series([pd.NaT]) (GH10747)
- Bug in PeriodIndex.order reset freq (GH10295)
- Bug in date_range when freq divides end as nanos (GH10885)
- Bug in iloc allowing memory outside bounds of a Series to be accessed with negative integers (GH10779)
- Bug in read_msgpack where encoding is not respected (GH10581)
- Bug preventing access to the first index when using iloc with a list containing the appropriate negative integer (GH10547, GH10779)
- Bug in TimedeltaIndex formatter causing error while trying to save DataFrame with TimedeltaIndex using to_csv (GH10833)
- Bug in DataFrame.where when handling Series slicing (GH10218, GH9558)
- Bug where pd.read_gbq throws ValueError when Bigquery returns zero rows (GH10273)
- Bug in to_json which was causing segmentation fault when serializing 0-rank ndarray (GH9576)
- Bug in plotting functions may raise IndexError when plotted on GridSpec (GH10819)
- Bug in plot result may show unnecessary minor ticklabels (GH10657)
- Bug in groupby incorrect computation for aggregation on DataFrame with NaT (E.g first, last, min). (GH10590, GH11010)
- Bug when constructing DataFrame where passing a dictionary with only scalar values and specifying columns did not raise an error (GH10856)
- Bug in .var() causing roundoff errors for highly similar values (GH10242)
- Bug in DataFrame.plot(subplots=True) with duplicated columns outputs incorrect result (GH10962)
- Bug in Index arithmetic may result in incorrect class (GH10638)
- Bug in date_range results in empty if freq is negative annualy, quarterly and monthly (GH11018)
- Bug in DatetimeIndex cannot infer negative freq (GH11018)
- Remove use of some deprecated numpy comparison operations, mainly in tests. (GH10569)
- Bug in Index dtype may not applied properly (GH11017)
- Bug in io.gbq when testing for minimum google api client version (GH10652)
- Bug in DataFrame construction from nested dict with timedelta keys (GH11129)
- Bug in .fillna against may raise TypeError when data contains datetime dtype (GH7095, GH11153)
- Bug in .groupby when number of keys to group by is same as length of index (GH11185)
- Bug in convert_objects where converted values might not be returned if all null and coerce (GH9589)
- Bug in convert_objects where copy keyword was not respected (GH9589)
v0.16.2 (June 12, 2015)¶
This is a minor bug-fix release from 0.16.1 and includes a a large number of bug fixes along some new features (pipe() method), enhancements, and performance improvements.
We recommend that all users upgrade to this version.
Highlights include:
What’s new in v0.16.2
New features¶
Pipe¶
We’ve introduced a new method DataFrame.pipe(). As suggested by the name, pipe should be used to pipe data through a chain of function calls. The goal is to avoid confusing nested function calls like
# df is a DataFrame
# f, g, and h are functions that take and return DataFrames
f(g(h(df), arg1=1), arg2=2, arg3=3)
The logic flows from inside out, and function names are separated from their keyword arguments. This can be rewritten as
(df.pipe(h)
.pipe(g, arg1=1)
.pipe(f, arg2=2, arg3=3)
)
Now both the code and the logic flow from top to bottom. Keyword arguments are next to their functions. Overall the code is much more readable.
In the example above, the functions f, g, and h each expected the DataFrame as the first positional argument. When the function you wish to apply takes its data anywhere other than the first argument, pass a tuple of (function, keyword) indicating where the DataFrame should flow. For example:
In [1]: import statsmodels.formula.api as sm
In [2]: bb = pd.read_csv('data/baseball.csv', index_col='id')
# sm.poisson takes (formula, data)
In [3]: (bb.query('h > 0')
...: .assign(ln_h = lambda df: np.log(df.h))
...: .pipe((sm.poisson, 'data'), 'hr ~ ln_h + year + g + C(lg)')
...: .fit()
...: .summary()
...: )
...:
Optimization terminated successfully.
Current function value: 2.116284
Iterations 24
Out[3]:
<class 'statsmodels.iolib.summary.Summary'>
"""
Poisson Regression Results
==============================================================================
Dep. Variable: hr No. Observations: 68
Model: Poisson Df Residuals: 63
Method: MLE Df Model: 4
Date: Sat, 21 Nov 2015 Pseudo R-squ.: 0.6878
Time: 02:38:40 Log-Likelihood: -143.91
converged: True LL-Null: -460.91
LLR p-value: 6.774e-136
===============================================================================
coef std err z P>|z| [95.0% Conf. Int.]
-------------------------------------------------------------------------------
Intercept -1267.3636 457.867 -2.768 0.006 -2164.767 -369.960
C(lg)[T.NL] -0.2057 0.101 -2.044 0.041 -0.403 -0.008
ln_h 0.9280 0.191 4.866 0.000 0.554 1.302
year 0.6301 0.228 2.762 0.006 0.183 1.077
g 0.0099 0.004 2.754 0.006 0.003 0.017
===============================================================================
"""
The pipe method is inspired by unix pipes, which stream text through processes. More recently dplyr and magrittr have introduced the popular (%>%) pipe operator for R.
See the documentation for more. (GH10129)
Other Enhancements¶
Added rsplit to Index/Series StringMethods (GH10303)
Removed the hard-coded size limits on the DataFrame HTML representation in the IPython notebook, and leave this to IPython itself (only for IPython v3.0 or greater). This eliminates the duplicate scroll bars that appeared in the notebook with large frames (GH10231).
Note that the notebook has a toggle output scrolling feature to limit the display of very large frames (by clicking left of the output). You can also configure the way DataFrames are displayed using the pandas options, see here here.
axis parameter of DataFrame.quantile now accepts also index and column. (GH9543)
API Changes¶
- Holiday now raises NotImplementedError if both offset and observance are used in the constructor instead of returning an incorrect result (GH10217).
Performance Improvements¶
Bug Fixes¶
- Bug in Series.hist raises an error when a one row Series was given (GH10214)
- Bug where HDFStore.select modifies the passed columns list (GH7212)
- Bug in Categorical repr with display.width of None in Python 3 (GH10087)
- Bug in to_json with certain orients and a CategoricalIndex would segfault (GH10317)
- Bug where some of the nan funcs do not have consistent return dtypes (GH10251)
- Bug in DataFrame.quantile on checking that a valid axis was passed (GH9543)
- Bug in groupby.apply aggregation for Categorical not preserving categories (GH10138)
- Bug in to_csv where date_format is ignored if the datetime is fractional (GH10209)
- Bug in DataFrame.to_json with mixed data types (GH10289)
- Bug in cache updating when consolidating (GH10264)
- Bug in mean() where integer dtypes can overflow (GH10172)
- Bug where Panel.from_dict does not set dtype when specified (GH10058)
- Bug in Index.union raises AttributeError when passing array-likes. (GH10149)
- Bug in Timestamp‘s’ microsecond, quarter, dayofyear, week and daysinmonth properties return np.int type, not built-in int. (GH10050)
- Bug in NaT raises AttributeError when accessing to daysinmonth, dayofweek properties. (GH10096)
- Bug in Index repr when using the max_seq_items=None setting (GH10182).
- Bug in getting timezone data with dateutil on various platforms ( GH9059, GH8639, GH9663, GH10121)
- Bug in displaying datetimes with mixed frequencies; display ‘ms’ datetimes to the proper precision. (GH10170)
- Bug in setitem where type promotion is applied to the entire block (GH10280)
- Bug in Series arithmetic methods may incorrectly hold names (GH10068)
- Bug in GroupBy.get_group when grouping on multiple keys, one of which is categorical. (GH10132)
- Bug in DatetimeIndex and TimedeltaIndex names are lost after timedelta arithmetics ( GH9926)
- Bug in DataFrame construction from nested dict with datetime64 (GH10160)
- Bug in Series construction from dict with datetime64 keys (GH9456)
- Bug in Series.plot(label="LABEL") not correctly setting the label (GH10119)
- Bug in plot not defaulting to matplotlib axes.grid setting (GH9792)
- Bug causing strings containing an exponent, but no decimal to be parsed as int instead of float in engine='python' for the read_csv parser (GH9565)
- Bug in Series.align resets name when fill_value is specified (GH10067)
- Bug in read_csv causing index name not to be set on an empty DataFrame (GH10184)
- Bug in SparseSeries.abs resets name (GH10241)
- Bug in TimedeltaIndex slicing may reset freq (GH10292)
- Bug in GroupBy.get_group raises ValueError when group key contains NaT (GH6992)
- Bug in SparseSeries constructor ignores input data name (GH10258)
- Bug in Categorical.remove_categories causing a ValueError when removing the NaN category if underlying dtype is floating-point (GH10156)
- Bug where infer_freq infers timerule (WOM-5XXX) unsupported by to_offset (GH9425)
- Bug in DataFrame.to_hdf() where table format would raise a seemingly unrelated error for invalid (non-string) column names. This is now explicitly forbidden. (GH9057)
- Bug to handle masking empty DataFrame (GH10126).
- Bug where MySQL interface could not handle numeric table/column names (GH10255)
- Bug in read_csv with a date_parser that returned a datetime64 array of other time resolution than [ns] (GH10245)
- Bug in Panel.apply when the result has ndim=0 (GH10332)
- Bug in read_hdf where auto_close could not be passed (GH9327).
- Bug in read_hdf where open stores could not be used (GH10330).
- Bug in adding empty DataFrame``s, now results in a ``DataFrame that .equals an empty DataFrame (GH10181).
- Bug in to_hdf and HDFStore which did not check that complib choices were valid (GH4582, GH8874).
v0.16.1 (May 11, 2015)¶
This is a minor bug-fix release from 0.16.0 and includes a a large number of bug fixes along several new features, enhancements, and performance improvements. We recommend that all users upgrade to this version.
Highlights include:
- Support for a CategoricalIndex, a category based index, see here
- New section on how-to-contribute to pandas, see here
- Revised “Merge, join, and concatenate” documentation, including graphical examples to make it easier to understand each operations, see here
- New method sample for drawing random samples from Series, DataFrames and Panels. See here
- The default Index printing has changed to a more uniform format, see here
- BusinessHour datetime-offset is now supported, see here
- Further enhancement to the .str accessor to make string operations easier, see here
What’s new in v0.16.1
Warning
In pandas 0.17.0, the sub-package pandas.io.data will be removed in favor of a separately installable package. See here for details (GH8961)
Enhancements¶
CategoricalIndex¶
We introduce a CategoricalIndex, a new type of index object that is useful for supporting indexing with duplicates. This is a container around a Categorical (introduced in v0.15.0) and allows efficient indexing and storage of an index with a large number of duplicated elements. Prior to 0.16.1, setting the index of a DataFrame/Series with a category dtype would convert this to regular object-based Index.
In [1]: df = DataFrame({'A' : np.arange(6),
...: 'B' : Series(list('aabbca')).astype('category',
...: categories=list('cab'))
...: })
...:
In [2]: df
Out[2]:
A B
0 0 a
1 1 a
2 2 b
3 3 b
4 4 c
5 5 a
In [3]: df.dtypes
Out[3]:
A int32
B category
dtype: object
In [4]: df.B.cat.categories
Out[4]: Index([u'c', u'a', u'b'], dtype='object')
setting the index, will create create a CategoricalIndex
In [5]: df2 = df.set_index('B')
In [6]: df2.index
Out[6]: CategoricalIndex([u'a', u'a', u'b', u'b', u'c', u'a'], categories=[u'c', u'a', u'b'], ordered=False, name=u'B', dtype='category')
indexing with __getitem__/.iloc/.loc/.ix works similarly to an Index with duplicates. The indexers MUST be in the category or the operation will raise.
In [7]: df2.loc['a']
Out[7]:
A
B
a 0
a 1
a 5
and preserves the CategoricalIndex
In [8]: df2.loc['a'].index
Out[8]: CategoricalIndex([u'a', u'a', u'a'], categories=[u'c', u'a', u'b'], ordered=False, name=u'B', dtype='category')
sorting will order by the order of the categories
In [9]: df2.sort_index()
Out[9]:
A
B
c 4
a 0
a 1
a 5
b 2
b 3
groupby operations on the index will preserve the index nature as well
In [10]: df2.groupby(level=0).sum()
Out[10]:
A
B
c 4
a 6
b 5
In [11]: df2.groupby(level=0).sum().index
Out[11]: CategoricalIndex([u'c', u'a', u'b'], categories=[u'c', u'a', u'b'], ordered=False, name=u'B', dtype='category')
reindexing operations, will return a resulting index based on the type of the passed indexer, meaning that passing a list will return a plain-old-Index; indexing with a Categorical will return a CategoricalIndex, indexed according to the categories of the PASSED Categorical dtype. This allows one to arbitrarly index these even with values NOT in the categories, similarly to how you can reindex ANY pandas index.
In [12]: df2.reindex(['a','e'])
Out[12]:
A
B
a 0
a 1
a 5
e NaN
In [13]: df2.reindex(['a','e']).index
Out[13]: Index([u'a', u'a', u'a', u'e'], dtype='object', name=u'B')
In [14]: df2.reindex(pd.Categorical(['a','e'],categories=list('abcde')))
Out[14]:
A
B
a 0
a 1
a 5
e NaN
In [15]: df2.reindex(pd.Categorical(['a','e'],categories=list('abcde'))).index
Out[15]: CategoricalIndex([u'a', u'a', u'a', u'e'], categories=[u'a', u'e'], ordered=False, name=u'B', dtype='category')
See the documentation for more. (GH7629, GH10038, GH10039)
Sample¶
Series, DataFrames, and Panels now have a new method: sample(). The method accepts a specific number of rows or columns to return, or a fraction of the total number or rows or columns. It also has options for sampling with or without replacement, for passing in a column for weights for non-uniform sampling, and for setting seed values to facilitate replication. (GH2419)
In [16]: example_series = Series([0,1,2,3,4,5])
# When no arguments are passed, returns 1
In [17]: example_series.sample()
Out[17]:
4 4
dtype: int64
# One may specify either a number of rows:
In [18]: example_series.sample(n=3)
Out[18]:
2 2
0 0
4 4
dtype: int64
# Or a fraction of the rows:
In [19]: example_series.sample(frac=0.5)
Out[19]:
3 3
4 4
0 0
dtype: int64
# weights are accepted.
In [20]: example_weights = [0, 0, 0.2, 0.2, 0.2, 0.4]
In [21]: example_series.sample(n=3, weights=example_weights)
Out[21]:
5 5
4 4
2 2
dtype: int64
# weights will also be normalized if they do not sum to one,
# and missing values will be treated as zeros.
In [22]: example_weights2 = [0.5, 0, 0, 0, None, np.nan]
In [23]: example_series.sample(n=1, weights=example_weights2)
Out[23]:
0 0
dtype: int64
When applied to a DataFrame, one may pass the name of a column to specify sampling weights when sampling from rows.
In [24]: df = DataFrame({'col1':[9,8,7,6], 'weight_column':[0.5, 0.4, 0.1, 0]})
In [25]: df.sample(n=3, weights='weight_column')
Out[25]:
col1 weight_column
0 9 0.5
2 7 0.1
1 8 0.4
String Methods Enhancements¶
Continuing from v0.16.0, the following enhancements make string operations easier and more consistent with standard python string operations.
Added StringMethods (.str accessor) to Index (GH9068)
The .str accessor is now available for both Series and Index.
In [26]: idx = Index([' jack', 'jill ', ' jesse ', 'frank']) In [27]: idx.str.strip() Out[27]: Index([u'jack', u'jill', u'jesse', u'frank'], dtype='object')
One special case for the .str accessor on Index is that if a string method returns bool, the .str accessor will return a np.array instead of a boolean Index (GH8875). This enables the following expression to work naturally:
In [28]: idx = Index(['a1', 'a2', 'b1', 'b2']) In [29]: s = Series(range(4), index=idx) In [30]: s Out[30]: a1 0 a2 1 b1 2 b2 3 dtype: int64 In [31]: idx.str.startswith('a') Out[31]: array([ True, True, False, False], dtype=bool) In [32]: s[s.index.str.startswith('a')] Out[32]: a1 0 a2 1 dtype: int64
The following new methods are accesible via .str accessor to apply the function to each values. (GH9766, GH9773, GH10031, GH10045, GH10052)
Methods capitalize() swapcase() normalize() partition() rpartition() index() rindex() translate() split now takes expand keyword to specify whether to expand dimensionality. return_type is deprecated. (GH9847)
In [33]: s = Series(['a,b', 'a,c', 'b,c']) # return Series In [34]: s.str.split(',') Out[34]: 0 [a, b] 1 [a, c] 2 [b, c] dtype: object # return DataFrame In [35]: s.str.split(',', expand=True) Out[35]: 0 1 0 a b 1 a c 2 b c In [36]: idx = Index(['a,b', 'a,c', 'b,c']) # return Index In [37]: idx.str.split(',') Out[37]: Index([[u'a', u'b'], [u'a', u'c'], [u'b', u'c']], dtype='object') # return MultiIndex In [38]: idx.str.split(',', expand=True) Out[38]: MultiIndex(levels=[[u'a', u'b'], [u'b', u'c']], labels=[[0, 0, 1], [0, 1, 1]])
Improved extract and get_dummies methods for Index.str (GH9980)
Other Enhancements¶
BusinessHour offset is now supported, which represents business hours starting from 09:00 - 17:00 on BusinessDay by default. See Here for details. (GH7905)
In [39]: from pandas.tseries.offsets import BusinessHour In [40]: Timestamp('2014-08-01 09:00') + BusinessHour() Out[40]: Timestamp('2014-08-01 10:00:00') In [41]: Timestamp('2014-08-01 07:00') + BusinessHour() Out[41]: Timestamp('2014-08-01 10:00:00') In [42]: Timestamp('2014-08-01 16:30') + BusinessHour() Out[42]: Timestamp('2014-08-04 09:30:00')
DataFrame.diff now takes an axis parameter that determines the direction of differencing (GH9727)
Allow clip, clip_lower, and clip_upper to accept array-like arguments as thresholds (This is a regression from 0.11.0). These methods now have an axis parameter which determines how the Series or DataFrame will be aligned with the threshold(s). (GH6966)
DataFrame.mask() and Series.mask() now support same keywords as where (GH8801)
drop function can now accept errors keyword to suppress ValueError raised when any of label does not exist in the target data. (GH6736)
In [43]: df = DataFrame(np.random.randn(3, 3), columns=['A', 'B', 'C']) In [44]: df.drop(['A', 'X'], axis=1, errors='ignore') Out[44]: B C 0 0.991946 0.953324 1 -0.334077 0.002118 2 0.289092 1.321158
Add support for separating years and quarters using dashes, for example 2014-Q1. (GH9688)
Allow conversion of values with dtype datetime64 or timedelta64 to strings using astype(str) (GH9757)
get_dummies function now accepts sparse keyword. If set to True, the return DataFrame is sparse, e.g. SparseDataFrame. (GH8823)
Period now accepts datetime64 as value input. (GH9054)
Allow timedelta string conversion when leading zero is missing from time definition, ie 0:00:00 vs 00:00:00. (GH9570)
Allow Panel.shift with axis='items' (GH9890)
Trying to write an excel file now raises NotImplementedError if the DataFrame has a MultiIndex instead of writing a broken Excel file. (GH9794)
Allow Categorical.add_categories to accept Series or np.array. (GH9927)
Add/delete str/dt/cat accessors dynamically from __dir__. (GH9910)
Add normalize as a dt accessor method. (GH10047)
DataFrame and Series now have _constructor_expanddim property as overridable constructor for one higher dimensionality data. This should be used only when it is really needed, see here
pd.lib.infer_dtype now returns 'bytes' in Python 3 where appropriate. (GH10032)
API changes¶
- When passing in an ax to df.plot( ..., ax=ax), the sharex kwarg will now default to False. The result is that the visibility of xlabels and xticklabels will not anymore be changed. You have to do that by yourself for the right axes in your figure or set sharex=True explicitly (but this changes the visible for all axes in the figure, not only the one which is passed in!). If pandas creates the subplots itself (e.g. no passed in ax kwarg), then the default is still sharex=True and the visibility changes are applied.
- assign() now inserts new columns in alphabetical order. Previously the order was arbitrary. (GH9777)
- By default, read_csv and read_table will now try to infer the compression type based on the file extension. Set compression=None to restore the previous behavior (no decompression). (GH9770)
Index Representation¶
The string representation of Index and its sub-classes have now been unified. These will show a single-line display if there are few values; a wrapped multi-line display for a lot of values (but less than display.max_seq_items; if lots of items (> display.max_seq_items) will show a truncated display (the head and tail of the data). The formatting for MultiIndex is unchanges (a multi-line wrapped display). The display width responds to the option display.max_seq_items, which is defaulted to 100. (GH6482)
Previous Behavior
In [2]: pd.Index(range(4),name='foo')
Out[2]: Int64Index([0, 1, 2, 3], dtype='int64')
In [3]: pd.Index(range(104),name='foo')
Out[3]: Int64Index([0, 1, 2, 3, 4, 5, 6, 7, 8, 9, 10, 11, 12, 13, 14, 15, 16, 17, 18, 19, 20, 21, 22, 23, 24, 25, 26, 27, 28, 29, 30, 31, 32, 33, 34, 35, 36, 37, 38, 39, 40, 41, 42, 43, 44, 45, 46, 47, 48, 49, 50, 51, 52, 53, 54, 55, 56, 57, 58, 59, 60, 61, 62, 63, 64, 65, 66, 67, 68, 69, 70, 71, 72, 73, 74, 75, 76, 77, 78, 79, 80, 81, 82, 83, 84, 85, 86, 87, 88, 89, 90, 91, 92, 93, 94, 95, 96, 97, 98, 99, ...], dtype='int64')
In [4]: pd.date_range('20130101',periods=4,name='foo',tz='US/Eastern')
Out[4]:
<class 'pandas.tseries.index.DatetimeIndex'>
[2013-01-01 00:00:00-05:00, ..., 2013-01-04 00:00:00-05:00]
Length: 4, Freq: D, Timezone: US/Eastern
In [5]: pd.date_range('20130101',periods=104,name='foo',tz='US/Eastern')
Out[5]:
<class 'pandas.tseries.index.DatetimeIndex'>
[2013-01-01 00:00:00-05:00, ..., 2013-04-14 00:00:00-04:00]
Length: 104, Freq: D, Timezone: US/Eastern
New Behavior
In [45]: pd.set_option('display.width', 80)
In [46]: pd.Index(range(4), name='foo')
Out[46]: Int64Index([0, 1, 2, 3], dtype='int64', name=u'foo')
In [47]: pd.Index(range(30), name='foo')
Out[47]:
Int64Index([ 0, 1, 2, 3, 4, 5, 6, 7, 8, 9, 10, 11, 12, 13, 14, 15, 16,
17, 18, 19, 20, 21, 22, 23, 24, 25, 26, 27, 28, 29],
dtype='int64', name=u'foo')
In [48]: pd.Index(range(104), name='foo')
Out[48]:
Int64Index([ 0, 1, 2, 3, 4, 5, 6, 7, 8, 9,
...
94, 95, 96, 97, 98, 99, 100, 101, 102, 103],
dtype='int64', name=u'foo', length=104)
In [49]: pd.CategoricalIndex(['a','bb','ccc','dddd'], ordered=True, name='foobar')
Out[49]: CategoricalIndex([u'a', u'bb', u'ccc', u'dddd'], categories=[u'a', u'bb', u'ccc', u'dddd'], ordered=True, name=u'foobar', dtype='category')
In [50]: pd.CategoricalIndex(['a','bb','ccc','dddd']*10, ordered=True, name='foobar')
Out[50]:
CategoricalIndex([u'a', u'bb', u'ccc', u'dddd', u'a', u'bb', u'ccc', u'dddd',
u'a', u'bb', u'ccc', u'dddd', u'a', u'bb', u'ccc', u'dddd',
u'a', u'bb', u'ccc', u'dddd', u'a', u'bb', u'ccc', u'dddd',
u'a', u'bb', u'ccc', u'dddd', u'a', u'bb', u'ccc', u'dddd',
u'a', u'bb', u'ccc', u'dddd', u'a', u'bb', u'ccc', u'dddd'],
categories=[u'a', u'bb', u'ccc', u'dddd'], ordered=True, name=u'foobar', dtype='category')
In [51]: pd.CategoricalIndex(['a','bb','ccc','dddd']*100, ordered=True, name='foobar')
Out[51]:
CategoricalIndex([u'a', u'bb', u'ccc', u'dddd', u'a', u'bb', u'ccc', u'dddd',
u'a', u'bb',
...
u'ccc', u'dddd', u'a', u'bb', u'ccc', u'dddd', u'a', u'bb',
u'ccc', u'dddd'],
categories=[u'a', u'bb', u'ccc', u'dddd'], ordered=True, name=u'foobar', dtype='category', length=400)
In [52]: pd.date_range('20130101',periods=4, name='foo', tz='US/Eastern')
Out[52]:
DatetimeIndex(['2013-01-01 00:00:00-05:00', '2013-01-02 00:00:00-05:00',
'2013-01-03 00:00:00-05:00', '2013-01-04 00:00:00-05:00'],
dtype='datetime64[ns, US/Eastern]', name=u'foo', freq='D')
In [53]: pd.date_range('20130101',periods=25, freq='D')
Out[53]:
DatetimeIndex(['2013-01-01', '2013-01-02', '2013-01-03', '2013-01-04',
'2013-01-05', '2013-01-06', '2013-01-07', '2013-01-08',
'2013-01-09', '2013-01-10', '2013-01-11', '2013-01-12',
'2013-01-13', '2013-01-14', '2013-01-15', '2013-01-16',
'2013-01-17', '2013-01-18', '2013-01-19', '2013-01-20',
'2013-01-21', '2013-01-22', '2013-01-23', '2013-01-24',
'2013-01-25'],
dtype='datetime64[ns]', freq='D')
In [54]: pd.date_range('20130101',periods=104, name='foo', tz='US/Eastern')
Out[54]:
DatetimeIndex(['2013-01-01 00:00:00-05:00', '2013-01-02 00:00:00-05:00',
'2013-01-03 00:00:00-05:00', '2013-01-04 00:00:00-05:00',
'2013-01-05 00:00:00-05:00', '2013-01-06 00:00:00-05:00',
'2013-01-07 00:00:00-05:00', '2013-01-08 00:00:00-05:00',
'2013-01-09 00:00:00-05:00', '2013-01-10 00:00:00-05:00',
...
'2013-04-05 00:00:00-04:00', '2013-04-06 00:00:00-04:00',
'2013-04-07 00:00:00-04:00', '2013-04-08 00:00:00-04:00',
'2013-04-09 00:00:00-04:00', '2013-04-10 00:00:00-04:00',
'2013-04-11 00:00:00-04:00', '2013-04-12 00:00:00-04:00',
'2013-04-13 00:00:00-04:00', '2013-04-14 00:00:00-04:00'],
dtype='datetime64[ns, US/Eastern]', name=u'foo', length=104, freq='D')
Performance Improvements¶
Bug Fixes¶
- Bug where labels did not appear properly in the legend of DataFrame.plot(), passing label= arguments works, and Series indices are no longer mutated. (GH9542)
- Bug in json serialization causing a segfault when a frame had zero length. (GH9805)
- Bug in read_csv where missing trailing delimiters would cause segfault. (GH5664)
- Bug in retaining index name on appending (GH9862)
- Bug in scatter_matrix draws unexpected axis ticklabels (GH5662)
- Fixed bug in StataWriter resulting in changes to input DataFrame upon save (GH9795).
- Bug in transform causing length mismatch when null entries were present and a fast aggregator was being used (GH9697)
- Bug in equals causing false negatives when block order differed (GH9330)
- Bug in grouping with multiple pd.Grouper where one is non-time based (GH10063)
- Bug in read_sql_table error when reading postgres table with timezone (GH7139)
- Bug in DataFrame slicing may not retain metadata (GH9776)
- Bug where TimdeltaIndex were not properly serialized in fixed HDFStore (GH9635)
- Bug with TimedeltaIndex constructor ignoring name when given another TimedeltaIndex as data (GH10025).
- Bug in DataFrameFormatter._get_formatted_index with not applying max_colwidth to the DataFrame index (GH7856)
- Bug in .loc with a read-only ndarray data source (GH10043)
- Bug in groupby.apply() that would raise if a passed user defined function either returned only None (for all input). (GH9685)
- Always use temporary files in pytables tests (GH9992)
- Bug in plotting continuously using secondary_y may not show legend properly. (GH9610, GH9779)
- Bug in DataFrame.plot(kind="hist") results in TypeError when DataFrame contains non-numeric columns (GH9853)
- Bug where repeated plotting of DataFrame with a DatetimeIndex may raise TypeError (GH9852)
- Bug in setup.py that would allow an incompat cython version to build (GH9827)
- Bug in plotting secondary_y incorrectly attaches right_ax property to secondary axes specifying itself recursively. (GH9861)
- Bug in Series.quantile on empty Series of type Datetime or Timedelta (GH9675)
- Bug in where causing incorrect results when upcasting was required (GH9731)
- Bug in FloatArrayFormatter where decision boundary for displaying “small” floats in decimal format is off by one order of magnitude for a given display.precision (GH9764)
- Fixed bug where DataFrame.plot() raised an error when both color and style keywords were passed and there was no color symbol in the style strings (GH9671)
- Not showing a DeprecationWarning on combining list-likes with an Index (GH10083)
- Bug in read_csv and read_table when using skip_rows parameter if blank lines are present. (GH9832)
- Bug in read_csv() interprets index_col=True as 1 (GH9798)
- Bug in index equality comparisons using == failing on Index/MultiIndex type incompatibility (GH9785)
- Bug in which SparseDataFrame could not take nan as a column name (GH8822)
- Bug in to_msgpack and read_msgpack zlib and blosc compression support (GH9783)
- Bug GroupBy.size doesn’t attach index name properly if grouped by TimeGrouper (GH9925)
- Bug causing an exception in slice assignments because length_of_indexer returns wrong results (GH9995)
- Bug in csv parser causing lines with initial whitespace plus one non-space character to be skipped. (GH9710)
- Bug in C csv parser causing spurious NaNs when data started with newline followed by whitespace. (GH10022)
- Bug causing elements with a null group to spill into the final group when grouping by a Categorical (GH9603)
- Bug where .iloc and .loc behavior is not consistent on empty dataframes (GH9964)
- Bug in invalid attribute access on a TimedeltaIndex incorrectly raised ValueError instead of AttributeError (GH9680)
- Bug in unequal comparisons between categorical data and a scalar, which was not in the categories (e.g. Series(Categorical(list("abc"), ordered=True)) > "d". This returned False for all elements, but now raises a TypeError. Equality comparisons also now return False for == and True for !=. (GH9848)
- Bug in DataFrame __setitem__ when right hand side is a dictionary (GH9874)
- Bug in where when dtype is datetime64/timedelta64, but dtype of other is not (GH9804)
- Bug in MultiIndex.sortlevel() results in unicode level name breaks (GH9856)
- Bug in which groupby.transform incorrectly enforced output dtypes to match input dtypes. (GH9807)
- Bug in DataFrame constructor when columns parameter is set, and data is an empty list (GH9939)
- Bug in bar plot with log=True raises TypeError if all values are less than 1 (GH9905)
- Bug in horizontal bar plot ignores log=True (GH9905)
- Bug in PyTables queries that did not return proper results using the index (GH8265, GH9676)
- Bug where dividing a dataframe containing values of type Decimal by another Decimal would raise. (GH9787)
- Bug where using DataFrames asfreq would remove the name of the index. (GH9885)
- Bug causing extra index point when resample BM/BQ (GH9756)
- Changed caching in AbstractHolidayCalendar to be at the instance level rather than at the class level as the latter can result in unexpected behaviour. (GH9552)
- Fixed latex output for multi-indexed dataframes (GH9778)
- Bug causing an exception when setting an empty range using DataFrame.loc (GH9596)
- Bug in hiding ticklabels with subplots and shared axes when adding a new plot to an existing grid of axes (GH9158)
- Bug in transform and filter when grouping on a categorical variable (GH9921)
- Bug in transform when groups are equal in number and dtype to the input index (GH9700)
- Google BigQuery connector now imports dependencies on a per-method basis.(GH9713)
- Updated BigQuery connector to no longer use deprecated oauth2client.tools.run() (GH8327)
- Bug in subclassed DataFrame. It may not return the correct class, when slicing or subsetting it. (GH9632)
- Bug in .median() where non-float null values are not handled correctly (GH10040)
- Bug in Series.fillna() where it raises if a numerically convertible string is given (GH10092)
v0.16.0 (March 22, 2015)¶
This is a major release from 0.15.2 and includes a small number of API changes, several new features, enhancements, and performance improvements along with a large number of bug fixes. We recommend that all users upgrade to this version.
Highlights include:
- DataFrame.assign method, see here
- Series.to_coo/from_coo methods to interact with scipy.sparse, see here
- Backwards incompatible change to Timedelta to conform the .seconds attribute with datetime.timedelta, see here
- Changes to the .loc slicing API to conform with the behavior of .ix see here
- Changes to the default for ordering in the Categorical constructor, see here
- Enhancement to the .str accessor to make string operations easier, see here
- The pandas.tools.rplot, pandas.sandbox.qtpandas and pandas.rpy modules are deprecated. We refer users to external packages like seaborn, pandas-qt and rpy2 for similar or equivalent functionality, see here
Check the API Changes and deprecations before updating.
What’s new in v0.16.0
New features¶
DataFrame Assign¶
Inspired by dplyr’s mutate verb, DataFrame has a new assign() method. The function signature for assign is simply **kwargs. The keys are the column names for the new fields, and the values are either a value to be inserted (for example, a Series or NumPy array), or a function of one argument to be called on the DataFrame. The new values are inserted, and the entire DataFrame (with all original and new columns) is returned.
In [1]: iris = read_csv('data/iris.data')
In [2]: iris.head()
Out[2]:
SepalLength SepalWidth PetalLength PetalWidth Name
0 5.1 3.5 1.4 0.2 Iris-setosa
1 4.9 3.0 1.4 0.2 Iris-setosa
2 4.7 3.2 1.3 0.2 Iris-setosa
3 4.6 3.1 1.5 0.2 Iris-setosa
4 5.0 3.6 1.4 0.2 Iris-setosa
In [3]: iris.assign(sepal_ratio=iris['SepalWidth'] / iris['SepalLength']).head()
Out[3]:
SepalLength SepalWidth PetalLength PetalWidth Name sepal_ratio
0 5.1 3.5 1.4 0.2 Iris-setosa 0.686275
1 4.9 3.0 1.4 0.2 Iris-setosa 0.612245
2 4.7 3.2 1.3 0.2 Iris-setosa 0.680851
3 4.6 3.1 1.5 0.2 Iris-setosa 0.673913
4 5.0 3.6 1.4 0.2 Iris-setosa 0.720000
Above was an example of inserting a precomputed value. We can also pass in a function to be evalutated.
In [4]: iris.assign(sepal_ratio = lambda x: (x['SepalWidth'] /
...: x['SepalLength'])).head()
...:
Out[4]:
SepalLength SepalWidth PetalLength PetalWidth Name sepal_ratio
0 5.1 3.5 1.4 0.2 Iris-setosa 0.686275
1 4.9 3.0 1.4 0.2 Iris-setosa 0.612245
2 4.7 3.2 1.3 0.2 Iris-setosa 0.680851
3 4.6 3.1 1.5 0.2 Iris-setosa 0.673913
4 5.0 3.6 1.4 0.2 Iris-setosa 0.720000
The power of assign comes when used in chains of operations. For example, we can limit the DataFrame to just those with a Sepal Length greater than 5, calculate the ratio, and plot
In [5]: (iris.query('SepalLength > 5')
...: .assign(SepalRatio = lambda x: x.SepalWidth / x.SepalLength,
...: PetalRatio = lambda x: x.PetalWidth / x.PetalLength)
...: .plot(kind='scatter', x='SepalRatio', y='PetalRatio'))
...:
Out[5]: <matplotlib.axes._subplots.AxesSubplot at 0x94922a4c>

See the documentation for more. (GH9229)
Interaction with scipy.sparse¶
Added SparseSeries.to_coo() and SparseSeries.from_coo() methods (GH8048) for converting to and from scipy.sparse.coo_matrix instances (see here). For example, given a SparseSeries with MultiIndex we can convert to a scipy.sparse.coo_matrix by specifying the row and column labels as index levels:
In [6]: from numpy import nan
In [7]: s = Series([3.0, nan, 1.0, 3.0, nan, nan])
In [8]: s.index = MultiIndex.from_tuples([(1, 2, 'a', 0),
...: (1, 2, 'a', 1),
...: (1, 1, 'b', 0),
...: (1, 1, 'b', 1),
...: (2, 1, 'b', 0),
...: (2, 1, 'b', 1)],
...: names=['A', 'B', 'C', 'D'])
...:
In [9]: s
Out[9]:
A B C D
1 2 a 0 3
1 NaN
1 b 0 1
1 3
2 1 b 0 NaN
1 NaN
dtype: float64
# SparseSeries
In [10]: ss = s.to_sparse()
In [11]: ss
Out[11]:
A B C D
1 2 a 0 3
1 NaN
1 b 0 1
1 3
2 1 b 0 NaN
1 NaN
dtype: float64
BlockIndex
Block locations: array([0, 2])
Block lengths: array([1, 2])
In [12]: A, rows, columns = ss.to_coo(row_levels=['A', 'B'],
....: column_levels=['C', 'D'],
....: sort_labels=False)
....:
In [13]: A
Out[13]:
<3x4 sparse matrix of type '<type 'numpy.float64'>'
with 3 stored elements in COOrdinate format>
In [14]: A.todense()
Out[14]:
matrix([[ 3., 0., 0., 0.],
[ 0., 0., 1., 3.],
[ 0., 0., 0., 0.]])
In [15]: rows
Out[15]: [(1L, 2L), (1L, 1L), (2L, 1L)]
In [16]: columns
Out[16]: [('a', 0L), ('a', 1L), ('b', 0L), ('b', 1L)]
The from_coo method is a convenience method for creating a SparseSeries from a scipy.sparse.coo_matrix:
In [17]: from scipy import sparse
In [18]: A = sparse.coo_matrix(([3.0, 1.0, 2.0], ([1, 0, 0], [0, 2, 3])),
....: shape=(3, 4))
....:
In [19]: A
Out[19]:
<3x4 sparse matrix of type '<type 'numpy.float64'>'
with 3 stored elements in COOrdinate format>
In [20]: A.todense()
Out[20]:
matrix([[ 0., 0., 1., 2.],
[ 3., 0., 0., 0.],
[ 0., 0., 0., 0.]])
In [21]: ss = SparseSeries.from_coo(A)
In [22]: ss
Out[22]:
0 2 1
3 2
1 0 3
dtype: float64
BlockIndex
Block locations: array([0])
Block lengths: array([3])
String Methods Enhancements¶
Following new methods are accesible via .str accessor to apply the function to each values. This is intended to make it more consistent with standard methods on strings. (GH9282, GH9352, GH9386, GH9387, GH9439)
Methods isalnum() isalpha() isdigit() isdigit() isspace() islower() isupper() istitle() isnumeric() isdecimal() find() rfind() ljust() rjust() zfill() In [23]: s = Series(['abcd', '3456', 'EFGH']) In [24]: s.str.isalpha() Out[24]: 0 True 1 False 2 True dtype: bool In [25]: s.str.find('ab') Out[25]: 0 0 1 -1 2 -1 dtype: int64
Series.str.pad() and Series.str.center() now accept fillchar option to specify filling character (GH9352)
In [26]: s = Series(['12', '300', '25']) In [27]: s.str.pad(5, fillchar='_') Out[27]: 0 ___12 1 __300 2 ___25 dtype: object
Added Series.str.slice_replace(), which previously raised NotImplementedError (GH8888)
In [28]: s = Series(['ABCD', 'EFGH', 'IJK']) In [29]: s.str.slice_replace(1, 3, 'X') Out[29]: 0 AXD 1 EXH 2 IX dtype: object # replaced with empty char In [30]: s.str.slice_replace(0, 1) Out[30]: 0 BCD 1 FGH 2 JK dtype: object
Other enhancements¶
Reindex now supports method='nearest' for frames or series with a monotonic increasing or decreasing index (GH9258):
In [31]: df = pd.DataFrame({'x': range(5)}) In [32]: df.reindex([0.2, 1.8, 3.5], method='nearest') Out[32]: x 0.2 0 1.8 2 3.5 4
This method is also exposed by the lower level Index.get_indexer and Index.get_loc methods.
The read_excel() function’s sheetname argument now accepts a list and None, to get multiple or all sheets respectively. If more than one sheet is specified, a dictionary is returned. (GH9450)
# Returns the 1st and 4th sheet, as a dictionary of DataFrames. pd.read_excel('path_to_file.xls',sheetname=['Sheet1',3])
Allow Stata files to be read incrementally with an iterator; support for long strings in Stata files. See the docs here (GH9493:).
Paths beginning with ~ will now be expanded to begin with the user’s home directory (GH9066)
Added time interval selection in get_data_yahoo (GH9071)
Added Timestamp.to_datetime64() to complement Timedelta.to_timedelta64() (GH9255)
tseries.frequencies.to_offset() now accepts Timedelta as input (GH9064)
Lag parameter was added to the autocorrelation method of Series, defaults to lag-1 autocorrelation (GH9192)
Timedelta will now accept nanoseconds keyword in constructor (GH9273)
SQL code now safely escapes table and column names (GH8986)
Added auto-complete for Series.str.<tab>, Series.dt.<tab> and Series.cat.<tab> (GH9322)
Index.get_indexer now supports method='pad' and method='backfill' even for any target array, not just monotonic targets. These methods also work for monotonic decreasing as well as monotonic increasing indexes (GH9258).
Index.asof now works on all index types (GH9258).
A verbose argument has been augmented in io.read_excel(), defaults to False. Set to True to print sheet names as they are parsed. (GH9450)
Added days_in_month (compatibility alias daysinmonth) property to Timestamp, DatetimeIndex, Period, PeriodIndex, and Series.dt (GH9572)
Added decimal option in to_csv to provide formatting for non-‘.’ decimal separators (GH781)
Added normalize option for Timestamp to normalized to midnight (GH8794)
Added example for DataFrame import to R using HDF5 file and rhdf5 library. See the documentation for more (GH9636).
Backwards incompatible API changes¶
Changes in Timedelta¶
In v0.15.0 a new scalar type Timedelta was introduced, that is a sub-class of datetime.timedelta. Mentioned here was a notice of an API change w.r.t. the .seconds accessor. The intent was to provide a user-friendly set of accessors that give the ‘natural’ value for that unit, e.g. if you had a Timedelta('1 day, 10:11:12'), then .seconds would return 12. However, this is at odds with the definition of datetime.timedelta, which defines .seconds as 10 * 3600 + 11 * 60 + 12 == 36672.
So in v0.16.0, we are restoring the API to match that of datetime.timedelta. Further, the component values are still available through the .components accessor. This affects the .seconds and .microseconds accessors, and removes the .hours, .minutes, .milliseconds accessors. These changes affect TimedeltaIndex and the Series .dt accessor as well. (GH9185, GH9139)
Previous Behavior
In [2]: t = pd.Timedelta('1 day, 10:11:12.100123')
In [3]: t.days
Out[3]: 1
In [4]: t.seconds
Out[4]: 12
In [5]: t.microseconds
Out[5]: 123
New Behavior
In [33]: t = pd.Timedelta('1 day, 10:11:12.100123')
In [34]: t.days
Out[34]: 1L
In [35]: t.seconds
Out[35]: 36672L
In [36]: t.microseconds
Out[36]: 100123L
Using .components allows the full component access
In [37]: t.components
Out[37]: Components(days=1L, hours=10L, minutes=11L, seconds=12L, milliseconds=100L, microseconds=123L, nanoseconds=0L)
In [38]: t.components.seconds
Out[38]: 12L
Indexing Changes¶
The behavior of a small sub-set of edge cases for using .loc have changed (GH8613). Furthermore we have improved the content of the error messages that are raised:
Slicing with .loc where the start and/or stop bound is not found in the index is now allowed; this previously would raise a KeyError. This makes the behavior the same as .ix in this case. This change is only for slicing, not when indexing with a single label.
In [39]: df = DataFrame(np.random.randn(5,4), ....: columns=list('ABCD'), ....: index=date_range('20130101',periods=5)) ....: In [40]: df Out[40]: A B C D 2013-01-01 -1.546906 -0.202646 -0.655969 0.193421 2013-01-02 0.553439 1.318152 -0.469305 0.675554 2013-01-03 -1.817027 -0.183109 1.058969 -0.397840 2013-01-04 0.337438 1.047579 1.045938 0.863717 2013-01-05 -0.122092 0.124713 -0.322795 0.841675 In [41]: s = Series(range(5),[-2,-1,1,2,3]) In [42]: s Out[42]: -2 0 -1 1 1 2 2 3 3 4 dtype: int64
Previous Behavior
In [4]: df.loc['2013-01-02':'2013-01-10'] KeyError: 'stop bound [2013-01-10] is not in the [index]' In [6]: s.loc[-10:3] KeyError: 'start bound [-10] is not the [index]'
New Behavior
In [43]: df.loc['2013-01-02':'2013-01-10'] Out[43]: A B C D 2013-01-02 0.553439 1.318152 -0.469305 0.675554 2013-01-03 -1.817027 -0.183109 1.058969 -0.397840 2013-01-04 0.337438 1.047579 1.045938 0.863717 2013-01-05 -0.122092 0.124713 -0.322795 0.841675 In [44]: s.loc[-10:3] Out[44]: -2 0 -1 1 1 2 2 3 3 4 dtype: int64
Allow slicing with float-like values on an integer index for .ix. Previously this was only enabled for .loc:
Previous Behavior
In [8]: s.ix[-1.0:2] TypeError: the slice start value [-1.0] is not a proper indexer for this index type (Int64Index)
New Behavior
In [45]: s.ix[-1.0:2] Out[45]: -1 1 1 2 2 3 dtype: int64
Provide a useful exception for indexing with an invalid type for that index when using .loc. For example trying to use .loc on an index of type DatetimeIndex or PeriodIndex or TimedeltaIndex, with an integer (or a float).
Previous Behavior
In [4]: df.loc[2:3] KeyError: 'start bound [2] is not the [index]'
New Behavior
In [4]: df.loc[2:3] TypeError: Cannot do slice indexing on <class 'pandas.tseries.index.DatetimeIndex'> with <type 'int'> keys
Categorical Changes¶
In prior versions, Categoricals that had an unspecified ordering (meaning no ordered keyword was passed) were defaulted as ordered Categoricals. Going forward, the ordered keyword in the Categorical constructor will default to False. Ordering must now be explicit.
Furthermore, previously you could change the ordered attribute of a Categorical by just setting the attribute, e.g. cat.ordered=True; This is now deprecated and you should use cat.as_ordered() or cat.as_unordered(). These will by default return a new object and not modify the existing object. (GH9347, GH9190)
Previous Behavior
In [3]: s = Series([0,1,2], dtype='category')
In [4]: s
Out[4]:
0 0
1 1
2 2
dtype: category
Categories (3, int64): [0 < 1 < 2]
In [5]: s.cat.ordered
Out[5]: True
In [6]: s.cat.ordered = False
In [7]: s
Out[7]:
0 0
1 1
2 2
dtype: category
Categories (3, int64): [0, 1, 2]
New Behavior
In [46]: s = Series([0,1,2], dtype='category')
In [47]: s
Out[47]:
0 0
1 1
2 2
dtype: category
Categories (3, int64): [0, 1, 2]
In [48]: s.cat.ordered
Out[48]: False
In [49]: s = s.cat.as_ordered()
In [50]: s
Out[50]:
0 0
1 1
2 2
dtype: category
Categories (3, int64): [0 < 1 < 2]
In [51]: s.cat.ordered
Out[51]: True
# you can set in the constructor of the Categorical
In [52]: s = Series(Categorical([0,1,2],ordered=True))
In [53]: s
Out[53]:
0 0
1 1
2 2
dtype: category
Categories (3, int64): [0 < 1 < 2]
In [54]: s.cat.ordered
Out[54]: True
For ease of creation of series of categorical data, we have added the ability to pass keywords when calling .astype(). These are passed directly to the constructor.
In [55]: s = Series(["a","b","c","a"]).astype('category',ordered=True)
In [56]: s
Out[56]:
0 a
1 b
2 c
3 a
dtype: category
Categories (3, object): [a < b < c]
In [57]: s = Series(["a","b","c","a"]).astype('category',categories=list('abcdef'),ordered=False)
In [58]: s
Out[58]:
0 a
1 b
2 c
3 a
dtype: category
Categories (6, object): [a, b, c, d, e, f]
Other API Changes¶
Index.duplicated now returns np.array(dtype=bool) rather than Index(dtype=object) containing bool values. (GH8875)
DataFrame.to_json now returns accurate type serialisation for each column for frames of mixed dtype (GH9037)
Previously data was coerced to a common dtype before serialisation, which for example resulted in integers being serialised to floats:
In [2]: pd.DataFrame({'i': [1,2], 'f': [3.0, 4.2]}).to_json() Out[2]: '{"f":{"0":3.0,"1":4.2},"i":{"0":1.0,"1":2.0}}'
Now each column is serialised using its correct dtype:
In [2]: pd.DataFrame({'i': [1,2], 'f': [3.0, 4.2]}).to_json() Out[2]: '{"f":{"0":3.0,"1":4.2},"i":{"0":1,"1":2}}'
DatetimeIndex, PeriodIndex and TimedeltaIndex.summary now output the same format. (GH9116)
TimedeltaIndex.freqstr now output the same string format as DatetimeIndex. (GH9116)
Bar and horizontal bar plots no longer add a dashed line along the info axis. The prior style can be achieved with matplotlib’s axhline or axvline methods (GH9088).
Series accessors .dt, .cat and .str now raise AttributeError instead of TypeError if the series does not contain the appropriate type of data (GH9617). This follows Python’s built-in exception hierarchy more closely and ensures that tests like hasattr(s, 'cat') are consistent on both Python 2 and 3.
Series now supports bitwise operation for integral types (GH9016). Previously even if the input dtypes were integral, the output dtype was coerced to bool.
Previous Behavior
In [2]: pd.Series([0,1,2,3], list('abcd')) | pd.Series([4,4,4,4], list('abcd')) Out[2]: a True b True c True d True dtype: bool
New Behavior. If the input dtypes are integral, the output dtype is also integral and the output values are the result of the bitwise operation.
In [2]: pd.Series([0,1,2,3], list('abcd')) | pd.Series([4,4,4,4], list('abcd')) Out[2]: a 4 b 5 c 6 d 7 dtype: int64
During division involving a Series or DataFrame, 0/0 and 0//0 now give np.nan instead of np.inf. (GH9144, GH8445)
Previous Behavior
In [2]: p = pd.Series([0, 1]) In [3]: p / 0 Out[3]: 0 inf 1 inf dtype: float64 In [4]: p // 0 Out[4]: 0 inf 1 inf dtype: float64
New Behavior
In [59]: p = pd.Series([0, 1]) In [60]: p / 0 Out[60]: 0 NaN 1 inf dtype: float64 In [61]: p // 0 Out[61]: 0 NaN 1 inf dtype: float64
Series.values_counts and Series.describe for categorical data will now put NaN entries at the end. (GH9443)
Series.describe for categorical data will now give counts and frequencies of 0, not NaN, for unused categories (GH9443)
Due to a bug fix, looking up a partial string label with DatetimeIndex.asof now includes values that match the string, even if they are after the start of the partial string label (GH9258).
Old behavior:
In [4]: pd.to_datetime(['2000-01-31', '2000-02-28']).asof('2000-02') Out[4]: Timestamp('2000-01-31 00:00:00')
Fixed behavior:
In [62]: pd.to_datetime(['2000-01-31', '2000-02-28']).asof('2000-02') Out[62]: Timestamp('2000-02-28 00:00:00')
To reproduce the old behavior, simply add more precision to the label (e.g., use 2000-02-01 instead of 2000-02).
Deprecations¶
- The rplot trellis plotting interface is deprecated and will be removed in a future version. We refer to external packages like seaborn for similar but more refined functionality (GH3445). The documentation includes some examples how to convert your existing code using rplot to seaborn: rplot docs.
- The pandas.sandbox.qtpandas interface is deprecated and will be removed in a future version. We refer users to the external package pandas-qt. (GH9615)
- The pandas.rpy interface is deprecated and will be removed in a future version. Similar functionaility can be accessed thru the rpy2 project (GH9602)
- Adding DatetimeIndex/PeriodIndex to another DatetimeIndex/PeriodIndex is being deprecated as a set-operation. This will be changed to a TypeError in a future version. .union() should be used for the union set operation. (GH9094)
- Subtracting DatetimeIndex/PeriodIndex from another DatetimeIndex/PeriodIndex is being deprecated as a set-operation. This will be changed to an actual numeric subtraction yielding a TimeDeltaIndex in a future version. .difference() should be used for the differencing set operation. (GH9094)
Removal of prior version deprecations/changes¶
- DataFrame.pivot_table and crosstab‘s rows and cols keyword arguments were removed in favor of index and columns (GH6581)
- DataFrame.to_excel and DataFrame.to_csv cols keyword argument was removed in favor of columns (GH6581)
- Removed convert_dummies in favor of get_dummies (GH6581)
- Removed value_range in favor of describe (GH6581)
Performance Improvements¶
- Fixed a performance regression for .loc indexing with an array or list-like (GH9126:).
- DataFrame.to_json 30x performance improvement for mixed dtype frames. (GH9037)
- Performance improvements in MultiIndex.duplicated by working with labels instead of values (GH9125)
- Improved the speed of nunique by calling unique instead of value_counts (GH9129, GH7771)
- Performance improvement of up to 10x in DataFrame.count and DataFrame.dropna by taking advantage of homogeneous/heterogeneous dtypes appropriately (GH9136)
- Performance improvement of up to 20x in DataFrame.count when using a MultiIndex and the level keyword argument (GH9163)
- Performance and memory usage improvements in merge when key space exceeds int64 bounds (GH9151)
- Performance improvements in multi-key groupby (GH9429)
- Performance improvements in MultiIndex.sortlevel (GH9445)
- Performance and memory usage improvements in DataFrame.duplicated (GH9398)
- Cythonized Period (GH9440)
- Decreased memory usage on to_hdf (GH9648)
Bug Fixes¶
- Changed .to_html to remove leading/trailing spaces in table body (GH4987)
- Fixed issue using read_csv on s3 with Python 3 (GH9452)
- Fixed compatibility issue in DatetimeIndex affecting architectures where numpy.int_ defaults to numpy.int32 (GH8943)
- Bug in Panel indexing with an object-like (GH9140)
- Bug in the returned Series.dt.components index was reset to the default index (GH9247)
- Bug in Categorical.__getitem__/__setitem__ with listlike input getting incorrect results from indexer coercion (GH9469)
- Bug in partial setting with a DatetimeIndex (GH9478)
- Bug in groupby for integer and datetime64 columns when applying an aggregator that caused the value to be changed when the number was sufficiently large (GH9311, GH6620)
- Fixed bug in to_sql when mapping a Timestamp object column (datetime column with timezone info) to the appropriate sqlalchemy type (GH9085).
- Fixed bug in to_sql dtype argument not accepting an instantiated SQLAlchemy type (GH9083).
- Bug in .loc partial setting with a np.datetime64 (GH9516)
- Incorrect dtypes inferred on datetimelike looking Series & on .xs slices (GH9477)
- Items in Categorical.unique() (and s.unique() if s is of dtype category) now appear in the order in which they are originally found, not in sorted order (GH9331). This is now consistent with the behavior for other dtypes in pandas.
- Fixed bug on big endian platforms which produced incorrect results in StataReader (GH8688).
- Bug in MultiIndex.has_duplicates when having many levels causes an indexer overflow (GH9075, GH5873)
- Bug in pivot and unstack where nan values would break index alignment (GH4862, GH7401, GH7403, GH7405, GH7466, GH9497)
- Bug in left join on multi-index with sort=True or null values (GH9210).
- Bug in MultiIndex where inserting new keys would fail (GH9250).
- Bug in groupby when key space exceeds int64 bounds (GH9096).
- Bug in unstack with TimedeltaIndex or DatetimeIndex and nulls (GH9491).
- Bug in rank where comparing floats with tolerance will cause inconsistent behaviour (GH8365).
- Fixed character encoding bug in read_stata and StataReader when loading data from a URL (GH9231).
- Bug in adding offsets.Nano to other offets raises TypeError (GH9284)
- Bug in DatetimeIndex iteration, related to (GH8890), fixed in (GH9100)
- Bugs in resample around DST transitions. This required fixing offset classes so they behave correctly on DST transitions. (GH5172, GH8744, GH8653, GH9173, GH9468).
- Bug in binary operator method (eg .mul()) alignment with integer levels (GH9463).
- Bug in boxplot, scatter and hexbin plot may show an unnecessary warning (GH8877)
- Bug in subplot with layout kw may show unnecessary warning (GH9464)
- Bug in using grouper functions that need passed thru arguments (e.g. axis), when using wrapped function (e.g. fillna), (GH9221)
- DataFrame now properly supports simultaneous copy and dtype arguments in constructor (GH9099)
- Bug in read_csv when using skiprows on a file with CR line endings with the c engine. (GH9079)
- isnull now detects NaT in PeriodIndex (GH9129)
- Bug in groupby .nth() with a multiple column groupby (GH8979)
- Bug in DataFrame.where and Series.where coerce numerics to string incorrectly (GH9280)
- Bug in DataFrame.where and Series.where raise ValueError when string list-like is passed. (GH9280)
- Accessing Series.str methods on with non-string values now raises TypeError instead of producing incorrect results (GH9184)
- Bug in DatetimeIndex.__contains__ when index has duplicates and is not monotonic increasing (GH9512)
- Fixed division by zero error for Series.kurt() when all values are equal (GH9197)
- Fixed issue in the xlsxwriter engine where it added a default ‘General’ format to cells if no other format wass applied. This prevented other row or column formatting being applied. (GH9167)
- Fixes issue with index_col=False when usecols is also specified in read_csv. (GH9082)
- Bug where wide_to_long would modify the input stubnames list (GH9204)
- Bug in to_sql not storing float64 values using double precision. (GH9009)
- SparseSeries and SparsePanel now accept zero argument constructors (same as their non-sparse counterparts) (GH9272).
- Regression in merging Categorical and object dtypes (GH9426)
- Bug in read_csv with buffer overflows with certain malformed input files (GH9205)
- Bug in groupby MultiIndex with missing pair (GH9049, GH9344)
- Fixed bug in Series.groupby where grouping on MultiIndex levels would ignore the sort argument (GH9444)
- Fix bug in DataFrame.Groupby where sort=False is ignored in the case of Categorical columns. (GH8868)
- Fixed bug with reading CSV files from Amazon S3 on python 3 raising a TypeError (GH9452)
- Bug in the Google BigQuery reader where the ‘jobComplete’ key may be present but False in the query results (GH8728)
- Bug in Series.values_counts with excluding NaN for categorical type Series with dropna=True (GH9443)
- Fixed mising numeric_only option for DataFrame.std/var/sem (GH9201)
- Support constructing Panel or Panel4D with scalar data (GH8285)
- Series text representation disconnected from max_rows/max_columns (GH7508).
Series number formatting inconsistent when truncated (GH8532).
Previous Behavior
In [2]: pd.options.display.max_rows = 10 In [3]: s = pd.Series([1,1,1,1,1,1,1,1,1,1,0.9999,1,1]*10) In [4]: s Out[4]: 0 1 1 1 2 1 ... 127 0.9999 128 1.0000 129 1.0000 Length: 130, dtype: float64
New Behavior
0 1.0000 1 1.0000 2 1.0000 3 1.0000 4 1.0000 ... 125 1.0000 126 1.0000 127 0.9999 128 1.0000 129 1.0000 dtype: float64
A Spurious SettingWithCopy Warning was generated when setting a new item in a frame in some cases (GH8730)
The following would previously report a SettingWithCopy Warning.
In [1]: df1 = DataFrame({'x': Series(['a','b','c']), 'y': Series(['d','e','f'])}) In [2]: df2 = df1[['x']] In [3]: df2['y'] = ['g', 'h', 'i']
v0.15.2 (December 12, 2014)¶
This is a minor release from 0.15.1 and includes a large number of bug fixes along with several new features, enhancements, and performance improvements. A small number of API changes were necessary to fix existing bugs. We recommend that all users upgrade to this version.
API changes¶
Indexing in MultiIndex beyond lex-sort depth is now supported, though a lexically sorted index will have a better performance. (GH2646)
In [1]: df = pd.DataFrame({'jim':[0, 0, 1, 1], ...: 'joe':['x', 'x', 'z', 'y'], ...: 'jolie':np.random.rand(4)}).set_index(['jim', 'joe']) ...: In [2]: df Out[2]: jolie jim joe 0 x 0.043324 x 0.561433 1 z 0.329668 y 0.502967 In [3]: df.index.lexsort_depth Out[3]: 1 # in prior versions this would raise a KeyError # will now show a PerformanceWarning In [4]: df.loc[(1, 'z')] Out[4]: jolie jim joe 1 z 0.329668 # lexically sorting In [5]: df2 = df.sortlevel() In [6]: df2 Out[6]: jolie jim joe 0 x 0.043324 x 0.561433 1 y 0.502967 z 0.329668 In [7]: df2.index.lexsort_depth Out[7]: 2 In [8]: df2.loc[(1,'z')] Out[8]: jolie jim joe 1 z 0.329668
Bug in unique of Series with category dtype, which returned all categories regardless whether they were “used” or not (see GH8559 for the discussion). Previous behaviour was to return all categories:
In [3]: cat = pd.Categorical(['a', 'b', 'a'], categories=['a', 'b', 'c']) In [4]: cat Out[4]: [a, b, a] Categories (3, object): [a < b < c] In [5]: cat.unique() Out[5]: array(['a', 'b', 'c'], dtype=object)
Now, only the categories that do effectively occur in the array are returned:
In [9]: cat = pd.Categorical(['a', 'b', 'a'], categories=['a', 'b', 'c']) In [10]: cat.unique() Out[10]: [a, b] Categories (2, object): [a, b]
Series.all and Series.any now support the level and skipna parameters. Series.all, Series.any, Index.all, and Index.any no longer support the out and keepdims parameters, which existed for compatibility with ndarray. Various index types no longer support the all and any aggregation functions and will now raise TypeError. (GH8302).
Allow equality comparisons of Series with a categorical dtype and object dtype; previously these would raise TypeError (GH8938)
Bug in NDFrame: conflicting attribute/column names now behave consistently between getting and setting. Previously, when both a column and attribute named y existed, data.y would return the attribute, while data.y = z would update the column (GH8994)
In [11]: data = pd.DataFrame({'x':[1, 2, 3]}) In [12]: data.y = 2 In [13]: data['y'] = [2, 4, 6] In [14]: data Out[14]: x y 0 1 2 1 2 4 2 3 6 # this assignment was inconsistent In [15]: data.y = 5
Old behavior:
In [6]: data.y Out[6]: 2 In [7]: data['y'].values Out[7]: array([5, 5, 5])
New behavior:
In [16]: data.y Out[16]: 5 In [17]: data['y'].values Out[17]: array([2, 4, 6], dtype=int64)
Timestamp('now') is now equivalent to Timestamp.now() in that it returns the local time rather than UTC. Also, Timestamp('today') is now equivalent to Timestamp.today() and both have tz as a possible argument. (GH9000)
Fix negative step support for label-based slices (GH8753)
Old behavior:
In [1]: s = pd.Series(np.arange(3), ['a', 'b', 'c']) Out[1]: a 0 b 1 c 2 dtype: int64 In [2]: s.loc['c':'a':-1] Out[2]: c 2 dtype: int64
New behavior:
In [18]: s = pd.Series(np.arange(3), ['a', 'b', 'c']) In [19]: s.loc['c':'a':-1] Out[19]: c 2 b 1 a 0 dtype: int32
Enhancements¶
Categorical enhancements:
- Added ability to export Categorical data to Stata (GH8633). See here for limitations of categorical variables exported to Stata data files.
- Added flag order_categoricals to StataReader and read_stata to select whether to order imported categorical data (GH8836). See here for more information on importing categorical variables from Stata data files.
- Added ability to export Categorical data to to/from HDF5 (GH7621). Queries work the same as if it was an object array. However, the category dtyped data is stored in a more efficient manner. See here for an example and caveats w.r.t. prior versions of pandas.
- Added support for searchsorted() on Categorical class (GH8420).
Other enhancements:
Added the ability to specify the SQL type of columns when writing a DataFrame to a database (GH8778). For example, specifying to use the sqlalchemy String type instead of the default Text type for string columns:
from sqlalchemy.types import String data.to_sql('data_dtype', engine, dtype={'Col_1': String})
Series.all and Series.any now support the level and skipna parameters (GH8302):
In [20]: s = pd.Series([False, True, False], index=[0, 0, 1]) In [21]: s.any(level=0) Out[21]: 0 True 1 False dtype: bool
Panel now supports the all and any aggregation functions. (GH8302):
In [22]: p = pd.Panel(np.random.rand(2, 5, 4) > 0.1) In [23]: p.all() Out[23]: 0 1 0 True False 1 True True 2 True True 3 False True
Added support for utcfromtimestamp(), fromtimestamp(), and combine() on Timestamp class (GH5351).
Added Google Analytics (pandas.io.ga) basic documentation (GH8835). See here.
Timedelta arithmetic returns NotImplemented in unknown cases, allowing extensions by custom classes (GH8813).
Timedelta now supports arithemtic with numpy.ndarray objects of the appropriate dtype (numpy 1.8 or newer only) (GH8884).
Added Timedelta.to_timedelta64() method to the public API (GH8884).
Added gbq.generate_bq_schema() function to the gbq module (GH8325).
Series now works with map objects the same way as generators (GH8909).
Added context manager to HDFStore for automatic closing (GH8791).
to_datetime gains an exact keyword to allow for a format to not require an exact match for a provided format string (if its False). exact defaults to True (meaning that exact matching is still the default) (GH8904)
Added axvlines boolean option to parallel_coordinates plot function, determines whether vertical lines will be printed, default is True
Added ability to read table footers to read_html (GH8552)
to_sql now infers datatypes of non-NA values for columns that contain NA values and have dtype object (GH8778).
Performance¶
Bug Fixes¶
- Bug in concat of Series with category dtype which were coercing to object. (GH8641)
- Bug in Timestamp-Timestamp not returning a Timedelta type and datelike-datelike ops with timezones (GH8865)
- Made consistent a timezone mismatch exception (either tz operated with None or incompatible timezone), will now return TypeError rather than ValueError (a couple of edge cases only), (GH8865)
- Bug in using a pd.Grouper(key=...) with no level/axis or level only (GH8795, GH8866)
- Report a TypeError when invalid/no paramaters are passed in a groupby (GH8015)
- Bug in packaging pandas with py2app/cx_Freeze (GH8602, GH8831)
- Bug in groupby signatures that didn’t include *args or **kwargs (GH8733).
- io.data.Options now raises RemoteDataError when no expiry dates are available from Yahoo and when it receives no data from Yahoo (GH8761), (GH8783).
- Unclear error message in csv parsing when passing dtype and names and the parsed data is a different data type (GH8833)
- Bug in slicing a multi-index with an empty list and at least one boolean indexer (GH8781)
- io.data.Options now raises RemoteDataError when no expiry dates are available from Yahoo (GH8761).
- Timedelta kwargs may now be numpy ints and floats (GH8757).
- Fixed several outstanding bugs for Timedelta arithmetic and comparisons (GH8813, GH5963, GH5436).
- sql_schema now generates dialect appropriate CREATE TABLE statements (GH8697)
- slice string method now takes step into account (GH8754)
- Bug in BlockManager where setting values with different type would break block integrity (GH8850)
- Bug in DatetimeIndex when using time object as key (GH8667)
- Bug in merge where how='left' and sort=False would not preserve left frame order (GH7331)
- Bug in MultiIndex.reindex where reindexing at level would not reorder labels (GH4088)
- Bug in certain operations with dateutil timezones, manifesting with dateutil 2.3 (GH8639)
- Regression in DatetimeIndex iteration with a Fixed/Local offset timezone (GH8890)
- Bug in to_datetime when parsing a nanoseconds using the %f format (GH8989)
- io.data.Options now raises RemoteDataError when no expiry dates are available from Yahoo and when it receives no data from Yahoo (GH8761), (GH8783).
- Fix: The font size was only set on x axis if vertical or the y axis if horizontal. (GH8765)
- Fixed division by 0 when reading big csv files in python 3 (GH8621)
- Bug in outputing a Multindex with to_html,index=False which would add an extra column (GH8452)
- Imported categorical variables from Stata files retain the ordinal information in the underlying data (GH8836).
- Defined .size attribute across NDFrame objects to provide compat with numpy >= 1.9.1; buggy with np.array_split (GH8846)
- Skip testing of histogram plots for matplotlib <= 1.2 (GH8648).
- Bug where get_data_google returned object dtypes (GH3995)
- Bug in DataFrame.stack(..., dropna=False) when the DataFrame’s columns is a MultiIndex whose labels do not reference all its levels. (GH8844)
- Bug in that Option context applied on __enter__ (GH8514)
- Bug in resample that causes a ValueError when resampling across multiple days and the last offset is not calculated from the start of the range (GH8683)
- Bug where DataFrame.plot(kind='scatter') fails when checking if an np.array is in the DataFrame (GH8852)
- Bug in pd.infer_freq/DataFrame.inferred_freq that prevented proper sub-daily frequency inference when the index contained DST days (GH8772).
- Bug where index name was still used when plotting a series with use_index=False (GH8558).
- Bugs when trying to stack multiple columns, when some (or all) of the level names are numbers (GH8584).
- Bug in MultiIndex where __contains__ returns wrong result if index is not lexically sorted or unique (GH7724)
- BUG CSV: fix problem with trailing whitespace in skipped rows, (GH8679), (GH8661), (GH8983)
- Regression in Timestamp does not parse ‘Z’ zone designator for UTC (GH8771)
- Bug in StataWriter the produces writes strings with 244 characters irrespective of actual size (GH8969)
- Fixed ValueError raised by cummin/cummax when datetime64 Series contains NaT. (GH8965)
- Bug in Datareader returns object dtype if there are missing values (GH8980)
- Bug in plotting if sharex was enabled and index was a timeseries, would show labels on multiple axes (GH3964).
- Bug where passing a unit to the TimedeltaIndex constructor applied the to nano-second conversion twice. (GH9011).
- Bug in plotting of a period-like array (GH9012)
v0.15.1 (November 9, 2014)¶
This is a minor bug-fix release from 0.15.0 and includes a small number of API changes, several new features, enhancements, and performance improvements along with a large number of bug fixes. We recommend that all users upgrade to this version.
API changes¶
s.dt.hour and other .dt accessors will now return np.nan for missing values (rather than previously -1), (GH8689)
In [1]: s = Series(date_range('20130101',periods=5,freq='D')) In [2]: s.iloc[2] = np.nan In [3]: s Out[3]: 0 2013-01-01 1 2013-01-02 2 NaT 3 2013-01-04 4 2013-01-05 dtype: datetime64[ns]
previous behavior:
In [6]: s.dt.hour Out[6]: 0 0 1 0 2 -1 3 0 4 0 dtype: int64
current behavior:
In [4]: s.dt.hour Out[4]: 0 0 1 0 2 NaN 3 0 4 0 dtype: float64
groupby with as_index=False will not add erroneous extra columns to result (GH8582):
In [5]: np.random.seed(2718281) In [6]: df = pd.DataFrame(np.random.randint(0, 100, (10, 2)), ...: columns=['jim', 'joe']) ...: In [7]: df.head() Out[7]: jim joe 0 61 81 1 96 49 2 55 65 3 72 51 4 77 12 In [8]: ts = pd.Series(5 * np.random.randint(0, 3, 10))
previous behavior:
In [4]: df.groupby(ts, as_index=False).max() Out[4]: NaN jim joe 0 0 72 83 1 5 77 84 2 10 96 65
current behavior:
In [9]: df.groupby(ts, as_index=False).max() Out[9]: jim joe 0 72 83 1 77 84 2 96 65
groupby will not erroneously exclude columns if the column name conflics with the grouper name (GH8112):
In [10]: df = pd.DataFrame({'jim': range(5), 'joe': range(5, 10)}) In [11]: df Out[11]: jim joe 0 0 5 1 1 6 2 2 7 3 3 8 4 4 9 In [12]: gr = df.groupby(df['jim'] < 2)
previous behavior (excludes 1st column from output):
In [4]: gr.apply(sum) Out[4]: joe jim False 24 True 11
current behavior:
In [13]: gr.apply(sum) Out[13]: jim joe jim False 9 24 True 1 11
Support for slicing with monotonic decreasing indexes, even if start or stop is not found in the index (GH7860):
In [14]: s = pd.Series(['a', 'b', 'c', 'd'], [4, 3, 2, 1]) In [15]: s Out[15]: 4 a 3 b 2 c 1 d dtype: object
previous behavior:
In [8]: s.loc[3.5:1.5] KeyError: 3.5
current behavior:
In [16]: s.loc[3.5:1.5] Out[16]: 3 b 2 c dtype: object
io.data.Options has been fixed for a change in the format of the Yahoo Options page (GH8612), (GH8741)
Note
As a result of a change in Yahoo’s option page layout, when an expiry date is given, Options methods now return data for a single expiry date. Previously, methods returned all data for the selected month.
The month and year parameters have been undeprecated and can be used to get all options data for a given month.
If an expiry date that is not valid is given, data for the next expiry after the given date is returned.
Option data frames are now saved on the instance as callsYYMMDD or putsYYMMDD. Previously they were saved as callsMMYY and putsMMYY. The next expiry is saved as calls and puts.
New features:
- The expiry parameter can now be a single date or a list-like object containing dates.
- A new property expiry_dates was added, which returns all available expiry dates.
Current behavior:
In [17]: from pandas.io.data import Options In [18]: aapl = Options('aapl','yahoo') In [19]: aapl.get_call_data().iloc[0:5,0:1] Out[19]: Last Strike Expiry Type Symbol 80 2015-11-27 call AAPL151127C00080000 34.16 90 2015-11-27 call AAPL151127C00090000 32.39 95 2015-11-27 call AAPL151127C00095000 21.45 96 2015-11-27 call AAPL151127C00096000 26.42 97 2015-11-27 call AAPL151127C00097000 21.90 In [20]: aapl.expiry_dates Out[20]: [datetime.date(2015, 11, 27), datetime.date(2015, 12, 4), datetime.date(2015, 12, 11), datetime.date(2015, 12, 18), datetime.date(2015, 12, 24), datetime.date(2015, 12, 31), datetime.date(2016, 1, 15), datetime.date(2016, 2, 19), datetime.date(2016, 4, 15), datetime.date(2016, 6, 17), datetime.date(2016, 7, 15), datetime.date(2017, 1, 20), datetime.date(2018, 1, 19)] In [21]: aapl.get_near_stock_price(expiry=aapl.expiry_dates[0:3]).iloc[0:5,0:1] Out[21]: Last Strike Expiry Type Symbol 119 2015-12-04 call AAPL151204C00119000 1.95 2015-12-11 call AAPL151211C00119000 2.50 120 2015-11-27 call AAPL151127C00120000 0.78 2015-12-04 call AAPL151204C00120000 1.47 2015-12-11 call AAPL151211C00120000 2.00
See the Options documentation in Remote Data
- pandas now also registers the datetime64 dtype in matplotlib’s units registry to plot such values as datetimes. This is activated once pandas is imported. In previous versions, plotting an array of datetime64 values will have resulted in plotted integer values. To keep the previous behaviour, you can do del matplotlib.units.registry[np.datetime64] (GH8614).
Enhancements¶
concat permits a wider variety of iterables of pandas objects to be passed as the first parameter (GH8645):
In [22]: from collections import deque In [23]: df1 = pd.DataFrame([1, 2, 3]) In [24]: df2 = pd.DataFrame([4, 5, 6])
previous behavior:
In [7]: pd.concat(deque((df1, df2))) TypeError: first argument must be a list-like of pandas objects, you passed an object of type "deque"
current behavior:
In [25]: pd.concat(deque((df1, df2))) Out[25]: 0 0 1 1 2 2 3 0 4 1 5 2 6
Represent MultiIndex labels with a dtype that utilizes memory based on the level size. In prior versions, the memory usage was a constant 8 bytes per element in each level. In addition, in prior versions, the reported memory usage was incorrect as it didn’t show the usage for the memory occupied by the underling data array. (GH8456)
In [26]: dfi = DataFrame(1,index=pd.MultiIndex.from_product([['a'],range(1000)]),columns=['A'])
previous behavior:
# this was underreported in prior versions In [1]: dfi.memory_usage(index=True) Out[1]: Index 8000 # took about 24008 bytes in < 0.15.1 A 8000 dtype: int64
current behavior:
In [27]: dfi.memory_usage(index=True) Out[27]: Index 4000 A 8000 dtype: int64
Added Index properties is_monotonic_increasing and is_monotonic_decreasing (GH8680).
Added option to select columns when importing Stata files (GH7935)
Qualify memory usage in DataFrame.info() by adding + if it is a lower bound (GH8578)
Raise errors in certain aggregation cases where an argument such as numeric_only is not handled (GH8592).
Added support for 3-character ISO and non-standard country codes in io.wb.download() (GH8482)
World Bank data requests now will warn/raise based on an errors argument, as well as a list of hard-coded country codes and the World Bank’s JSON response. In prior versions, the error messages didn’t look at the World Bank’s JSON response. Problem-inducing input were simply dropped prior to the request. The issue was that many good countries were cropped in the hard-coded approach. All countries will work now, but some bad countries will raise exceptions because some edge cases break the entire response. (GH8482)
Added option to Series.str.split() to return a DataFrame rather than a Series (GH8428)
Added option to df.info(null_counts=None|True|False) to override the default display options and force showing of the null-counts (GH8701)
Bug Fixes¶
- Bug in unpickling of a CustomBusinessDay object (GH8591)
- Bug in coercing Categorical to a records array, e.g. df.to_records() (GH8626)
- Bug in Categorical not created properly with Series.to_frame() (GH8626)
- Bug in coercing in astype of a Categorical of a passed pd.Categorical (this now raises TypeError correctly), (GH8626)
- Bug in cut/qcut when using Series and retbins=True (GH8589)
- Bug in writing Categorical columns to an SQL database with to_sql (GH8624).
- Bug in comparing Categorical of datetime raising when being compared to a scalar datetime (GH8687)
- Bug in selecting from a Categorical with .iloc (GH8623)
- Bug in groupby-transform with a Categorical (GH8623)
- Bug in duplicated/drop_duplicates with a Categorical (GH8623)
- Bug in Categorical reflected comparison operator raising if the first argument was a numpy array scalar (e.g. np.int64) (GH8658)
- Bug in Panel indexing with a list-like (GH8710)
- Compat issue is DataFrame.dtypes when options.mode.use_inf_as_null is True (GH8722)
- Bug in read_csv, dialect parameter would not take a string (:issue: 8703)
- Bug in slicing a multi-index level with an empty-list (GH8737)
- Bug in numeric index operations of add/sub with Float/Index Index with numpy arrays (GH8608)
- Bug in setitem with empty indexer and unwanted coercion of dtypes (GH8669)
- Bug in ix/loc block splitting on setitem (manifests with integer-like dtypes, e.g. datetime64) (GH8607)
- Bug when doing label based indexing with integers not found in the index for non-unique but monotonic indexes (GH8680).
- Bug when indexing a Float64Index with np.nan on numpy 1.7 (GH8980).
- Fix shape attribute for MultiIndex (GH8609)
- Bug in GroupBy where a name conflict between the grouper and columns would break groupby operations (GH7115, GH8112)
- Fixed a bug where plotting a column y and specifying a label would mutate the index name of the original DataFrame (GH8494)
- Fix regression in plotting of a DatetimeIndex directly with matplotlib (GH8614).
- Bug in date_range where partially-specified dates would incorporate current date (GH6961)
- Bug in Setting by indexer to a scalar value with a mixed-dtype Panel4d was failing (GH8702)
- Bug where DataReader‘s would fail if one of the symbols passed was invalid. Now returns data for valid symbols and np.nan for invalid (GH8494)
- Bug in get_quote_yahoo that wouldn’t allow non-float return values (GH5229).
v0.15.0 (October 18, 2014)¶
This is a major release from 0.14.1 and includes a small number of API changes, several new features, enhancements, and performance improvements along with a large number of bug fixes. We recommend that all users upgrade to this version.
Warning
pandas >= 0.15.0 will no longer support compatibility with NumPy versions < 1.7.0. If you want to use the latest versions of pandas, please upgrade to NumPy >= 1.7.0 (GH7711)
- Highlights include:
- The Categorical type was integrated as a first-class pandas type, see here
- New scalar type Timedelta, and a new index type TimedeltaIndex, see here
- New datetimelike properties accessor .dt for Series, see Datetimelike Properties
- New DataFrame default display for df.info() to include memory usage, see Memory Usage
- read_csv will now by default ignore blank lines when parsing, see here
- API change in using Indexes in set operations, see here
- Enhancements in the handling of timezones, see here
- A lot of improvements to the rolling and expanding moment funtions, see here
- Internal refactoring of the Index class to no longer sub-class ndarray, see Internal Refactoring
- dropping support for PyTables less than version 3.0.0, and numexpr less than version 2.1 (GH7990)
- Split indexing documentation into Indexing and Selecting Data and MultiIndex / Advanced Indexing
- Split out string methods documentation into Working with Text Data
- Check the API Changes and deprecations before updating
- Other Enhancements
- Performance Improvements
- Bug Fixes
Warning
In 0.15.0 Index has internally been refactored to no longer sub-class ndarray but instead subclass PandasObject, similarly to the rest of the pandas objects. This change allows very easy sub-classing and creation of new index types. This should be a transparent change with only very limited API implications (See the Internal Refactoring)
Warning
The refactorings in Categorical changed the two argument constructor from “codes/labels and levels” to “values and levels (now called ‘categories’)”. This can lead to subtle bugs. If you use Categorical directly, please audit your code before updating to this pandas version and change it to use the from_codes() constructor. See more on Categorical here
New features¶
Categoricals in Series/DataFrame¶
Categorical can now be included in Series and DataFrames and gained new methods to manipulate. Thanks to Jan Schulz for much of this API/implementation. (GH3943, GH5313, GH5314, GH7444, GH7839, GH7848, GH7864, GH7914, GH7768, GH8006, GH3678, GH8075, GH8076, GH8143, GH8453, GH8518).
For full docs, see the categorical introduction and the API documentation.
In [1]: df = DataFrame({"id":[1,2,3,4,5,6], "raw_grade":['a', 'b', 'b', 'a', 'a', 'e']})
In [2]: df["grade"] = df["raw_grade"].astype("category")
In [3]: df["grade"]
Out[3]:
0 a
1 b
2 b
3 a
4 a
5 e
Name: grade, dtype: category
Categories (3, object): [a, b, e]
# Rename the categories
In [4]: df["grade"].cat.categories = ["very good", "good", "very bad"]
# Reorder the categories and simultaneously add the missing categories
In [5]: df["grade"] = df["grade"].cat.set_categories(["very bad", "bad", "medium", "good", "very good"])
In [6]: df["grade"]
Out[6]:
0 very good
1 good
2 good
3 very good
4 very good
5 very bad
Name: grade, dtype: category
Categories (5, object): [very bad, bad, medium, good, very good]
In [7]: df.sort("grade")
Out[7]:
id raw_grade grade
5 6 e very bad
1 2 b good
2 3 b good
0 1 a very good
3 4 a very good
4 5 a very good
In [8]: df.groupby("grade").size()
Out[8]:
grade
very bad 1
bad 0
medium 0
good 2
very good 3
dtype: int64
- pandas.core.group_agg and pandas.core.factor_agg were removed. As an alternative, construct a dataframe and use df.groupby(<group>).agg(<func>).
- Supplying “codes/labels and levels” to the Categorical constructor is not supported anymore. Supplying two arguments to the constructor is now interpreted as “values and levels (now called ‘categories’)”. Please change your code to use the from_codes() constructor.
- The Categorical.labels attribute was renamed to Categorical.codes and is read only. If you want to manipulate codes, please use one of the API methods on Categoricals.
- The Categorical.levels attribute is renamed to Categorical.categories.
TimedeltaIndex/Scalar¶
We introduce a new scalar type Timedelta, which is a subclass of datetime.timedelta, and behaves in a similar manner, but allows compatibility with np.timedelta64 types as well as a host of custom representation, parsing, and attributes. This type is very similar to how Timestamp works for datetimes. It is a nice-API box for the type. See the docs. (GH3009, GH4533, GH8209, GH8187, GH8190, GH7869, GH7661, GH8345, GH8471)
Warning
Timedelta scalars (and TimedeltaIndex) component fields are not the same as the component fields on a datetime.timedelta object. For example, .seconds on a datetime.timedelta object returns the total number of seconds combined between hours, minutes and seconds. In contrast, the pandas Timedelta breaks out hours, minutes, microseconds and nanoseconds separately.
# Timedelta accessor
In [9]: tds = Timedelta('31 days 5 min 3 sec')
In [10]: tds.minutes
Out[10]: 5L
In [11]: tds.seconds
Out[11]: 3L
# datetime.timedelta accessor
# this is 5 minutes * 60 + 3 seconds
In [12]: tds.to_pytimedelta().seconds
Out[12]: 303
Note: this is no longer true starting from v0.16.0, where full compatibility with datetime.timedelta is introduced. See the 0.16.0 whatsnew entry
Warning
Prior to 0.15.0 pd.to_timedelta would return a Series for list-like/Series input, and a np.timedelta64 for scalar input. It will now return a TimedeltaIndex for list-like input, Series for Series input, and Timedelta for scalar input.
The arguments to pd.to_timedelta are now (arg,unit='ns',box=True,coerce=False), previously were (arg,box=True,unit='ns') as these are more logical.
Consruct a scalar
In [9]: Timedelta('1 days 06:05:01.00003')
Out[9]: Timedelta('1 days 06:05:01.000030')
In [10]: Timedelta('15.5us')
Out[10]: Timedelta('0 days 00:00:00.000015')
In [11]: Timedelta('1 hour 15.5us')
Out[11]: Timedelta('0 days 01:00:00.000015')
# negative Timedeltas have this string repr
# to be more consistent with datetime.timedelta conventions
In [12]: Timedelta('-1us')
Out[12]: Timedelta('-1 days +23:59:59.999999')
# a NaT
In [13]: Timedelta('nan')
Out[13]: NaT
Access fields for a Timedelta
In [14]: td = Timedelta('1 hour 3m 15.5us')
In [15]: td.seconds
Out[15]: 3780L
In [16]: td.microseconds
Out[16]: 15L
In [17]: td.nanoseconds
Out[17]: 500L
Construct a TimedeltaIndex
In [18]: TimedeltaIndex(['1 days','1 days, 00:00:05',
....: np.timedelta64(2,'D'),timedelta(days=2,seconds=2)])
....:
Out[18]:
TimedeltaIndex(['1 days 00:00:00', '1 days 00:00:05', '2 days 00:00:00',
'2 days 00:00:02'],
dtype='timedelta64[ns]', freq=None)
Constructing a TimedeltaIndex with a regular range
In [19]: timedelta_range('1 days',periods=5,freq='D')
Out[19]: TimedeltaIndex(['1 days', '2 days', '3 days', '4 days', '5 days'], dtype='timedelta64[ns]', freq='D')
In [20]: timedelta_range(start='1 days',end='2 days',freq='30T')
Out[20]:
TimedeltaIndex(['1 days 00:00:00', '1 days 00:30:00', '1 days 01:00:00',
'1 days 01:30:00', '1 days 02:00:00', '1 days 02:30:00',
'1 days 03:00:00', '1 days 03:30:00', '1 days 04:00:00',
'1 days 04:30:00', '1 days 05:00:00', '1 days 05:30:00',
'1 days 06:00:00', '1 days 06:30:00', '1 days 07:00:00',
'1 days 07:30:00', '1 days 08:00:00', '1 days 08:30:00',
'1 days 09:00:00', '1 days 09:30:00', '1 days 10:00:00',
'1 days 10:30:00', '1 days 11:00:00', '1 days 11:30:00',
'1 days 12:00:00', '1 days 12:30:00', '1 days 13:00:00',
'1 days 13:30:00', '1 days 14:00:00', '1 days 14:30:00',
'1 days 15:00:00', '1 days 15:30:00', '1 days 16:00:00',
'1 days 16:30:00', '1 days 17:00:00', '1 days 17:30:00',
'1 days 18:00:00', '1 days 18:30:00', '1 days 19:00:00',
'1 days 19:30:00', '1 days 20:00:00', '1 days 20:30:00',
'1 days 21:00:00', '1 days 21:30:00', '1 days 22:00:00',
'1 days 22:30:00', '1 days 23:00:00', '1 days 23:30:00',
'2 days 00:00:00'],
dtype='timedelta64[ns]', freq='30T')
You can now use a TimedeltaIndex as the index of a pandas object
In [21]: s = Series(np.arange(5),
....: index=timedelta_range('1 days',periods=5,freq='s'))
....:
In [22]: s
Out[22]:
1 days 00:00:00 0
1 days 00:00:01 1
1 days 00:00:02 2
1 days 00:00:03 3
1 days 00:00:04 4
Freq: S, dtype: int32
You can select with partial string selections
In [23]: s['1 day 00:00:02']
Out[23]: 2
In [24]: s['1 day':'1 day 00:00:02']
Out[24]:
1 days 00:00:00 0
1 days 00:00:01 1
1 days 00:00:02 2
Freq: S, dtype: int32
Finally, the combination of TimedeltaIndex with DatetimeIndex allow certain combination operations that are NaT preserving:
In [25]: tdi = TimedeltaIndex(['1 days',pd.NaT,'2 days'])
In [26]: tdi.tolist()
Out[26]: [Timedelta('1 days 00:00:00'), NaT, Timedelta('2 days 00:00:00')]
In [27]: dti = date_range('20130101',periods=3)
In [28]: dti.tolist()
Out[28]:
[Timestamp('2013-01-01 00:00:00', offset='D'),
Timestamp('2013-01-02 00:00:00', offset='D'),
Timestamp('2013-01-03 00:00:00', offset='D')]
In [29]: (dti + tdi).tolist()
Out[29]: [Timestamp('2013-01-02 00:00:00'), NaT, Timestamp('2013-01-05 00:00:00')]
In [30]: (dti - tdi).tolist()
Out[30]: [Timestamp('2012-12-31 00:00:00'), NaT, Timestamp('2013-01-01 00:00:00')]
- iteration of a Series e.g. list(Series(...)) of timedelta64[ns] would prior to v0.15.0 return np.timedelta64 for each element. These will now be wrapped in Timedelta.
Memory Usage¶
Implemented methods to find memory usage of a DataFrame. See the FAQ for more. (GH6852).
A new display option display.memory_usage (see Options and Settings) sets the default behavior of the memory_usage argument in the df.info() method. By default display.memory_usage is True.
In [31]: dtypes = ['int64', 'float64', 'datetime64[ns]', 'timedelta64[ns]',
....: 'complex128', 'object', 'bool']
....:
In [32]: n = 5000
In [33]: data = dict([ (t, np.random.randint(100, size=n).astype(t))
....: for t in dtypes])
....:
In [34]: df = DataFrame(data)
In [35]: df['categorical'] = df['object'].astype('category')
In [36]: df.info()
<class 'pandas.core.frame.DataFrame'>
Int64Index: 5000 entries, 0 to 4999
Data columns (total 8 columns):
bool 5000 non-null bool
complex128 5000 non-null complex128
datetime64[ns] 5000 non-null datetime64[ns]
float64 5000 non-null float64
int64 5000 non-null int64
object 5000 non-null object
timedelta64[ns] 5000 non-null timedelta64[ns]
categorical 5000 non-null category
dtypes: bool(1), category(1), complex128(1), datetime64[ns](1), float64(1), int64(1), object(1), timedelta64[ns](1)
memory usage: 303.5+ KB
Additionally memory_usage() is an available method for a dataframe object which returns the memory usage of each column.
In [37]: df.memory_usage(index=True)
Out[37]:
Index 40000
bool 5000
complex128 80000
datetime64[ns] 40000
float64 40000
int64 40000
object 20000
timedelta64[ns] 40000
categorical 5800
dtype: int64
.dt accessor¶
Series has gained an accessor to succinctly return datetime like properties for the values of the Series, if its a datetime/period like Series. (GH7207) This will return a Series, indexed like the existing Series. See the docs
# datetime
In [38]: s = Series(date_range('20130101 09:10:12',periods=4))
In [39]: s
Out[39]:
0 2013-01-01 09:10:12
1 2013-01-02 09:10:12
2 2013-01-03 09:10:12
3 2013-01-04 09:10:12
dtype: datetime64[ns]
In [40]: s.dt.hour
Out[40]:
0 9
1 9
2 9
3 9
dtype: int64
In [41]: s.dt.second
Out[41]:
0 12
1 12
2 12
3 12
dtype: int64
In [42]: s.dt.day
Out[42]:
0 1
1 2
2 3
3 4
dtype: int64
In [43]: s.dt.freq
Out[43]: <Day>
This enables nice expressions like this:
In [44]: s[s.dt.day==2]
Out[44]:
1 2013-01-02 09:10:12
dtype: datetime64[ns]
You can easily produce tz aware transformations:
In [45]: stz = s.dt.tz_localize('US/Eastern')
In [46]: stz
Out[46]:
0 2013-01-01 09:10:12-05:00
1 2013-01-02 09:10:12-05:00
2 2013-01-03 09:10:12-05:00
3 2013-01-04 09:10:12-05:00
dtype: datetime64[ns, US/Eastern]
In [47]: stz.dt.tz
Out[47]: <DstTzInfo 'US/Eastern' LMT-1 day, 19:04:00 STD>
You can also chain these types of operations:
In [48]: s.dt.tz_localize('UTC').dt.tz_convert('US/Eastern')
Out[48]:
0 2013-01-01 04:10:12-05:00
1 2013-01-02 04:10:12-05:00
2 2013-01-03 04:10:12-05:00
3 2013-01-04 04:10:12-05:00
dtype: datetime64[ns, US/Eastern]
The .dt accessor works for period and timedelta dtypes.
# period
In [49]: s = Series(period_range('20130101',periods=4,freq='D'))
In [50]: s
Out[50]:
0 2013-01-01
1 2013-01-02
2 2013-01-03
3 2013-01-04
dtype: object
In [51]: s.dt.year
Out[51]:
0 2013
1 2013
2 2013
3 2013
dtype: int64
In [52]: s.dt.day
Out[52]:
0 1
1 2
2 3
3 4
dtype: int64
# timedelta
In [53]: s = Series(timedelta_range('1 day 00:00:05',periods=4,freq='s'))
In [54]: s
Out[54]:
0 1 days 00:00:05
1 1 days 00:00:06
2 1 days 00:00:07
3 1 days 00:00:08
dtype: timedelta64[ns]
In [55]: s.dt.days
Out[55]:
0 1
1 1
2 1
3 1
dtype: int64
In [56]: s.dt.seconds
Out[56]:
0 5
1 6
2 7
3 8
dtype: int64
In [57]: s.dt.components
Out[57]:
days hours minutes seconds milliseconds microseconds nanoseconds
0 1 0 0 5 0 0 0
1 1 0 0 6 0 0 0
2 1 0 0 7 0 0 0
3 1 0 0 8 0 0 0
Timezone handling improvements¶
tz_localize(None) for tz-aware Timestamp and DatetimeIndex now removes timezone holding local time, previously this resulted in Exception or TypeError (GH7812)
In [58]: ts = Timestamp('2014-08-01 09:00', tz='US/Eastern') In [59]: ts Out[59]: Timestamp('2014-08-01 09:00:00-0400', tz='US/Eastern') In [60]: ts.tz_localize(None) Out[60]: Timestamp('2014-08-01 09:00:00') In [61]: didx = DatetimeIndex(start='2014-08-01 09:00', freq='H', periods=10, tz='US/Eastern') In [62]: didx Out[62]: DatetimeIndex(['2014-08-01 09:00:00-04:00', '2014-08-01 10:00:00-04:00', '2014-08-01 11:00:00-04:00', '2014-08-01 12:00:00-04:00', '2014-08-01 13:00:00-04:00', '2014-08-01 14:00:00-04:00', '2014-08-01 15:00:00-04:00', '2014-08-01 16:00:00-04:00', '2014-08-01 17:00:00-04:00', '2014-08-01 18:00:00-04:00'], dtype='datetime64[ns, US/Eastern]', freq='H') In [63]: didx.tz_localize(None) Out[63]: DatetimeIndex(['2014-08-01 09:00:00', '2014-08-01 10:00:00', '2014-08-01 11:00:00', '2014-08-01 12:00:00', '2014-08-01 13:00:00', '2014-08-01 14:00:00', '2014-08-01 15:00:00', '2014-08-01 16:00:00', '2014-08-01 17:00:00', '2014-08-01 18:00:00'], dtype='datetime64[ns]', freq='H')
tz_localize now accepts the ambiguous keyword which allows for passing an array of bools indicating whether the date belongs in DST or not, ‘NaT’ for setting transition times to NaT, ‘infer’ for inferring DST/non-DST, and ‘raise’ (default) for an AmbiguousTimeError to be raised. See the docs for more details (GH7943)
DataFrame.tz_localize and DataFrame.tz_convert now accepts an optional level argument for localizing a specific level of a MultiIndex (GH7846)
Timestamp.tz_localize and Timestamp.tz_convert now raise TypeError in error cases, rather than Exception (GH8025)
a timeseries/index localized to UTC when inserted into a Series/DataFrame will preserve the UTC timezone (rather than being a naive datetime64[ns]) as object dtype (GH8411)
Timestamp.__repr__ displays dateutil.tz.tzoffset info (GH7907)
Rolling/Expanding Moments improvements¶
rolling_min(), rolling_max(), rolling_cov(), and rolling_corr() now return objects with all NaN when len(arg) < min_periods <= window rather than raising. (This makes all rolling functions consistent in this behavior). (GH7766)
Prior to 0.15.0
In [64]: s = Series([10, 11, 12, 13])
In [15]: rolling_min(s, window=10, min_periods=5) ValueError: min_periods (5) must be <= window (4)
New behavior
In [65]: rolling_min(s, window=10, min_periods=5) Out[65]: 0 NaN 1 NaN 2 NaN 3 NaN dtype: float64
rolling_max(), rolling_min(), rolling_sum(), rolling_mean(), rolling_median(), rolling_std(), rolling_var(), rolling_skew(), rolling_kurt(), rolling_quantile(), rolling_cov(), rolling_corr(), rolling_corr_pairwise(), rolling_window(), and rolling_apply() with center=True previously would return a result of the same structure as the input arg with NaN in the final (window-1)/2 entries.
Now the final (window-1)/2 entries of the result are calculated as if the input arg were followed by (window-1)/2 NaN values (or with shrinking windows, in the case of rolling_apply()). (GH7925, GH8269)
Prior behavior (note final value is NaN):
In [7]: rolling_sum(Series(range(4)), window=3, min_periods=0, center=True) Out[7]: 0 1 1 3 2 6 3 NaN dtype: float64
New behavior (note final value is 5 = sum([2, 3, NaN])):
In [66]: rolling_sum(Series(range(4)), window=3, min_periods=0, center=True) Out[66]: 0 1 1 3 2 6 3 5 dtype: float64
rolling_window() now normalizes the weights properly in rolling mean mode (mean=True) so that the calculated weighted means (e.g. ‘triang’, ‘gaussian’) are distributed about the same means as those calculated without weighting (i.e. ‘boxcar’). See the note on normalization for further details. (GH7618)
In [67]: s = Series([10.5, 8.8, 11.4, 9.7, 9.3])
Behavior prior to 0.15.0:
In [39]: rolling_window(s, window=3, win_type='triang', center=True) Out[39]: 0 NaN 1 6.583333 2 6.883333 3 6.683333 4 NaN dtype: float64
New behavior
In [68]: rolling_window(s, window=3, win_type='triang', center=True) Out[68]: 0 NaN 1 9.875 2 10.325 3 10.025 4 NaN dtype: float64
Removed center argument from all expanding_ functions (see list), as the results produced when center=True did not make much sense. (GH7925)
Added optional ddof argument to expanding_cov() and rolling_cov(). The default value of 1 is backwards-compatible. (GH8279)
Documented the ddof argument to expanding_var(), expanding_std(), rolling_var(), and rolling_std(). These functions’ support of a ddof argument (with a default value of 1) was previously undocumented. (GH8064)
ewma(), ewmstd(), ewmvol(), ewmvar(), ewmcov(), and ewmcorr() now interpret min_periods in the same manner that the rolling_*() and expanding_*() functions do: a given result entry will be NaN if the (expanding, in this case) window does not contain at least min_periods values. The previous behavior was to set to NaN the min_periods entries starting with the first non- NaN value. (GH7977)
Prior behavior (note values start at index 2, which is min_periods after index 0 (the index of the first non-empty value)):
In [69]: s = Series([1, None, None, None, 2, 3])
In [51]: ewma(s, com=3., min_periods=2) Out[51]: 0 NaN 1 NaN 2 1.000000 3 1.000000 4 1.571429 5 2.189189 dtype: float64
New behavior (note values start at index 4, the location of the 2nd (since min_periods=2) non-empty value):
In [70]: ewma(s, com=3., min_periods=2) Out[70]: 0 NaN 1 NaN 2 NaN 3 NaN 4 1.759644 5 2.383784 dtype: float64
ewmstd(), ewmvol(), ewmvar(), ewmcov(), and ewmcorr() now have an optional adjust argument, just like ewma() does, affecting how the weights are calculated. The default value of adjust is True, which is backwards-compatible. See Exponentially weighted moment functions for details. (GH7911)
ewma(), ewmstd(), ewmvol(), ewmvar(), ewmcov(), and ewmcorr() now have an optional ignore_na argument. When ignore_na=False (the default), missing values are taken into account in the weights calculation. When ignore_na=True (which reproduces the pre-0.15.0 behavior), missing values are ignored in the weights calculation. (GH7543)
In [71]: ewma(Series([None, 1., 8.]), com=2.) Out[71]: 0 NaN 1 1.0 2 5.2 dtype: float64 In [72]: ewma(Series([1., None, 8.]), com=2., ignore_na=True) # pre-0.15.0 behavior Out[72]: 0 1.0 1 1.0 2 5.2 dtype: float64 In [73]: ewma(Series([1., None, 8.]), com=2., ignore_na=False) # new default Out[73]: 0 1.000000 1 1.000000 2 5.846154 dtype: float64
Warning
By default (ignore_na=False) the ewm*() functions’ weights calculation in the presence of missing values is different than in pre-0.15.0 versions. To reproduce the pre-0.15.0 calculation of weights in the presence of missing values one must specify explicitly ignore_na=True.
Bug in expanding_cov(), expanding_corr(), rolling_cov(), rolling_cor(), ewmcov(), and ewmcorr() returning results with columns sorted by name and producing an error for non-unique columns; now handles non-unique columns and returns columns in original order (except for the case of two DataFrames with pairwise=False, where behavior is unchanged) (GH7542)
Bug in rolling_count() and expanding_*() functions unnecessarily producing error message for zero-length data (GH8056)
Bug in rolling_apply() and expanding_apply() interpreting min_periods=0 as min_periods=1 (GH8080)
Bug in expanding_std() and expanding_var() for a single value producing a confusing error message (GH7900)
Bug in rolling_std() and rolling_var() for a single value producing 0 rather than NaN (GH7900)
Bug in ewmstd(), ewmvol(), ewmvar(), and ewmcov() calculation of de-biasing factors when bias=False (the default). Previously an incorrect constant factor was used, based on adjust=True, ignore_na=True, and an infinite number of observations. Now a different factor is used for each entry, based on the actual weights (analogous to the usual N/(N-1) factor). In particular, for a single point a value of NaN is returned when bias=False, whereas previously a value of (approximately) 0 was returned.
For example, consider the following pre-0.15.0 results for ewmvar(..., bias=False), and the corresponding debiasing factors:
In [74]: s = Series([1., 2., 0., 4.])
In [89]: ewmvar(s, com=2., bias=False) Out[89]: 0 -2.775558e-16 1 3.000000e-01 2 9.556787e-01 3 3.585799e+00 dtype: float64 In [90]: ewmvar(s, com=2., bias=False) / ewmvar(s, com=2., bias=True) Out[90]: 0 1.25 1 1.25 2 1.25 3 1.25 dtype: float64
Note that entry 0 is approximately 0, and the debiasing factors are a constant 1.25. By comparison, the following 0.15.0 results have a NaN for entry 0, and the debiasing factors are decreasing (towards 1.25):
In [75]: ewmvar(s, com=2., bias=False) Out[75]: 0 NaN 1 0.500000 2 1.210526 3 4.089069 dtype: float64 In [76]: ewmvar(s, com=2., bias=False) / ewmvar(s, com=2., bias=True) Out[76]: 0 NaN 1 2.083333 2 1.583333 3 1.425439 dtype: float64
See Exponentially weighted moment functions for details. (GH7912)
Improvements in the sql io module¶
Added support for a chunksize parameter to to_sql function. This allows DataFrame to be written in chunks and avoid packet-size overflow errors (GH8062).
Added support for a chunksize parameter to read_sql function. Specifying this argument will return an iterator through chunks of the query result (GH2908).
Added support for writing datetime.date and datetime.time object columns with to_sql (GH6932).
Added support for specifying a schema to read from/write to with read_sql_table and to_sql (GH7441, GH7952). For example:
df.to_sql('table', engine, schema='other_schema') pd.read_sql_table('table', engine, schema='other_schema')
Added support for writing NaN values with to_sql (GH2754).
Added support for writing datetime64 columns with to_sql for all database flavors (GH7103).
Backwards incompatible API changes¶
Breaking changes¶
API changes related to Categorical (see here for more details):
The Categorical constructor with two arguments changed from “codes/labels and levels” to “values and levels (now called ‘categories’)”. This can lead to subtle bugs. If you use Categorical directly, please audit your code by changing it to use the from_codes() constructor.
An old function call like (prior to 0.15.0):
pd.Categorical([0,1,0,2,1], levels=['a', 'b', 'c'])
will have to adapted to the following to keep the same behaviour:
In [2]: pd.Categorical.from_codes([0,1,0,2,1], categories=['a', 'b', 'c']) Out[2]: [a, b, a, c, b] Categories (3, object): [a, b, c]
API changes related to the introduction of the Timedelta scalar (see above for more details):
- Prior to 0.15.0 to_timedelta() would return a Series for list-like/Series input, and a np.timedelta64 for scalar input. It will now return a TimedeltaIndex for list-like input, Series for Series input, and Timedelta for scalar input.
For API changes related to the rolling and expanding functions, see detailed overview above.
Other notable API changes:
Consistency when indexing with .loc and a list-like indexer when no values are found.
In [77]: df = DataFrame([['a'],['b']],index=[1,2]) In [78]: df Out[78]: 0 1 a 2 b
In prior versions there was a difference in these two constructs:
- df.loc[[3]] would return a frame reindexed by 3 (with all np.nan values)
- df.loc[[3],:] would raise KeyError.
Both will now raise a KeyError. The rule is that at least 1 indexer must be found when using a list-like and .loc (GH7999)
Furthermore in prior versions these were also different:
- df.loc[[1,3]] would return a frame reindexed by [1,3]
- df.loc[[1,3],:] would raise KeyError.
Both will now return a frame reindex by [1,3]. E.g.
In [79]: df.loc[[1,3]] Out[79]: 0 1 a 3 NaN In [80]: df.loc[[1,3],:] Out[80]: 0 1 a 3 NaN
This can also be seen in multi-axis indexing with a Panel.
In [81]: p = Panel(np.arange(2*3*4).reshape(2,3,4), ....: items=['ItemA','ItemB'], ....: major_axis=[1,2,3], ....: minor_axis=['A','B','C','D']) ....: In [82]: p Out[82]: <class 'pandas.core.panel.Panel'> Dimensions: 2 (items) x 3 (major_axis) x 4 (minor_axis) Items axis: ItemA to ItemB Major_axis axis: 1 to 3 Minor_axis axis: A to D
The following would raise KeyError prior to 0.15.0:
In [83]: p.loc[['ItemA','ItemD'],:,'D'] Out[83]: ItemA ItemD 1 3 NaN 2 7 NaN 3 11 NaN
Furthermore, .loc will raise If no values are found in a multi-index with a list-like indexer:
In [84]: s = Series(np.arange(3,dtype='int64'), ....: index=MultiIndex.from_product([['A'],['foo','bar','baz']], ....: names=['one','two']) ....: ).sortlevel() ....: In [85]: s Out[85]: one two A bar 1 baz 2 foo 0 dtype: int64 In [86]: try: ....: s.loc[['D']] ....: except KeyError as e: ....: print("KeyError: " + str(e)) ....: KeyError: 'cannot index a multi-index axis with these keys'
Assigning values to None now considers the dtype when choosing an ‘empty’ value (GH7941).
Previously, assigning to None in numeric containers changed the dtype to object (or errored, depending on the call). It now uses NaN:
In [87]: s = Series([1, 2, 3]) In [88]: s.loc[0] = None In [89]: s Out[89]: 0 NaN 1 2 2 3 dtype: float64
NaT is now used similarly for datetime containers.
For object containers, we now preserve None values (previously these were converted to NaN values).
In [90]: s = Series(["a", "b", "c"]) In [91]: s.loc[0] = None In [92]: s Out[92]: 0 None 1 b 2 c dtype: object
To insert a NaN, you must explicitly use np.nan. See the docs.
In prior versions, updating a pandas object inplace would not reflect in other python references to this object. (GH8511, GH5104)
In [93]: s = Series([1, 2, 3]) In [94]: s2 = s In [95]: s += 1.5
Behavior prior to v0.15.0
# the original object In [5]: s Out[5]: 0 2.5 1 3.5 2 4.5 dtype: float64 # a reference to the original object In [7]: s2 Out[7]: 0 1 1 2 2 3 dtype: int64
This is now the correct behavior
# the original object In [96]: s Out[96]: 0 2.5 1 3.5 2 4.5 dtype: float64 # a reference to the original object In [97]: s2 Out[97]: 0 2.5 1 3.5 2 4.5 dtype: float64
Made both the C-based and Python engines for read_csv and read_table ignore empty lines in input as well as whitespace-filled lines, as long as sep is not whitespace. This is an API change that can be controlled by the keyword parameter skip_blank_lines. See the docs (GH4466)
A timeseries/index localized to UTC when inserted into a Series/DataFrame will preserve the UTC timezone and inserted as object dtype rather than being converted to a naive datetime64[ns] (GH8411).
Bug in passing a DatetimeIndex with a timezone that was not being retained in DataFrame construction from a dict (GH7822)
In prior versions this would drop the timezone, now it retains the timezone, but gives a column of object dtype:
In [98]: i = date_range('1/1/2011', periods=3, freq='10s', tz = 'US/Eastern') In [99]: i Out[99]: DatetimeIndex(['2011-01-01 00:00:00-05:00', '2011-01-01 00:00:10-05:00', '2011-01-01 00:00:20-05:00'], dtype='datetime64[ns, US/Eastern]', freq='10S') In [100]: df = DataFrame( {'a' : i } ) In [101]: df Out[101]: a 0 2011-01-01 00:00:00-05:00 1 2011-01-01 00:00:10-05:00 2 2011-01-01 00:00:20-05:00 In [102]: df.dtypes Out[102]: a datetime64[ns, US/Eastern] dtype: object
Previously this would have yielded a column of datetime64 dtype, but without timezone info.
The behaviour of assigning a column to an existing dataframe as df[‘a’] = i remains unchanged (this already returned an object column with a timezone).
When passing multiple levels to stack(), it will now raise a ValueError when the levels aren’t all level names or all level numbers (GH7660). See Reshaping by stacking and unstacking.
Raise a ValueError in df.to_hdf with ‘fixed’ format, if df has non-unique columns as the resulting file will be broken (GH7761)
SettingWithCopy raise/warnings (according to the option mode.chained_assignment) will now be issued when setting a value on a sliced mixed-dtype DataFrame using chained-assignment. (GH7845, GH7950)
In [1]: df = DataFrame(np.arange(0,9), columns=['count']) In [2]: df['group'] = 'b' In [3]: df.iloc[0:5]['group'] = 'a' /usr/local/bin/ipython:1: SettingWithCopyWarning: A value is trying to be set on a copy of a slice from a DataFrame. Try using .loc[row_indexer,col_indexer] = value instead See the the caveats in the documentation: http://pandas.pydata.org/pandas-docs/stable/indexing.html#indexing-view-versus-copy
merge, DataFrame.merge, and ordered_merge now return the same type as the left argument (GH7737).
Previously an enlargement with a mixed-dtype frame would act unlike .append which will preserve dtypes (related GH2578, GH8176):
In [103]: df = DataFrame([[True, 1],[False, 2]], .....: columns=["female","fitness"]) .....: In [104]: df Out[104]: female fitness 0 True 1 1 False 2 In [105]: df.dtypes Out[105]: female bool fitness int64 dtype: object # dtypes are now preserved In [106]: df.loc[2] = df.loc[1] In [107]: df Out[107]: female fitness 0 True 1 1 False 2 2 False 2 In [108]: df.dtypes Out[108]: female bool fitness int64 dtype: object
Series.to_csv() now returns a string when path=None, matching the behaviour of DataFrame.to_csv() (GH8215).
read_hdf now raises IOError when a file that doesn’t exist is passed in. Previously, a new, empty file was created, and a KeyError raised (GH7715).
DataFrame.info() now ends its output with a newline character (GH8114)
Concatenating no objects will now raise a ValueError rather than a bare Exception.
Merge errors will now be sub-classes of ValueError rather than raw Exception (GH8501)
DataFrame.plot and Series.plot keywords are now have consistent orders (GH8037)
Internal Refactoring¶
In 0.15.0 Index has internally been refactored to no longer sub-class ndarray but instead subclass PandasObject, similarly to the rest of the pandas objects. This change allows very easy sub-classing and creation of new index types. This should be a transparent change with only very limited API implications (GH5080, GH7439, GH7796, GH8024, GH8367, GH7997, GH8522):
- you may need to unpickle pandas version < 0.15.0 pickles using pd.read_pickle rather than pickle.load. See pickle docs
- when plotting with a PeriodIndex, the matplotlib internal axes will now be arrays of Period rather than a PeriodIndex (this is similar to how a DatetimeIndex passes arrays of datetimes now)
- MultiIndexes will now raise similary to other pandas objects w.r.t. truth testing, see here (GH7897).
- When plotting a DatetimeIndex directly with matplotlib’s plot function, the axis labels will no longer be formatted as dates but as integers (the internal representation of a datetime64). UPDATE This is fixed in 0.15.1, see here.
Deprecations¶
- The attributes Categorical labels and levels attributes are deprecated and renamed to codes and categories.
- The outtype argument to pd.DataFrame.to_dict has been deprecated in favor of orient. (GH7840)
- The convert_dummies method has been deprecated in favor of get_dummies (GH8140)
- The infer_dst argument in tz_localize will be deprecated in favor of ambiguous to allow for more flexibility in dealing with DST transitions. Replace infer_dst=True with ambiguous='infer' for the same behavior (GH7943). See the docs for more details.
- The top-level pd.value_range has been deprecated and can be replaced by .describe() (GH8481)
The Index set operations + and - were deprecated in order to provide these for numeric type operations on certain index types. + can be replaced by .union() or |, and - by .difference(). Further the method name Index.diff() is deprecated and can be replaced by Index.difference() (GH8226)
# + Index(['a','b','c']) + Index(['b','c','d']) # should be replaced by Index(['a','b','c']).union(Index(['b','c','d']))
# - Index(['a','b','c']) - Index(['b','c','d']) # should be replaced by Index(['a','b','c']).difference(Index(['b','c','d']))
The infer_types argument to read_html() now has no effect and is deprecated (GH7762, GH7032).
Removal of prior version deprecations/changes¶
- Remove DataFrame.delevel method in favor of DataFrame.reset_index
Enhancements¶
Enhancements in the importing/exporting of Stata files:
- Added support for bool, uint8, uint16 and uint32 datatypes in to_stata (GH7097, GH7365)
- Added conversion option when importing Stata files (GH8527)
- DataFrame.to_stata and StataWriter check string length for compatibility with limitations imposed in dta files where fixed-width strings must contain 244 or fewer characters. Attempting to write Stata dta files with strings longer than 244 characters raises a ValueError. (GH7858)
- read_stata and StataReader can import missing data information into a DataFrame by setting the argument convert_missing to True. When using this options, missing values are returned as StataMissingValue objects and columns containing missing values have object data type. (GH8045)
Enhancements in the plotting functions:
- Added layout keyword to DataFrame.plot. You can pass a tuple of (rows, columns), one of which can be -1 to automatically infer (GH6667, GH8071).
- Allow to pass multiple axes to DataFrame.plot, hist and boxplot (GH5353, GH6970, GH7069)
- Added support for c, colormap and colorbar arguments for DataFrame.plot with kind='scatter' (GH7780)
- Histogram from DataFrame.plot with kind='hist' (GH7809), See the docs.
- Boxplot from DataFrame.plot with kind='box' (GH7998), See the docs.
Other:
read_csv now has a keyword parameter float_precision which specifies which floating-point converter the C engine should use during parsing, see here (GH8002, GH8044)
Added searchsorted method to Series objects (GH7447)
describe() on mixed-types DataFrames is more flexible. Type-based column filtering is now possible via the include/exclude arguments. See the docs (GH8164).
In [109]: df = DataFrame({'catA': ['foo', 'foo', 'bar'] * 8, .....: 'catB': ['a', 'b', 'c', 'd'] * 6, .....: 'numC': np.arange(24), .....: 'numD': np.arange(24.) + .5}) .....: In [110]: df.describe(include=["object"]) Out[110]: catA catB count 24 24 unique 2 4 top foo d freq 16 6 In [111]: df.describe(include=["number", "object"], exclude=["float"]) Out[111]: catA catB numC count 24 24 24.000000 unique 2 4 NaN top foo d NaN freq 16 6 NaN mean NaN NaN 11.500000 std NaN NaN 7.071068 min NaN NaN 0.000000 25% NaN NaN 5.750000 50% NaN NaN 11.500000 75% NaN NaN 17.250000 max NaN NaN 23.000000
Requesting all columns is possible with the shorthand ‘all’
In [112]: df.describe(include='all') Out[112]: catA catB numC numD count 24 24 24.000000 24.000000 unique 2 4 NaN NaN top foo d NaN NaN freq 16 6 NaN NaN mean NaN NaN 11.500000 12.000000 std NaN NaN 7.071068 7.071068 min NaN NaN 0.000000 0.500000 25% NaN NaN 5.750000 6.250000 50% NaN NaN 11.500000 12.000000 75% NaN NaN 17.250000 17.750000 max NaN NaN 23.000000 23.500000
Without those arguments, ‘describe` will behave as before, including only numerical columns or, if none are, only categorical columns. See also the docs
Added split as an option to the orient argument in pd.DataFrame.to_dict. (GH7840)
The get_dummies method can now be used on DataFrames. By default only catagorical columns are encoded as 0’s and 1’s, while other columns are left untouched.
In [113]: df = DataFrame({'A': ['a', 'b', 'a'], 'B': ['c', 'c', 'b'], .....: 'C': [1, 2, 3]}) .....: In [114]: pd.get_dummies(df) Out[114]: C A_a A_b B_b B_c 0 1 1 0 0 1 1 2 0 1 0 1 2 3 1 0 1 0
PeriodIndex supports resolution as the same as DatetimeIndex (GH7708)
pandas.tseries.holiday has added support for additional holidays and ways to observe holidays (GH7070)
pandas.tseries.holiday.Holiday now supports a list of offsets in Python3 (GH7070)
pandas.tseries.holiday.Holiday now supports a days_of_week parameter (GH7070)
GroupBy.nth() now supports selecting multiple nth values (GH7910)
In [115]: business_dates = date_range(start='4/1/2014', end='6/30/2014', freq='B') In [116]: df = DataFrame(1, index=business_dates, columns=['a', 'b']) # get the first, 4th, and last date index for each month In [117]: df.groupby((df.index.year, df.index.month)).nth([0, 3, -1]) Out[117]: a b 2014-04-01 1 1 2014-04-04 1 1 2014-04-30 1 1 2014-05-01 1 1 2014-05-06 1 1 2014-05-30 1 1 2014-06-02 1 1 2014-06-05 1 1 2014-06-30 1 1
Period and PeriodIndex supports addition/subtraction with timedelta-likes (GH7966)
If Period freq is D, H, T, S, L, U, N, Timedelta-like can be added if the result can have same freq. Otherwise, only the same offsets can be added.
In [118]: idx = pd.period_range('2014-07-01 09:00', periods=5, freq='H') In [119]: idx Out[119]: PeriodIndex(['2014-07-01 09:00', '2014-07-01 10:00', '2014-07-01 11:00', '2014-07-01 12:00', '2014-07-01 13:00'], dtype='int64', freq='H') In [120]: idx + pd.offsets.Hour(2) Out[120]: PeriodIndex(['2014-07-01 11:00', '2014-07-01 12:00', '2014-07-01 13:00', '2014-07-01 14:00', '2014-07-01 15:00'], dtype='int64', freq='H') In [121]: idx + Timedelta('120m') Out[121]: PeriodIndex(['2014-07-01 11:00', '2014-07-01 12:00', '2014-07-01 13:00', '2014-07-01 14:00', '2014-07-01 15:00'], dtype='int64', freq='H') In [122]: idx = pd.period_range('2014-07', periods=5, freq='M') In [123]: idx Out[123]: PeriodIndex(['2014-07', '2014-08', '2014-09', '2014-10', '2014-11'], dtype='int64', freq='M') In [124]: idx + pd.offsets.MonthEnd(3) Out[124]: PeriodIndex(['2014-10', '2014-11', '2014-12', '2015-01', '2015-02'], dtype='int64', freq='M')
Added experimental compatibility with openpyxl for versions >= 2.0. The DataFrame.to_excel method engine keyword now recognizes openpyxl1 and openpyxl2 which will explicitly require openpyxl v1 and v2 respectively, failing if the requested version is not available. The openpyxl engine is a now a meta-engine that automatically uses whichever version of openpyxl is installed. (GH7177)
DataFrame.fillna can now accept a DataFrame as a fill value (GH8377)
Passing multiple levels to stack() will now work when multiple level numbers are passed (GH7660). See Reshaping by stacking and unstacking.
set_names(), set_labels(), and set_levels() methods now take an optional level keyword argument to all modification of specific level(s) of a MultiIndex. Additionally set_names() now accepts a scalar string value when operating on an Index or on a specific level of a MultiIndex (GH7792)
In [125]: idx = MultiIndex.from_product([['a'], range(3), list("pqr")], names=['foo', 'bar', 'baz']) In [126]: idx.set_names('qux', level=0) Out[126]: MultiIndex(levels=[[u'a'], [0, 1, 2], [u'p', u'q', u'r']], labels=[[0, 0, 0, 0, 0, 0, 0, 0, 0], [0, 0, 0, 1, 1, 1, 2, 2, 2], [0, 1, 2, 0, 1, 2, 0, 1, 2]], names=[u'qux', u'bar', u'baz']) In [127]: idx.set_names(['qux','baz'], level=[0,1]) Out[127]: MultiIndex(levels=[[u'a'], [0, 1, 2], [u'p', u'q', u'r']], labels=[[0, 0, 0, 0, 0, 0, 0, 0, 0], [0, 0, 0, 1, 1, 1, 2, 2, 2], [0, 1, 2, 0, 1, 2, 0, 1, 2]], names=[u'qux', u'baz', u'baz']) In [128]: idx.set_levels(['a','b','c'], level='bar') Out[128]: MultiIndex(levels=[[u'a'], [u'a', u'b', u'c'], [u'p', u'q', u'r']], labels=[[0, 0, 0, 0, 0, 0, 0, 0, 0], [0, 0, 0, 1, 1, 1, 2, 2, 2], [0, 1, 2, 0, 1, 2, 0, 1, 2]], names=[u'foo', u'bar', u'baz']) In [129]: idx.set_levels([['a','b','c'],[1,2,3]], level=[1,2]) Out[129]: MultiIndex(levels=[[u'a'], [u'a', u'b', u'c'], [1, 2, 3]], labels=[[0, 0, 0, 0, 0, 0, 0, 0, 0], [0, 0, 0, 1, 1, 1, 2, 2, 2], [0, 1, 2, 0, 1, 2, 0, 1, 2]], names=[u'foo', u'bar', u'baz'])
Index.isin now supports a level argument to specify which index level to use for membership tests (GH7892, GH7890)
In [1]: idx = MultiIndex.from_product([[0, 1], ['a', 'b', 'c']]) In [2]: idx.values Out[2]: array([(0, 'a'), (0, 'b'), (0, 'c'), (1, 'a'), (1, 'b'), (1, 'c')], dtype=object) In [3]: idx.isin(['a', 'c', 'e'], level=1) Out[3]: array([ True, False, True, True, False, True], dtype=bool)
Index now supports duplicated and drop_duplicates. (GH4060)
In [130]: idx = Index([1, 2, 3, 4, 1, 2]) In [131]: idx Out[131]: Int64Index([1, 2, 3, 4, 1, 2], dtype='int64') In [132]: idx.duplicated() Out[132]: array([False, False, False, False, True, True], dtype=bool) In [133]: idx.drop_duplicates() Out[133]: Int64Index([1, 2, 3, 4], dtype='int64')
add copy=True argument to pd.concat to enable pass thru of complete blocks (GH8252)
Added support for numpy 1.8+ data types (bool_, int_, float_, string_) for conversion to R dataframe (GH8400)
Performance¶
- Performance improvements in DatetimeIndex.__iter__ to allow faster iteration (GH7683)
- Performance improvements in Period creation (and PeriodIndex setitem) (GH5155)
- Improvements in Series.transform for significant performance gains (revised) (GH6496)
- Performance improvements in StataReader when reading large files (GH8040, GH8073)
- Performance improvements in StataWriter when writing large files (GH8079)
- Performance and memory usage improvements in multi-key groupby (GH8128)
- Performance improvements in groupby .agg and .apply where builtins max/min were not mapped to numpy/cythonized versions (GH7722)
- Performance improvement in writing to sql (to_sql) of up to 50% (GH8208).
- Performance benchmarking of groupby for large value of ngroups (GH6787)
- Performance improvement in CustomBusinessDay, CustomBusinessMonth (GH8236)
- Performance improvement for MultiIndex.values for multi-level indexes containing datetimes (GH8543)
Bug Fixes¶
- Bug in pivot_table, when using margins and a dict aggfunc (GH8349)
- Bug in read_csv where squeeze=True would return a view (GH8217)
- Bug in checking of table name in read_sql in certain cases (GH7826).
- Bug in DataFrame.groupby where Grouper does not recognize level when frequency is specified (GH7885)
- Bug in multiindexes dtypes getting mixed up when DataFrame is saved to SQL table (GH8021)
- Bug in Series 0-division with a float and integer operand dtypes (GH7785)
- Bug in Series.astype("unicode") not calling unicode on the values correctly (GH7758)
- Bug in DataFrame.as_matrix() with mixed datetime64[ns] and timedelta64[ns] dtypes (GH7778)
- Bug in HDFStore.select_column() not preserving UTC timezone info when selecting a DatetimeIndex (GH7777)
- Bug in to_datetime when format='%Y%m%d' and coerce=True are specified, where previously an object array was returned (rather than a coerced time-series with NaT), (GH7930)
- Bug in DatetimeIndex and PeriodIndex in-place addition and subtraction cause different result from normal one (GH6527)
- Bug in adding and subtracting PeriodIndex with PeriodIndex raise TypeError (GH7741)
- Bug in combine_first with PeriodIndex data raises TypeError (GH3367)
- Bug in multi-index slicing with missing indexers (GH7866)
- Bug in multi-index slicing with various edge cases (GH8132)
- Regression in multi-index indexing with a non-scalar type object (GH7914)
- Bug in Timestamp comparisons with == and int64 dtype (GH8058)
- Bug in pickles contains DateOffset may raise AttributeError when normalize attribute is reffered internally (GH7748)
- Bug in Panel when using major_xs and copy=False is passed (deprecation warning fails because of missing warnings) (GH8152).
- Bug in pickle deserialization that failed for pre-0.14.1 containers with dup items trying to avoid ambiguity when matching block and manager items, when there’s only one block there’s no ambiguity (GH7794)
- Bug in putting a PeriodIndex into a Series would convert to int64 dtype, rather than object of Periods (GH7932)
- Bug in HDFStore iteration when passing a where (GH8014)
- Bug in DataFrameGroupby.transform when transforming with a passed non-sorted key (GH8046, GH8430)
- Bug in repeated timeseries line and area plot may result in ValueError or incorrect kind (GH7733)
- Bug in inference in a MultiIndex with datetime.date inputs (GH7888)
- Bug in get where an IndexError would not cause the default value to be returned (GH7725)
- Bug in offsets.apply, rollforward and rollback may reset nanosecond (GH7697)
- Bug in offsets.apply, rollforward and rollback may raise AttributeError if Timestamp has dateutil tzinfo (GH7697)
- Bug in sorting a multi-index frame with a Float64Index (GH8017)
- Bug in inconsistent panel setitem with a rhs of a DataFrame for alignment (GH7763)
- Bug in is_superperiod and is_subperiod cannot handle higher frequencies than S (GH7760, GH7772, GH7803)
- Bug in 32-bit platforms with Series.shift (GH8129)
- Bug in PeriodIndex.unique returns int64 np.ndarray (GH7540)
- Bug in groupby.apply with a non-affecting mutation in the function (GH8467)
- Bug in DataFrame.reset_index which has MultiIndex contains PeriodIndex or DatetimeIndex with tz raises ValueError (GH7746, GH7793)
- Bug in DataFrame.plot with subplots=True may draw unnecessary minor xticks and yticks (GH7801)
- Bug in StataReader which did not read variable labels in 117 files due to difference between Stata documentation and implementation (GH7816)
- Bug in StataReader where strings were always converted to 244 characters-fixed width irrespective of underlying string size (GH7858)
- Bug in DataFrame.plot and Series.plot may ignore rot and fontsize keywords (GH7844)
- Bug in DatetimeIndex.value_counts doesn’t preserve tz (GH7735)
- Bug in PeriodIndex.value_counts results in Int64Index (GH7735)
- Bug in DataFrame.join when doing left join on index and there are multiple matches (GH5391)
- Bug in GroupBy.transform() where int groups with a transform that didn’t preserve the index were incorrectly truncated (GH7972).
- Bug in groupby where callable objects without name attributes would take the wrong path, and produce a DataFrame instead of a Series (GH7929)
- Bug in groupby error message when a DataFrame grouping column is duplicated (GH7511)
- Bug in read_html where the infer_types argument forced coercion of date-likes incorrectly (GH7762, GH7032).
- Bug in Series.str.cat with an index which was filtered as to not include the first item (GH7857)
- Bug in Timestamp cannot parse nanosecond from string (GH7878)
- Bug in Timestamp with string offset and tz results incorrect (GH7833)
- Bug in tslib.tz_convert and tslib.tz_convert_single may return different results (GH7798)
- Bug in DatetimeIndex.intersection of non-overlapping timestamps with tz raises IndexError (GH7880)
- Bug in alignment with TimeOps and non-unique indexes (GH8363)
- Bug in GroupBy.filter() where fast path vs. slow path made the filter return a non scalar value that appeared valid but wasn’t (GH7870).
- Bug in date_range()/DatetimeIndex() when the timezone was inferred from input dates yet incorrect times were returned when crossing DST boundaries (GH7835, GH7901).
- Bug in to_excel() where a negative sign was being prepended to positive infinity and was absent for negative infinity (GH7949)
- Bug in area plot draws legend with incorrect alpha when stacked=True (GH8027)
- Period and PeriodIndex addition/subtraction with np.timedelta64 results in incorrect internal representations (GH7740)
- Bug in Holiday with no offset or observance (GH7987)
- Bug in DataFrame.to_latex formatting when columns or index is a MultiIndex (GH7982).
- Bug in DateOffset around Daylight Savings Time produces unexpected results (GH5175).
- Bug in DataFrame.shift where empty columns would throw ZeroDivisionError on numpy 1.7 (GH8019)
- Bug in installation where html_encoding/*.html wasn’t installed and therefore some tests were not running correctly (GH7927).
- Bug in read_html where bytes objects were not tested for in _read (GH7927).
- Bug in DataFrame.stack() when one of the column levels was a datelike (GH8039)
- Bug in broadcasting numpy scalars with DataFrame (GH8116)
- Bug in pivot_table performed with nameless index and columns raises KeyError (GH8103)
- Bug in DataFrame.plot(kind='scatter') draws points and errorbars with different colors when the color is specified by c keyword (GH8081)
- Bug in Float64Index where iat and at were not testing and were failing (GH8092).
- Bug in DataFrame.boxplot() where y-limits were not set correctly when producing multiple axes (GH7528, GH5517).
- Bug in read_csv where line comments were not handled correctly given a custom line terminator or delim_whitespace=True (GH8122).
- Bug in read_html where empty tables caused a StopIteration (GH7575)
- Bug in casting when setting a column in a same-dtype block (GH7704)
- Bug in accessing groups from a GroupBy when the original grouper was a tuple (GH8121).
- Bug in .at that would accept integer indexers on a non-integer index and do fallback (GH7814)
- Bug with kde plot and NaNs (GH8182)
- Bug in GroupBy.count with float32 data type were nan values were not excluded (GH8169).
- Bug with stacked barplots and NaNs (GH8175).
- Bug in resample with non evenly divisible offsets (e.g. ‘7s’) (GH8371)
- Bug in interpolation methods with the limit keyword when no values needed interpolating (GH7173).
- Bug where col_space was ignored in DataFrame.to_string() when header=False (GH8230).
- Bug with DatetimeIndex.asof incorrectly matching partial strings and returning the wrong date (GH8245).
- Bug in plotting methods modifying the global matplotlib rcParams (GH8242).
- Bug in DataFrame.__setitem__ that caused errors when setting a dataframe column to a sparse array (GH8131)
- Bug where Dataframe.boxplot() failed when entire column was empty (GH8181).
- Bug with messed variables in radviz visualization (GH8199).
- Bug in interpolation methods with the limit keyword when no values needed interpolating (GH7173).
- Bug where col_space was ignored in DataFrame.to_string() when header=False (GH8230).
- Bug in to_clipboard that would clip long column data (GH8305)
- Bug in DataFrame terminal display: Setting max_column/max_rows to zero did not trigger auto-resizing of dfs to fit terminal width/height (GH7180).
- Bug in OLS where running with “cluster” and “nw_lags” parameters did not work correctly, but also did not throw an error (GH5884).
- Bug in DataFrame.dropna that interpreted non-existent columns in the subset argument as the ‘last column’ (GH8303)
- Bug in Index.intersection on non-monotonic non-unique indexes (GH8362).
- Bug in masked series assignment where mismatching types would break alignment (GH8387)
- Bug in NDFrame.equals gives false negatives with dtype=object (GH8437)
- Bug in assignment with indexer where type diversity would break alignment (GH8258)
- Bug in NDFrame.loc indexing when row/column names were lost when target was a list/ndarray (GH6552)
- Regression in NDFrame.loc indexing when rows/columns were converted to Float64Index if target was an empty list/ndarray (GH7774)
- Bug in Series that allows it to be indexed by a DataFrame which has unexpected results. Such indexing is no longer permitted (GH8444)
- Bug in item assignment of a DataFrame with multi-index columns where right-hand-side columns were not aligned (GH7655)
- Suppress FutureWarning generated by NumPy when comparing object arrays containing NaN for equality (GH7065)
- Bug in DataFrame.eval() where the dtype of the not operator (~) was not correctly inferred as bool.
v0.14.1 (July 11, 2014)¶
This is a minor release from 0.14.0 and includes a small number of API changes, several new features, enhancements, and performance improvements along with a large number of bug fixes. We recommend that all users upgrade to this version.
- Highlights include:
- New methods select_dtypes() to select columns based on the dtype and sem() to calculate the standard error of the mean.
- Support for dateutil timezones (see docs).
- Support for ignoring full line comments in the read_csv() text parser.
- New documentation section on Options and Settings.
- Lots of bug fixes.
- Enhancements
- API Changes
- Performance Improvements
- Experimental Changes
- Bug Fixes
API changes¶
Openpyxl now raises a ValueError on construction of the openpyxl writer instead of warning on pandas import (GH7284).
For StringMethods.extract, when no match is found, the result - only containing NaN values - now also has dtype=object instead of float (GH7242)
Period objects no longer raise a TypeError when compared using == with another object that isn’t a Period. Instead when comparing a Period with another object using == if the other object isn’t a Period False is returned. (GH7376)
Previously, the behaviour on resetting the time or not in offsets.apply, rollforward and rollback operations differed between offsets. With the support of the normalize keyword for all offsets(see below) with a default value of False (preserve time), the behaviour changed for certain offsets (BusinessMonthBegin, MonthEnd, BusinessMonthEnd, CustomBusinessMonthEnd, BusinessYearBegin, LastWeekOfMonth, FY5253Quarter, LastWeekOfMonth, Easter):
In [6]: from pandas.tseries import offsets In [7]: d = pd.Timestamp('2014-01-01 09:00') # old behaviour < 0.14.1 In [8]: d + offsets.MonthEnd() Out[8]: Timestamp('2014-01-31 00:00:00')
Starting from 0.14.1 all offsets preserve time by default. The old behaviour can be obtained with normalize=True
# new behaviour In [1]: d + offsets.MonthEnd() Out[1]: Timestamp('2014-01-31 09:00:00') In [2]: d + offsets.MonthEnd(normalize=True) Out[2]: Timestamp('2014-01-31 00:00:00')
Note that for the other offsets the default behaviour did not change.
Add back #N/A N/A as a default NA value in text parsing, (regresion from 0.12) (GH5521)
Raise a TypeError on inplace-setting with a .where and a non np.nan value as this is inconsistent with a set-item expression like df[mask] = None (GH7656)
Enhancements¶
Add dropna argument to value_counts and nunique (GH5569).
Add select_dtypes() method to allow selection of columns based on dtype (GH7316). See the docs.
All offsets suppports the normalize keyword to specify whether offsets.apply, rollforward and rollback resets the time (hour, minute, etc) or not (default False, preserves time) (GH7156):
In [3]: import pandas.tseries.offsets as offsets In [4]: day = offsets.Day() In [5]: day.apply(Timestamp('2014-01-01 09:00')) Out[5]: Timestamp('2014-01-02 09:00:00') In [6]: day = offsets.Day(normalize=True) In [7]: day.apply(Timestamp('2014-01-01 09:00')) Out[7]: Timestamp('2014-01-02 00:00:00')
PeriodIndex is represented as the same format as DatetimeIndex (GH7601)
StringMethods now work on empty Series (GH7242)
The file parsers read_csv and read_table now ignore line comments provided by the parameter comment, which accepts only a single character for the C reader. In particular, they allow for comments before file data begins (GH2685)
Add NotImplementedError for simultaneous use of chunksize and nrows for read_csv() (GH6774).
Tests for basic reading of public S3 buckets now exist (GH7281).
read_html now sports an encoding argument that is passed to the underlying parser library. You can use this to read non-ascii encoded web pages (GH7323).
read_excel now supports reading from URLs in the same way that read_csv does. (GH6809)
Support for dateutil timezones, which can now be used in the same way as pytz timezones across pandas. (GH4688)
In [8]: rng = date_range('3/6/2012 00:00', periods=10, freq='D', ...: tz='dateutil/Europe/London') ...: In [9]: rng.tz Out[9]: tzfile('/usr/share/zoneinfo/Europe/London')
See the docs.
Implemented sem (standard error of the mean) operation for Series, DataFrame, Panel, and Groupby (GH6897)
Add nlargest and nsmallest to the Series groupby whitelist, which means you can now use these methods on a SeriesGroupBy object (GH7053).
All offsets apply, rollforward and rollback can now handle np.datetime64, previously results in ApplyTypeError (GH7452)
Period and PeriodIndex can contain NaT in its values (GH7485)
Support pickling Series, DataFrame and Panel objects with non-unique labels along item axis (index, columns and items respectively) (GH7370).
Improved inference of datetime/timedelta with mixed null objects. Regression from 0.13.1 in interpretation of an object Index with all null elements (GH7431)
Performance¶
- Improvements in dtype inference for numeric operations involving yielding performance gains for dtypes: int64, timedelta64, datetime64 (GH7223)
- Improvements in Series.transform for significant performance gains (GH6496)
- Improvements in DataFrame.transform with ufuncs and built-in grouper functions for signifcant performance gains (GH7383)
- Regression in groupby aggregation of datetime64 dtypes (GH7555)
- Improvements in MultiIndex.from_product for large iterables (GH7627)
Experimental¶
- pandas.io.data.Options has a new method, get_all_data method, and now consistently returns a multi-indexed DataFrame, see the docs. (GH5602)
- io.gbq.read_gbq and io.gbq.to_gbq were refactored to remove the dependency on the Google bq.py command line client. This submodule now uses httplib2 and the Google apiclient and oauth2client API client libraries which should be more stable and, therefore, reliable than bq.py. See the docs. (GH6937).
Bug Fixes¶
- Bug in DataFrame.where with a symmetric shaped frame and a passed other of a DataFrame (GH7506)
- Bug in Panel indexing with a multi-index axis (GH7516)
- Regression in datetimelike slice indexing with a duplicated index and non-exact end-points (GH7523)
- Bug in setitem with list-of-lists and single vs mixed types (GH7551:)
- Bug in timeops with non-aligned Series (GH7500)
- Bug in timedelta inference when assigning an incomplete Series (GH7592)
- Bug in groupby .nth with a Series and integer-like column name (GH7559)
- Bug in Series.get with a boolean accessor (GH7407)
- Bug in value_counts where NaT did not qualify as missing (NaN) (GH7423)
- Bug in to_timedelta that accepted invalid units and misinterpreted ‘m/h’ (GH7611, GH6423)
- Bug in line plot doesn’t set correct xlim if secondary_y=True (GH7459)
- Bug in grouped hist and scatter plots use old figsize default (GH7394)
- Bug in plotting subplots with DataFrame.plot, hist clears passed ax even if the number of subplots is one (GH7391).
- Bug in plotting subplots with DataFrame.boxplot with by kw raises ValueError if the number of subplots exceeds 1 (GH7391).
- Bug in subplots displays ticklabels and labels in different rule (GH5897)
- Bug in Panel.apply with a multi-index as an axis (GH7469)
- Bug in DatetimeIndex.insert doesn’t preserve name and tz (GH7299)
- Bug in DatetimeIndex.asobject doesn’t preserve name (GH7299)
- Bug in multi-index slicing with datetimelike ranges (strings and Timestamps), (GH7429)
- Bug in Index.min and max doesn’t handle nan and NaT properly (GH7261)
- Bug in PeriodIndex.min/max results in int (GH7609)
- Bug in resample where fill_method was ignored if you passed how (GH2073)
- Bug in TimeGrouper doesn’t exclude column specified by key (GH7227)
- Bug in DataFrame and Series bar and barh plot raises TypeError when bottom and left keyword is specified (GH7226)
- Bug in DataFrame.hist raises TypeError when it contains non numeric column (GH7277)
- Bug in Index.delete does not preserve name and freq attributes (GH7302)
- Bug in DataFrame.query()/eval where local string variables with the @ sign were being treated as temporaries attempting to be deleted (GH7300).
- Bug in Float64Index which didn’t allow duplicates (GH7149).
- Bug in DataFrame.replace() where truthy values were being replaced (GH7140).
- Bug in StringMethods.extract() where a single match group Series would use the matcher’s name instead of the group name (GH7313).
- Bug in isnull() when mode.use_inf_as_null == True where isnull wouldn’t test True when it encountered an inf/-inf (GH7315).
- Bug in inferred_freq results in None for eastern hemisphere timezones (GH7310)
- Bug in Easter returns incorrect date when offset is negative (GH7195)
- Bug in broadcasting with .div, integer dtypes and divide-by-zero (GH7325)
- Bug in CustomBusinessDay.apply raiases NameError when np.datetime64 object is passed (GH7196)
- Bug in MultiIndex.append, concat and pivot_table don’t preserve timezone (GH6606)
- Bug in .loc with a list of indexers on a single-multi index level (that is not nested) (GH7349)
- Bug in Series.map when mapping a dict with tuple keys of different lengths (GH7333)
- Bug all StringMethods now work on empty Series (GH7242)
- Fix delegation of read_sql to read_sql_query when query does not contain ‘select’ (GH7324).
- Bug where a string column name assignment to a DataFrame with a Float64Index raised a TypeError during a call to np.isnan (GH7366).
- Bug where NDFrame.replace() didn’t correctly replace objects with Period values (GH7379).
- Bug in .ix getitem should always return a Series (GH7150)
- Bug in multi-index slicing with incomplete indexers (GH7399)
- Bug in multi-index slicing with a step in a sliced level (GH7400)
- Bug where negative indexers in DatetimeIndex were not correctly sliced (GH7408)
- Bug where NaT wasn’t repr’d correctly in a MultiIndex (GH7406, GH7409).
- Bug where bool objects were converted to nan in convert_objects (GH7416).
- Bug in quantile ignoring the axis keyword argument (:issue`7306`)
- Bug where nanops._maybe_null_out doesn’t work with complex numbers (GH7353)
- Bug in several nanops functions when axis==0 for 1-dimensional nan arrays (GH7354)
- Bug where nanops.nanmedian doesn’t work when axis==None (GH7352)
- Bug where nanops._has_infs doesn’t work with many dtypes (GH7357)
- Bug in StataReader.data where reading a 0-observation dta failed (GH7369)
- Bug in StataReader when reading Stata 13 (117) files containing fixed width strings (GH7360)
- Bug in StataWriter where encoding was ignored (GH7286)
- Bug in DatetimeIndex comparison doesn’t handle NaT properly (GH7529)
- Bug in passing input with tzinfo to some offsets apply, rollforward or rollback resets tzinfo or raises ValueError (GH7465)
- Bug in DatetimeIndex.to_period, PeriodIndex.asobject, PeriodIndex.to_timestamp doesn’t preserve name (GH7485)
- Bug in DatetimeIndex.to_period and PeriodIndex.to_timestanp handle NaT incorrectly (GH7228)
- Bug in offsets.apply, rollforward and rollback may return normal datetime (GH7502)
- Bug in resample raises ValueError when target contains NaT (GH7227)
- Bug in Timestamp.tz_localize resets nanosecond info (GH7534)
- Bug in DatetimeIndex.asobject raises ValueError when it contains NaT (GH7539)
- Bug in Timestamp.__new__ doesn’t preserve nanosecond properly (GH7610)
- Bug in Index.astype(float) where it would return an object dtype Index (GH7464).
- Bug in DataFrame.reset_index loses tz (GH3950)
- Bug in DatetimeIndex.freqstr raises AttributeError when freq is None (GH7606)
- Bug in GroupBy.size created by TimeGrouper raises AttributeError (GH7453)
- Bug in single column bar plot is misaligned (GH7498).
- Bug in area plot with tz-aware time series raises ValueError (GH7471)
- Bug in non-monotonic Index.union may preserve name incorrectly (GH7458)
- Bug in DatetimeIndex.intersection doesn’t preserve timezone (GH4690)
- Bug in rolling_var where a window larger than the array would raise an error(GH7297)
- Bug with last plotted timeseries dictating xlim (GH2960)
- Bug with secondary_y axis not being considered for timeseries xlim (GH3490)
- Bug in Float64Index assignment with a non scalar indexer (GH7586)
- Bug in pandas.core.strings.str_contains does not properly match in a case insensitive fashion when regex=False and case=False (GH7505)
- Bug in expanding_cov, expanding_corr, rolling_cov, and rolling_corr for two arguments with mismatched index (GH7512)
- Bug in to_sql taking the boolean column as text column (GH7678)
- Bug in grouped hist doesn’t handle rot kw and sharex kw properly (GH7234)
- Bug in .loc performing fallback integer indexing with object dtype indices (GH7496)
- Bug (regression) in PeriodIndex constructor when passed Series objects (GH7701).
v0.14.0 (May 31 , 2014)¶
This is a major release from 0.13.1 and includes a small number of API changes, several new features, enhancements, and performance improvements along with a large number of bug fixes. We recommend that all users upgrade to this version.
- Highlights include:
- Officially support Python 3.4
- SQL interfaces updated to use sqlalchemy, See Here.
- Display interface changes, See Here
- MultiIndexing Using Slicers, See Here.
- Ability to join a singly-indexed DataFrame with a multi-indexed DataFrame, see Here
- More consistency in groupby results and more flexible groupby specifications, See Here
- Holiday calendars are now supported in CustomBusinessDay, see Here
- Several improvements in plotting functions, including: hexbin, area and pie plots, see Here.
- Performance doc section on I/O operations, See Here
- Other Enhancements
- API Changes
- Text Parsing API Changes
- Groupby API Changes
- Performance Improvements
- Prior Deprecations
- Deprecations
- Known Issues
- Bug Fixes
Warning
In 0.14.0 all NDFrame based containers have undergone significant internal refactoring. Before that each block of homogeneous data had its own labels and extra care was necessary to keep those in sync with the parent container’s labels. This should not have any visible user/API behavior changes (GH6745)
API changes¶
read_excel uses 0 as the default sheet (GH6573)
iloc will now accept out-of-bounds indexers for slices, e.g. a value that exceeds the length of the object being indexed. These will be excluded. This will make pandas conform more with python/numpy indexing of out-of-bounds values. A single indexer that is out-of-bounds and drops the dimensions of the object will still raise IndexError (GH6296, GH6299). This could result in an empty axis (e.g. an empty DataFrame being returned)
In [1]: dfl = DataFrame(np.random.randn(5,2),columns=list('AB')) In [2]: dfl Out[2]: A B 0 1.583584 -0.438313 1 -0.402537 -0.780572 2 -0.141685 0.542241 3 0.370966 -0.251642 4 0.787484 1.666563 In [3]: dfl.iloc[:,2:3] Out[3]: Empty DataFrame Columns: [] Index: [0, 1, 2, 3, 4] In [4]: dfl.iloc[:,1:3] Out[4]: B 0 -0.438313 1 -0.780572 2 0.542241 3 -0.251642 4 1.666563 In [5]: dfl.iloc[4:6] Out[5]: A B 4 0.787484 1.666563
These are out-of-bounds selections
dfl.iloc[[4,5,6]] IndexError: positional indexers are out-of-bounds dfl.iloc[:,4] IndexError: single positional indexer is out-of-bounds
Slicing with negative start, stop & step values handles corner cases better (GH6531):
- df.iloc[:-len(df)] is now empty
- df.iloc[len(df)::-1] now enumerates all elements in reverse
The DataFrame.interpolate() keyword downcast default has been changed from infer to None. This is to preseve the original dtype unless explicitly requested otherwise (GH6290).
When converting a dataframe to HTML it used to return Empty DataFrame. This special case has been removed, instead a header with the column names is returned (GH6062).
Series and Index now internall share more common operations, e.g. factorize(),nunique(),value_counts() are now supported on Index types as well. The Series.weekday property from is removed from Series for API consistency. Using a DatetimeIndex/PeriodIndex method on a Series will now raise a TypeError. (GH4551, GH4056, GH5519, GH6380, GH7206).
Add is_month_start, is_month_end, is_quarter_start, is_quarter_end, is_year_start, is_year_end accessors for DateTimeIndex / Timestamp which return a boolean array of whether the timestamp(s) are at the start/end of the month/quarter/year defined by the frequency of the DateTimeIndex / Timestamp (GH4565, GH6998)
Local variable usage has changed in pandas.eval()/DataFrame.eval()/DataFrame.query() (GH5987). For the DataFrame methods, two things have changed
- Column names are now given precedence over locals
- Local variables must be referred to explicitly. This means that even if you have a local variable that is not a column you must still refer to it with the '@' prefix.
- You can have an expression like df.query('@a < a') with no complaints from pandas about ambiguity of the name a.
- The top-level pandas.eval() function does not allow you use the '@' prefix and provides you with an error message telling you so.
- NameResolutionError was removed because it isn’t necessary anymore.
Define and document the order of column vs index names in query/eval (GH6676)
concat will now concatenate mixed Series and DataFrames using the Series name or numbering columns as needed (GH2385). See the docs
Slicing and advanced/boolean indexing operations on Index classes as well as Index.delete() and Index.drop() methods will no longer change the type of the resulting index (GH6440, GH7040)
In [6]: i = pd.Index([1, 2, 3, 'a' , 'b', 'c']) In [7]: i[[0,1,2]] Out[7]: Index([1, 2, 3], dtype='object') In [8]: i.drop(['a', 'b', 'c']) Out[8]: Index([1, 2, 3], dtype='object')
Previously, the above operation would return Int64Index. If you’d like to do this manually, use Index.astype()
In [9]: i[[0,1,2]].astype(np.int_) Out[9]: Int64Index([1, 2, 3], dtype='int32')
set_index no longer converts MultiIndexes to an Index of tuples. For example, the old behavior returned an Index in this case (GH6459):
# Old behavior, casted MultiIndex to an Index In [10]: tuple_ind Out[10]: Index([(u'a', u'c'), (u'a', u'd'), (u'b', u'c'), (u'b', u'd')], dtype='object') In [11]: df_multi.set_index(tuple_ind) Out[11]: 0 1 (a, c) 0.471435 -1.190976 (a, d) 1.432707 -0.312652 (b, c) -0.720589 0.887163 (b, d) 0.859588 -0.636524 # New behavior In [12]: mi Out[12]: MultiIndex(levels=[[u'a', u'b'], [u'c', u'd']], labels=[[0, 0, 1, 1], [0, 1, 0, 1]]) In [13]: df_multi.set_index(mi) Out[13]: 0 1 a c 0.471435 -1.190976 d 1.432707 -0.312652 b c -0.720589 0.887163 d 0.859588 -0.636524
This also applies when passing multiple indices to set_index:
# Old output, 2-level MultiIndex of tuples In [14]: df_multi.set_index([df_multi.index, df_multi.index]) Out[14]: 0 1 (a, c) (a, c) 0.471435 -1.190976 (a, d) (a, d) 1.432707 -0.312652 (b, c) (b, c) -0.720589 0.887163 (b, d) (b, d) 0.859588 -0.636524 # New output, 4-level MultiIndex In [15]: df_multi.set_index([df_multi.index, df_multi.index]) Out[15]: 0 1 a c a c 0.471435 -1.190976 d a d 1.432707 -0.312652 b c b c -0.720589 0.887163 d b d 0.859588 -0.636524
pairwise keyword was added to the statistical moment functions rolling_cov, rolling_corr, ewmcov, ewmcorr, expanding_cov, expanding_corr to allow the calculation of moving window covariance and correlation matrices (GH4950). See Computing rolling pairwise covariances and correlations in the docs.
In [16]: df = DataFrame(np.random.randn(10,4),columns=list('ABCD')) In [17]: covs = rolling_cov(df[['A','B','C']], df[['B','C','D']], 5, pairwise=True) In [18]: covs[df.index[-1]] Out[18]: B C D A 0.128104 0.183628 -0.047358 B 0.856265 0.058945 0.145447 C 0.058945 0.335350 0.390637
Series.iteritems() is now lazy (returns an iterator rather than a list). This was the documented behavior prior to 0.14. (GH6760)
Added nunique and value_counts functions to Index for counting unique elements. (GH6734)
stack and unstack now raise a ValueError when the level keyword refers to a non-unique item in the Index (previously raised a KeyError). (GH6738)
drop unused order argument from Series.sort; args now are in the same order as Series.order; add na_position arg to conform to Series.order (GH6847)
default sorting algorithm for Series.order is now quicksort, to conform with Series.sort (and numpy defaults)
add inplace keyword to Series.order/sort to make them inverses (GH6859)
DataFrame.sort now places NaNs at the beginning or end of the sort according to the na_position parameter. (GH3917)
accept TextFileReader in concat, which was affecting a common user idiom (GH6583), this was a regression from 0.13.1
Added factorize functions to Index and Series to get indexer and unique values (GH7090)
describe on a DataFrame with a mix of Timestamp and string like objects returns a different Index (GH7088). Previously the index was unintentionally sorted.
Arithmetic operations with only bool dtypes now give a warning indicating that they are evaluated in Python space for +, -, and * operations and raise for all others (GH7011, GH6762, GH7015, GH7210)
x = pd.Series(np.random.rand(10) > 0.5) y = True x + y # warning generated: should do x | y instead x / y # this raises because it doesn't make sense NotImplementedError: operator '/' not implemented for bool dtypes
In HDFStore, select_as_multiple will always raise a KeyError, when a key or the selector is not found (GH6177)
df['col'] = value and df.loc[:,'col'] = value are now completely equivalent; previously the .loc would not necessarily coerce the dtype of the resultant series (GH6149)
dtypes and ftypes now return a series with dtype=object on empty containers (GH5740)
df.to_csv will now return a string of the CSV data if neither a target path nor a buffer is provided (GH6061)
pd.infer_freq() will now raise a TypeError if given an invalid Series/Index type (GH6407, GH6463)
A tuple passed to DataFame.sort_index will be interpreted as the levels of the index, rather than requiring a list of tuple (GH4370)
all offset operations now return Timestamp types (rather than datetime), Business/Week frequencies were incorrect (GH4069)
to_excel now converts np.inf into a string representation, customizable by the inf_rep keyword argument (Excel has no native inf representation) (GH6782)
Replace pandas.compat.scipy.scoreatpercentile with numpy.percentile (GH6810)
.quantile on a datetime[ns] series now returns Timestamp instead of np.datetime64 objects (GH6810)
change AssertionError to TypeError for invalid types passed to concat (GH6583)
Raise a TypeError when DataFrame is passed an iterator as the data argument (GH5357)
Display Changes¶
The default way of printing large DataFrames has changed. DataFrames exceeding max_rows and/or max_columns are now displayed in a centrally truncated view, consistent with the printing of a pandas.Series (GH5603).
In previous versions, a DataFrame was truncated once the dimension constraints were reached and an ellipse (...) signaled that part of the data was cut off.
In the current version, large DataFrames are centrally truncated, showing a preview of head and tail in both dimensions.
allow option 'truncate' for display.show_dimensions to only show the dimensions if the frame is truncated (GH6547).
The default for display.show_dimensions will now be truncate. This is consistent with how Series display length.
In [19]: dfd = pd.DataFrame(np.arange(25).reshape(-1,5), index=[0,1,2,3,4], columns=[0,1,2,3,4]) # show dimensions since this is truncated In [20]: with pd.option_context('display.max_rows', 2, 'display.max_columns', 2, ....: 'display.show_dimensions', 'truncate'): ....: print(dfd) ....: 0 ... 4 0 0 ... 4 .. .. ... .. 4 20 ... 24 [5 rows x 5 columns] # will not show dimensions since it is not truncated In [21]: with pd.option_context('display.max_rows', 10, 'display.max_columns', 40, ....: 'display.show_dimensions', 'truncate'): ....: print(dfd) ....: 0 1 2 3 4 0 0 1 2 3 4 1 5 6 7 8 9 2 10 11 12 13 14 3 15 16 17 18 19 4 20 21 22 23 24
Regression in the display of a MultiIndexed Series with display.max_rows is less than the length of the series (GH7101)
Fixed a bug in the HTML repr of a truncated Series or DataFrame not showing the class name with the large_repr set to ‘info’ (GH7105)
The verbose keyword in DataFrame.info(), which controls whether to shorten the info representation, is now None by default. This will follow the global setting in display.max_info_columns. The global setting can be overriden with verbose=True or verbose=False.
Fixed a bug with the info repr not honoring the display.max_info_columns setting (GH6939)
Offset/freq info now in Timestamp __repr__ (GH4553)
Text Parsing API Changes¶
read_csv()/read_table() will now be noiser w.r.t invalid options rather than falling back to the PythonParser.
- Raise ValueError when sep specified with delim_whitespace=True in read_csv()/read_table() (GH6607)
- Raise ValueError when engine='c' specified with unsupported options in read_csv()/read_table() (GH6607)
- Raise ValueError when fallback to python parser causes options to be ignored (GH6607)
- Produce ParserWarning on fallback to python parser when no options are ignored (GH6607)
- Translate sep='\s+' to delim_whitespace=True in read_csv()/read_table() if no other C-unsupported options specified (GH6607)
Groupby API Changes¶
More consistent behaviour for some groupby methods:
groupby head and tail now act more like filter rather than an aggregation:
In [22]: df = pd.DataFrame([[1, 2], [1, 4], [5, 6]], columns=['A', 'B']) In [23]: g = df.groupby('A') In [24]: g.head(1) # filters DataFrame Out[24]: A B 0 1 2 2 5 6 In [25]: g.apply(lambda x: x.head(1)) # used to simply fall-through Out[25]: A B A 1 0 1 2 5 2 5 6
groupby head and tail respect column selection:
In [26]: g[['B']].head(1) Out[26]: B 0 2 2 6
groupby nth now reduces by default; filtering can be achieved by passing as_index=False. With an optional dropna argument to ignore NaN. See the docs.
Reducing
In [27]: df = DataFrame([[1, np.nan], [1, 4], [5, 6]], columns=['A', 'B']) In [28]: g = df.groupby('A') In [29]: g.nth(0) Out[29]: B A 1 NaN 5 6 # this is equivalent to g.first() In [30]: g.nth(0, dropna='any') Out[30]: B A 1 4 5 6 # this is equivalent to g.last() In [31]: g.nth(-1, dropna='any') Out[31]: B A 1 4 5 6
Filtering
In [32]: gf = df.groupby('A',as_index=False) In [33]: gf.nth(0) Out[33]: A B 0 1 NaN 2 5 6 In [34]: gf.nth(0, dropna='any') Out[34]: B A 1 4 5 6
groupby will now not return the grouped column for non-cython functions (GH5610, GH5614, GH6732), as its already the index
In [35]: df = DataFrame([[1, np.nan], [1, 4], [5, 6], [5, 8]], columns=['A', 'B']) In [36]: g = df.groupby('A') In [37]: g.count() Out[37]: B A 1 1 5 2 In [38]: g.describe() Out[38]: B A 1 count 1.000000 mean 4.000000 std NaN min 4.000000 25% 4.000000 50% 4.000000 75% 4.000000 ... ... 5 mean 7.000000 std 1.414214 min 6.000000 25% 6.500000 50% 7.000000 75% 7.500000 max 8.000000 [16 rows x 1 columns]
passing as_index will leave the grouped column in-place (this is not change in 0.14.0)
In [39]: df = DataFrame([[1, np.nan], [1, 4], [5, 6], [5, 8]], columns=['A', 'B']) In [40]: g = df.groupby('A',as_index=False) In [41]: g.count() Out[41]: A B 0 1 1 1 5 2 In [42]: g.describe() Out[42]: A B 0 count 2 1.000000 mean 1 4.000000 std 0 NaN min 1 4.000000 25% 1 4.000000 50% 1 4.000000 75% 1 4.000000 ... .. ... 1 mean 5 7.000000 std 0 1.414214 min 5 6.000000 25% 5 6.500000 50% 5 7.000000 75% 5 7.500000 max 5 8.000000 [16 rows x 2 columns]
Allow specification of a more complex groupby via pd.Grouper, such as grouping by a Time and a string field simultaneously. See the docs. (GH3794)
Better propagation/preservation of Series names when performing groupby operations:
- SeriesGroupBy.agg will ensure that the name attribute of the original series is propagated to the result (GH6265).
- If the function provided to GroupBy.apply returns a named series, the name of the series will be kept as the name of the column index of the DataFrame returned by GroupBy.apply (GH6124). This facilitates DataFrame.stack operations where the name of the column index is used as the name of the inserted column containing the pivoted data.
SQL¶
The SQL reading and writing functions now support more database flavors through SQLAlchemy (GH2717, GH4163, GH5950, GH6292). All databases supported by SQLAlchemy can be used, such as PostgreSQL, MySQL, Oracle, Microsoft SQL server (see documentation of SQLAlchemy on included dialects).
The functionality of providing DBAPI connection objects will only be supported for sqlite3 in the future. The 'mysql' flavor is deprecated.
The new functions read_sql_query() and read_sql_table() are introduced. The function read_sql() is kept as a convenience wrapper around the other two and will delegate to specific function depending on the provided input (database table name or sql query).
In practice, you have to provide a SQLAlchemy engine to the sql functions. To connect with SQLAlchemy you use the create_engine() function to create an engine object from database URI. You only need to create the engine once per database you are connecting to. For an in-memory sqlite database:
In [43]: from sqlalchemy import create_engine
# Create your connection.
In [44]: engine = create_engine('sqlite:///:memory:')
This engine can then be used to write or read data to/from this database:
In [45]: df = pd.DataFrame({'A': [1,2,3], 'B': ['a', 'b', 'c']})
In [46]: df.to_sql('db_table', engine, index=False)
You can read data from a database by specifying the table name:
In [47]: pd.read_sql_table('db_table', engine)
Out[47]:
A B
0 1 a
1 2 b
2 3 c
or by specifying a sql query:
In [48]: pd.read_sql_query('SELECT * FROM db_table', engine)
Out[48]:
A B
0 1 a
1 2 b
2 3 c
Some other enhancements to the sql functions include:
- support for writing the index. This can be controlled with the index keyword (default is True).
- specify the column label to use when writing the index with index_label.
- specify string columns to parse as datetimes withh the parse_dates keyword in read_sql_query() and read_sql_table().
Warning
Some of the existing functions or function aliases have been deprecated and will be removed in future versions. This includes: tquery, uquery, read_frame, frame_query, write_frame.
Warning
The support for the ‘mysql’ flavor when using DBAPI connection objects has been deprecated. MySQL will be further supported with SQLAlchemy engines (GH6900).
MultiIndexing Using Slicers¶
In 0.14.0 we added a new way to slice multi-indexed objects. You can slice a multi-index by providing multiple indexers.
You can provide any of the selectors as if you are indexing by label, see Selection by Label, including slices, lists of labels, labels, and boolean indexers.
You can use slice(None) to select all the contents of that level. You do not need to specify all the deeper levels, they will be implied as slice(None).
As usual, both sides of the slicers are included as this is label indexing.
See the docs See also issues (GH6134, GH4036, GH3057, GH2598, GH5641, GH7106)
Warning
You should specify all axes in the .loc specifier, meaning the indexer for the index and for the columns. Their are some ambiguous cases where the passed indexer could be mis-interpreted as indexing both axes, rather than into say the MuliIndex for the rows.
You should do this:
df.loc[(slice('A1','A3'),.....),:]
rather than this:
df.loc[(slice('A1','A3'),.....)]
Warning
You will need to make sure that the selection axes are fully lexsorted!
In [49]: def mklbl(prefix,n):
....: return ["%s%s" % (prefix,i) for i in range(n)]
....:
In [50]: index = MultiIndex.from_product([mklbl('A',4),
....: mklbl('B',2),
....: mklbl('C',4),
....: mklbl('D',2)])
....:
In [51]: columns = MultiIndex.from_tuples([('a','foo'),('a','bar'),
....: ('b','foo'),('b','bah')],
....: names=['lvl0', 'lvl1'])
....:
In [52]: df = DataFrame(np.arange(len(index)*len(columns)).reshape((len(index),len(columns))),
....: index=index,
....: columns=columns).sortlevel().sortlevel(axis=1)
....:
In [53]: df
Out[53]:
lvl0 a b
lvl1 bar foo bah foo
A0 B0 C0 D0 1 0 3 2
D1 5 4 7 6
C1 D0 9 8 11 10
D1 13 12 15 14
C2 D0 17 16 19 18
D1 21 20 23 22
C3 D0 25 24 27 26
... ... ... ... ...
A3 B1 C0 D1 229 228 231 230
C1 D0 233 232 235 234
D1 237 236 239 238
C2 D0 241 240 243 242
D1 245 244 247 246
C3 D0 249 248 251 250
D1 253 252 255 254
[64 rows x 4 columns]
Basic multi-index slicing using slices, lists, and labels.
In [54]: df.loc[(slice('A1','A3'),slice(None), ['C1','C3']),:]
Out[54]:
lvl0 a b
lvl1 bar foo bah foo
A1 B0 C1 D0 73 72 75 74
D1 77 76 79 78
C3 D0 89 88 91 90
D1 93 92 95 94
B1 C1 D0 105 104 107 106
D1 109 108 111 110
C3 D0 121 120 123 122
... ... ... ... ...
A3 B0 C1 D1 205 204 207 206
C3 D0 217 216 219 218
D1 221 220 223 222
B1 C1 D0 233 232 235 234
D1 237 236 239 238
C3 D0 249 248 251 250
D1 253 252 255 254
[24 rows x 4 columns]
You can use a pd.IndexSlice to shortcut the creation of these slices
In [55]: idx = pd.IndexSlice
In [56]: df.loc[idx[:,:,['C1','C3']],idx[:,'foo']]
Out[56]:
lvl0 a b
lvl1 foo foo
A0 B0 C1 D0 8 10
D1 12 14
C3 D0 24 26
D1 28 30
B1 C1 D0 40 42
D1 44 46
C3 D0 56 58
... ... ...
A3 B0 C1 D1 204 206
C3 D0 216 218
D1 220 222
B1 C1 D0 232 234
D1 236 238
C3 D0 248 250
D1 252 254
[32 rows x 2 columns]
It is possible to perform quite complicated selections using this method on multiple axes at the same time.
In [57]: df.loc['A1',(slice(None),'foo')]
Out[57]:
lvl0 a b
lvl1 foo foo
B0 C0 D0 64 66
D1 68 70
C1 D0 72 74
D1 76 78
C2 D0 80 82
D1 84 86
C3 D0 88 90
... ... ...
B1 C0 D1 100 102
C1 D0 104 106
D1 108 110
C2 D0 112 114
D1 116 118
C3 D0 120 122
D1 124 126
[16 rows x 2 columns]
In [58]: df.loc[idx[:,:,['C1','C3']],idx[:,'foo']]
Out[58]:
lvl0 a b
lvl1 foo foo
A0 B0 C1 D0 8 10
D1 12 14
C3 D0 24 26
D1 28 30
B1 C1 D0 40 42
D1 44 46
C3 D0 56 58
... ... ...
A3 B0 C1 D1 204 206
C3 D0 216 218
D1 220 222
B1 C1 D0 232 234
D1 236 238
C3 D0 248 250
D1 252 254
[32 rows x 2 columns]
Using a boolean indexer you can provide selection related to the values.
In [59]: mask = df[('a','foo')]>200
In [60]: df.loc[idx[mask,:,['C1','C3']],idx[:,'foo']]
Out[60]:
lvl0 a b
lvl1 foo foo
A3 B0 C1 D1 204 206
C3 D0 216 218
D1 220 222
B1 C1 D0 232 234
D1 236 238
C3 D0 248 250
D1 252 254
You can also specify the axis argument to .loc to interpret the passed slicers on a single axis.
In [61]: df.loc(axis=0)[:,:,['C1','C3']]
Out[61]:
lvl0 a b
lvl1 bar foo bah foo
A0 B0 C1 D0 9 8 11 10
D1 13 12 15 14
C3 D0 25 24 27 26
D1 29 28 31 30
B1 C1 D0 41 40 43 42
D1 45 44 47 46
C3 D0 57 56 59 58
... ... ... ... ...
A3 B0 C1 D1 205 204 207 206
C3 D0 217 216 219 218
D1 221 220 223 222
B1 C1 D0 233 232 235 234
D1 237 236 239 238
C3 D0 249 248 251 250
D1 253 252 255 254
[32 rows x 4 columns]
Furthermore you can set the values using these methods
In [62]: df2 = df.copy()
In [63]: df2.loc(axis=0)[:,:,['C1','C3']] = -10
In [64]: df2
Out[64]:
lvl0 a b
lvl1 bar foo bah foo
A0 B0 C0 D0 1 0 3 2
D1 5 4 7 6
C1 D0 -10 -10 -10 -10
D1 -10 -10 -10 -10
C2 D0 17 16 19 18
D1 21 20 23 22
C3 D0 -10 -10 -10 -10
... ... ... ... ...
A3 B1 C0 D1 229 228 231 230
C1 D0 -10 -10 -10 -10
D1 -10 -10 -10 -10
C2 D0 241 240 243 242
D1 245 244 247 246
C3 D0 -10 -10 -10 -10
D1 -10 -10 -10 -10
[64 rows x 4 columns]
You can use a right-hand-side of an alignable object as well.
In [65]: df2 = df.copy()
In [66]: df2.loc[idx[:,:,['C1','C3']],:] = df2*1000
In [67]: df2
Out[67]:
lvl0 a b
lvl1 bar foo bah foo
A0 B0 C0 D0 1 0 3 2
D1 5 4 7 6
C1 D0 9000 8000 11000 10000
D1 13000 12000 15000 14000
C2 D0 17 16 19 18
D1 21 20 23 22
C3 D0 25000 24000 27000 26000
... ... ... ... ...
A3 B1 C0 D1 229 228 231 230
C1 D0 233000 232000 235000 234000
D1 237000 236000 239000 238000
C2 D0 241 240 243 242
D1 245 244 247 246
C3 D0 249000 248000 251000 250000
D1 253000 252000 255000 254000
[64 rows x 4 columns]
Plotting¶
Hexagonal bin plots from DataFrame.plot with kind='hexbin' (GH5478), See the docs.
DataFrame.plot and Series.plot now supports area plot with specifying kind='area' (GH6656), See the docs
Pie plots from Series.plot and DataFrame.plot with kind='pie' (GH6976), See the docs.
Plotting with Error Bars is now supported in the .plot method of DataFrame and Series objects (GH3796, GH6834), See the docs.
DataFrame.plot and Series.plot now support a table keyword for plotting matplotlib.Table, See the docs. The table keyword can receive the following values.
- False: Do nothing (default).
- True: Draw a table using the DataFrame or Series called plot method. Data will be transposed to meet matplotlib’s default layout.
- DataFrame or Series: Draw matplotlib.table using the passed data. The data will be drawn as displayed in print method (not transposed automatically). Also, helper function pandas.tools.plotting.table is added to create a table from DataFrame and Series, and add it to an matplotlib.Axes.
plot(legend='reverse') will now reverse the order of legend labels for most plot kinds. (GH6014)
Line plot and area plot can be stacked by stacked=True (GH6656)
Following keywords are now acceptable for DataFrame.plot() with kind='bar' and kind='barh':
- width: Specify the bar width. In previous versions, static value 0.5 was passed to matplotlib and it cannot be overwritten. (GH6604)
- align: Specify the bar alignment. Default is center (different from matplotlib). In previous versions, pandas passes align=’edge’ to matplotlib and adjust the location to center by itself, and it results align keyword is not applied as expected. (GH4525)
- position: Specify relative alignments for bar plot layout. From 0 (left/bottom-end) to 1(right/top-end). Default is 0.5 (center). (GH6604)
Because of the default align value changes, coordinates of bar plots are now located on integer values (0.0, 1.0, 2.0 ...). This is intended to make bar plot be located on the same coodinates as line plot. However, bar plot may differs unexpectedly when you manually adjust the bar location or drawing area, such as using set_xlim, set_ylim, etc. In this cases, please modify your script to meet with new coordinates.
The parallel_coordinates() function now takes argument color instead of colors. A FutureWarning is raised to alert that the old colors argument will not be supported in a future release. (GH6956)
The parallel_coordinates() and andrews_curves() functions now take positional argument frame instead of data. A FutureWarning is raised if the old data argument is used by name. (GH6956)
DataFrame.boxplot() now supports layout keyword (GH6769)
DataFrame.boxplot() has a new keyword argument, return_type. It accepts 'dict', 'axes', or 'both', in which case a namedtuple with the matplotlib axes and a dict of matplotlib Lines is returned.
Prior Version Deprecations/Changes¶
There are prior version deprecations that are taking effect as of 0.14.0.
- Remove DateRange in favor of DatetimeIndex (GH6816)
- Remove column keyword from DataFrame.sort (GH4370)
- Remove precision keyword from set_eng_float_format() (GH395)
- Remove force_unicode keyword from DataFrame.to_string(), DataFrame.to_latex(), and DataFrame.to_html(); these function encode in unicode by default (GH2224, GH2225)
- Remove nanRep keyword from DataFrame.to_csv() and DataFrame.to_string() (GH275)
- Remove unique keyword from HDFStore.select_column() (GH3256)
- Remove inferTimeRule keyword from Timestamp.offset() (GH391)
- Remove name keyword from get_data_yahoo() and get_data_google() ( commit b921d1a )
- Remove offset keyword from DatetimeIndex constructor ( commit 3136390 )
- Remove time_rule from several rolling-moment statistical functions, such as rolling_sum() (GH1042)
- Removed neg - boolean operations on numpy arrays in favor of inv ~, as this is going to be deprecated in numpy 1.9 (GH6960)
Deprecations¶
The pivot_table()/DataFrame.pivot_table() and crosstab() functions now take arguments index and columns instead of rows and cols. A FutureWarning is raised to alert that the old rows and cols arguments will not be supported in a future release (GH5505)
The DataFrame.drop_duplicates() and DataFrame.duplicated() methods now take argument subset instead of cols to better align with DataFrame.dropna(). A FutureWarning is raised to alert that the old cols arguments will not be supported in a future release (GH6680)
The DataFrame.to_csv() and DataFrame.to_excel() functions now takes argument columns instead of cols. A FutureWarning is raised to alert that the old cols arguments will not be supported in a future release (GH6645)
Indexers will warn FutureWarning when used with a scalar indexer and a non-floating point Index (GH4892, GH6960)
# non-floating point indexes can only be indexed by integers / labels In [1]: Series(1,np.arange(5))[3.0] pandas/core/index.py:469: FutureWarning: scalar indexers for index type Int64Index should be integers and not floating point Out[1]: 1 In [2]: Series(1,np.arange(5)).iloc[3.0] pandas/core/index.py:469: FutureWarning: scalar indexers for index type Int64Index should be integers and not floating point Out[2]: 1 In [3]: Series(1,np.arange(5)).iloc[3.0:4] pandas/core/index.py:527: FutureWarning: slice indexers when using iloc should be integers and not floating point Out[3]: 3 1 dtype: int64 # these are Float64Indexes, so integer or floating point is acceptable In [4]: Series(1,np.arange(5.))[3] Out[4]: 1 In [5]: Series(1,np.arange(5.))[3.0] Out[6]: 1
Numpy 1.9 compat w.r.t. deprecation warnings (GH6960)
Panel.shift() now has a function signature that matches DataFrame.shift(). The old positional argument lags has been changed to a keyword argument periods with a default value of 1. A FutureWarning is raised if the old argument lags is used by name. (GH6910)
The order keyword argument of factorize() will be removed. (GH6926).
Remove the copy keyword from DataFrame.xs(), Panel.major_xs(), Panel.minor_xs(). A view will be returned if possible, otherwise a copy will be made. Previously the user could think that copy=False would ALWAYS return a view. (GH6894)
The parallel_coordinates() function now takes argument color instead of colors. A FutureWarning is raised to alert that the old colors argument will not be supported in a future release. (GH6956)
The parallel_coordinates() and andrews_curves() functions now take positional argument frame instead of data. A FutureWarning is raised if the old data argument is used by name. (GH6956)
The support for the ‘mysql’ flavor when using DBAPI connection objects has been deprecated. MySQL will be further supported with SQLAlchemy engines (GH6900).
The following io.sql functions have been deprecated: tquery, uquery, read_frame, frame_query, write_frame.
The percentile_width keyword argument in describe() has been deprecated. Use the percentiles keyword instead, which takes a list of percentiles to display. The default output is unchanged.
The default return type of boxplot() will change from a dict to a matpltolib Axes in a future release. You can use the future behavior now by passing return_type='axes' to boxplot.
Enhancements¶
DataFrame and Series will create a MultiIndex object if passed a tuples dict, See the docs (GH3323)
In [68]: Series({('a', 'b'): 1, ('a', 'a'): 0, ....: ('a', 'c'): 2, ('b', 'a'): 3, ('b', 'b'): 4}) ....: Out[68]: a a 0 b 1 c 2 b a 3 b 4 dtype: int64 In [69]: DataFrame({('a', 'b'): {('A', 'B'): 1, ('A', 'C'): 2}, ....: ('a', 'a'): {('A', 'C'): 3, ('A', 'B'): 4}, ....: ('a', 'c'): {('A', 'B'): 5, ('A', 'C'): 6}, ....: ('b', 'a'): {('A', 'C'): 7, ('A', 'B'): 8}, ....: ('b', 'b'): {('A', 'D'): 9, ('A', 'B'): 10}}) ....: Out[69]: a b a b c a b A B 4 1 5 8 10 C 3 2 6 7 NaN D NaN NaN NaN NaN 9
Added the sym_diff method to Index (GH5543)
DataFrame.to_latex now takes a longtable keyword, which if True will return a table in a longtable environment. (GH6617)
Add option to turn off escaping in DataFrame.to_latex (GH6472)
pd.read_clipboard will, if the keyword sep is unspecified, try to detect data copied from a spreadsheet and parse accordingly. (GH6223)
Joining a singly-indexed DataFrame with a multi-indexed DataFrame (GH3662)
See the docs. Joining multi-index DataFrames on both the left and right is not yet supported ATM.
In [70]: household = DataFrame(dict(household_id = [1,2,3], ....: male = [0,1,0], ....: wealth = [196087.3,316478.7,294750]), ....: columns = ['household_id','male','wealth'] ....: ).set_index('household_id') ....: In [71]: household Out[71]: male wealth household_id 1 0 196087.3 2 1 316478.7 3 0 294750.0 In [72]: portfolio = DataFrame(dict(household_id = [1,2,2,3,3,3,4], ....: asset_id = ["nl0000301109","nl0000289783","gb00b03mlx29", ....: "gb00b03mlx29","lu0197800237","nl0000289965",np.nan], ....: name = ["ABN Amro","Robeco","Royal Dutch Shell","Royal Dutch Shell", ....: "AAB Eastern Europe Equity Fund","Postbank BioTech Fonds",np.nan], ....: share = [1.0,0.4,0.6,0.15,0.6,0.25,1.0]), ....: columns = ['household_id','asset_id','name','share'] ....: ).set_index(['household_id','asset_id']) ....: In [73]: portfolio Out[73]: name share household_id asset_id 1 nl0000301109 ABN Amro 1.00 2 nl0000289783 Robeco 0.40 gb00b03mlx29 Royal Dutch Shell 0.60 3 gb00b03mlx29 Royal Dutch Shell 0.15 lu0197800237 AAB Eastern Europe Equity Fund 0.60 nl0000289965 Postbank BioTech Fonds 0.25 4 NaN NaN 1.00 In [74]: household.join(portfolio, how='inner') Out[74]: male wealth name \ household_id asset_id 1 nl0000301109 0 196087.3 ABN Amro 2 nl0000289783 1 316478.7 Robeco gb00b03mlx29 1 316478.7 Royal Dutch Shell 3 gb00b03mlx29 0 294750.0 Royal Dutch Shell lu0197800237 0 294750.0 AAB Eastern Europe Equity Fund nl0000289965 0 294750.0 Postbank BioTech Fonds share household_id asset_id 1 nl0000301109 1.00 2 nl0000289783 0.40 gb00b03mlx29 0.60 3 gb00b03mlx29 0.15 lu0197800237 0.60 nl0000289965 0.25
quotechar, doublequote, and escapechar can now be specified when using DataFrame.to_csv (GH5414, GH4528)
Partially sort by only the specified levels of a MultiIndex with the sort_remaining boolean kwarg. (GH3984)
Added to_julian_date to TimeStamp and DatetimeIndex. The Julian Date is used primarily in astronomy and represents the number of days from noon, January 1, 4713 BC. Because nanoseconds are used to define the time in pandas the actual range of dates that you can use is 1678 AD to 2262 AD. (GH4041)
DataFrame.to_stata will now check data for compatibility with Stata data types and will upcast when needed. When it is not possible to losslessly upcast, a warning is issued (GH6327)
DataFrame.to_stata and StataWriter will accept keyword arguments time_stamp and data_label which allow the time stamp and dataset label to be set when creating a file. (GH6545)
pandas.io.gbq now handles reading unicode strings properly. (GH5940)
Holidays Calendars are now available and can be used with the CustomBusinessDay offset (GH6719)
Float64Index is now backed by a float64 dtype ndarray instead of an object dtype array (GH6471).
Implemented Panel.pct_change (GH6904)
Added how option to rolling-moment functions to dictate how to handle resampling; rolling_max() defaults to max, rolling_min() defaults to min, and all others default to mean (GH6297)
CustomBuisnessMonthBegin and CustomBusinessMonthEnd are now available (GH6866)
Series.quantile() and DataFrame.quantile() now accept an array of quantiles.
describe() now accepts an array of percentiles to include in the summary statistics (GH4196)
pivot_table can now accept Grouper by index and columns keywords (GH6913)
In [75]: import datetime In [76]: df = DataFrame({ ....: 'Branch' : 'A A A A A B'.split(), ....: 'Buyer': 'Carl Mark Carl Carl Joe Joe'.split(), ....: 'Quantity': [1, 3, 5, 1, 8, 1], ....: 'Date' : [datetime.datetime(2013,11,1,13,0), datetime.datetime(2013,9,1,13,5), ....: datetime.datetime(2013,10,1,20,0), datetime.datetime(2013,10,2,10,0), ....: datetime.datetime(2013,11,1,20,0), datetime.datetime(2013,10,2,10,0)], ....: 'PayDay' : [datetime.datetime(2013,10,4,0,0), datetime.datetime(2013,10,15,13,5), ....: datetime.datetime(2013,9,5,20,0), datetime.datetime(2013,11,2,10,0), ....: datetime.datetime(2013,10,7,20,0), datetime.datetime(2013,9,5,10,0)]}) ....: In [77]: df Out[77]: Branch Buyer Date PayDay Quantity 0 A Carl 2013-11-01 13:00:00 2013-10-04 00:00:00 1 1 A Mark 2013-09-01 13:05:00 2013-10-15 13:05:00 3 2 A Carl 2013-10-01 20:00:00 2013-09-05 20:00:00 5 3 A Carl 2013-10-02 10:00:00 2013-11-02 10:00:00 1 4 A Joe 2013-11-01 20:00:00 2013-10-07 20:00:00 8 5 B Joe 2013-10-02 10:00:00 2013-09-05 10:00:00 1 In [78]: pivot_table(df, index=Grouper(freq='M', key='Date'), ....: columns=Grouper(freq='M', key='PayDay'), ....: values='Quantity', aggfunc=np.sum) ....: Out[78]: PayDay 2013-09-30 2013-10-31 2013-11-30 Date 2013-09-30 NaN 3 NaN 2013-10-31 6 NaN 1 2013-11-30 NaN 9 NaN
Arrays of strings can be wrapped to a specified width (str.wrap) (GH6999)
Add nsmallest() and Series.nlargest() methods to Series, See the docs (GH3960)
PeriodIndex fully supports partial string indexing like DatetimeIndex (GH7043)
In [79]: prng = period_range('2013-01-01 09:00', periods=100, freq='H') In [80]: ps = Series(np.random.randn(len(prng)), index=prng) In [81]: ps Out[81]: 2013-01-01 09:00 0.755414 2013-01-01 10:00 0.215269 2013-01-01 11:00 0.841009 2013-01-01 12:00 -1.445810 2013-01-01 13:00 -1.401973 2013-01-01 14:00 -0.100918 2013-01-01 15:00 -0.548242 ... 2013-01-05 06:00 -0.379811 2013-01-05 07:00 0.702562 2013-01-05 08:00 -0.850346 2013-01-05 09:00 1.176812 2013-01-05 10:00 -0.524336 2013-01-05 11:00 0.700908 2013-01-05 12:00 0.984188 Freq: H, dtype: float64 In [82]: ps['2013-01-02'] Out[82]: 2013-01-02 00:00 -0.208499 2013-01-02 01:00 1.033801 2013-01-02 02:00 -2.400454 2013-01-02 03:00 2.030604 2013-01-02 04:00 -1.142631 2013-01-02 05:00 0.211883 2013-01-02 06:00 0.704721 ... 2013-01-02 17:00 0.464392 2013-01-02 18:00 -3.563517 2013-01-02 19:00 1.321106 2013-01-02 20:00 0.152631 2013-01-02 21:00 0.164530 2013-01-02 22:00 -0.430096 2013-01-02 23:00 0.767369 Freq: H, dtype: float64
read_excel can now read milliseconds in Excel dates and times with xlrd >= 0.9.3. (GH5945)
pd.stats.moments.rolling_var now uses Welford’s method for increased numerical stability (GH6817)
pd.expanding_apply and pd.rolling_apply now take args and kwargs that are passed on to the func (GH6289)
DataFrame.rank() now has a percentage rank option (GH5971)
Series.rank() now has a percentage rank option (GH5971)
Series.rank() and DataFrame.rank() now accept method='dense' for ranks without gaps (GH6514)
Support passing encoding with xlwt (GH3710)
Refactor Block classes removing Block.items attributes to avoid duplication in item handling (GH6745, GH6988).
Testing statements updated to use specialized asserts (GH6175)
Performance¶
- Performance improvement when converting DatetimeIndex to floating ordinals using DatetimeConverter (GH6636)
- Performance improvement for DataFrame.shift (GH5609)
- Performance improvement in indexing into a multi-indexed Series (GH5567)
- Performance improvements in single-dtyped indexing (GH6484)
- Improve performance of DataFrame construction with certain offsets, by removing faulty caching (e.g. MonthEnd,BusinessMonthEnd), (GH6479)
- Improve performance of CustomBusinessDay (GH6584)
- improve performance of slice indexing on Series with string keys (GH6341, GH6372)
- Performance improvement for DataFrame.from_records when reading a specified number of rows from an iterable (GH6700)
- Performance improvements in timedelta conversions for integer dtypes (GH6754)
- Improved performance of compatible pickles (GH6899)
- Improve performance in certain reindexing operations by optimizing take_2d (GH6749)
- GroupBy.count() is now implemented in Cython and is much faster for large numbers of groups (GH7016).
Experimental¶
There are no experimental changes in 0.14.0
Bug Fixes¶
- Bug in Series ValueError when index doesn’t match data (GH6532)
- Prevent segfault due to MultiIndex not being supported in HDFStore table format (GH1848)
- Bug in pd.DataFrame.sort_index where mergesort wasn’t stable when ascending=False (GH6399)
- Bug in pd.tseries.frequencies.to_offset when argument has leading zeroes (GH6391)
- Bug in version string gen. for dev versions with shallow clones / install from tarball (GH6127)
- Inconsistent tz parsing Timestamp / to_datetime for current year (GH5958)
- Indexing bugs with reordered indexes (GH6252, GH6254)
- Bug in .xs with a Series multiindex (GH6258, GH5684)
- Bug in conversion of a string types to a DatetimeIndex with a specified frequency (GH6273, GH6274)
- Bug in eval where type-promotion failed for large expressions (GH6205)
- Bug in interpolate with inplace=True (GH6281)
- HDFStore.remove now handles start and stop (GH6177)
- HDFStore.select_as_multiple handles start and stop the same way as select (GH6177)
- HDFStore.select_as_coordinates and select_column works with a where clause that results in filters (GH6177)
- Regression in join of non_unique_indexes (GH6329)
- Issue with groupby agg with a single function and a a mixed-type frame (GH6337)
- Bug in DataFrame.replace() when passing a non- bool to_replace argument (GH6332)
- Raise when trying to align on different levels of a multi-index assignment (GH3738)
- Bug in setting complex dtypes via boolean indexing (GH6345)
- Bug in TimeGrouper/resample when presented with a non-monotonic DatetimeIndex that would return invalid results. (GH4161)
- Bug in index name propogation in TimeGrouper/resample (GH4161)
- TimeGrouper has a more compatible API to the rest of the groupers (e.g. groups was missing) (GH3881)
- Bug in multiple grouping with a TimeGrouper depending on target column order (GH6764)
- Bug in pd.eval when parsing strings with possible tokens like '&' (GH6351)
- Bug correctly handle placements of -inf in Panels when dividing by integer 0 (GH6178)
- DataFrame.shift with axis=1 was raising (GH6371)
- Disabled clipboard tests until release time (run locally with nosetests -A disabled) (GH6048).
- Bug in DataFrame.replace() when passing a nested dict that contained keys not in the values to be replaced (GH6342)
- str.match ignored the na flag (GH6609).
- Bug in take with duplicate columns that were not consolidated (GH6240)
- Bug in interpolate changing dtypes (GH6290)
- Bug in Series.get, was using a buggy access method (GH6383)
- Bug in hdfstore queries of the form where=[('date', '>=', datetime(2013,1,1)), ('date', '<=', datetime(2014,1,1))] (GH6313)
- Bug in DataFrame.dropna with duplicate indices (GH6355)
- Regression in chained getitem indexing with embedded list-like from 0.12 (GH6394)
- Float64Index with nans not comparing correctly (GH6401)
- eval/query expressions with strings containing the @ character will now work (GH6366).
- Bug in Series.reindex when specifying a method with some nan values was inconsistent (noted on a resample) (GH6418)
- Bug in DataFrame.replace() where nested dicts were erroneously depending on the order of dictionary keys and values (GH5338).
- Perf issue in concatting with empty objects (GH3259)
- Clarify sorting of sym_diff on Index objects with NaN values (GH6444)
- Regression in MultiIndex.from_product with a DatetimeIndex as input (GH6439)
- Bug in str.extract when passed a non-default index (GH6348)
- Bug in str.split when passed pat=None and n=1 (GH6466)
- Bug in io.data.DataReader when passed "F-F_Momentum_Factor" and data_source="famafrench" (GH6460)
- Bug in sum of a timedelta64[ns] series (GH6462)
- Bug in resample with a timezone and certain offsets (GH6397)
- Bug in iat/iloc with duplicate indices on a Series (GH6493)
- Bug in read_html where nan’s were incorrectly being used to indicate missing values in text. Should use the empty string for consistency with the rest of pandas (GH5129).
- Bug in read_html tests where redirected invalid URLs would make one test fail (GH6445).
- Bug in multi-axis indexing using .loc on non-unique indices (GH6504)
- Bug that caused _ref_locs corruption when slice indexing across columns axis of a DataFrame (GH6525)
- Regression from 0.13 in the treatment of numpy datetime64 non-ns dtypes in Series creation (GH6529)
- .names attribute of MultiIndexes passed to set_index are now preserved (GH6459).
- Bug in setitem with a duplicate index and an alignable rhs (GH6541)
- Bug in setitem with .loc on mixed integer Indexes (GH6546)
- Bug in pd.read_stata which would use the wrong data types and missing values (GH6327)
- Bug in DataFrame.to_stata that lead to data loss in certain cases, and could be exported using the wrong data types and missing values (GH6335)
- StataWriter replaces missing values in string columns by empty string (GH6802)
- Inconsistent types in Timestamp addition/subtraction (GH6543)
- Bug in preserving frequency across Timestamp addition/subtraction (GH4547)
- Bug in empty list lookup caused IndexError exceptions (GH6536, GH6551)
- Series.quantile raising on an object dtype (GH6555)
- Bug in .xs with a nan in level when dropped (GH6574)
- Bug in fillna with method='bfill/ffill' and datetime64[ns] dtype (GH6587)
- Bug in sql writing with mixed dtypes possibly leading to data loss (GH6509)
- Bug in Series.pop (GH6600)
- Bug in iloc indexing when positional indexer matched Int64Index of the corresponding axis and no reordering happened (GH6612)
- Bug in fillna with limit and value specified
- Bug in DataFrame.to_stata when columns have non-string names (GH4558)
- Bug in compat with np.compress, surfaced in (GH6658)
- Bug in binary operations with a rhs of a Series not aligning (GH6681)
- Bug in DataFrame.to_stata which incorrectly handles nan values and ignores with_index keyword argument (GH6685)
- Bug in resample with extra bins when using an evenly divisible frequency (GH4076)
- Bug in consistency of groupby aggregation when passing a custom function (GH6715)
- Bug in resample when how=None resample freq is the same as the axis frequency (GH5955)
- Bug in downcasting inference with empty arrays (GH6733)
- Bug in obj.blocks on sparse containers dropping all but the last items of same for dtype (GH6748)
- Bug in unpickling NaT (NaTType) (GH4606)
- Bug in DataFrame.replace() where regex metacharacters were being treated as regexs even when regex=False (GH6777).
- Bug in timedelta ops on 32-bit platforms (GH6808)
- Bug in setting a tz-aware index directly via .index (GH6785)
- Bug in expressions.py where numexpr would try to evaluate arithmetic ops (GH6762).
- Bug in Makefile where it didn’t remove Cython generated C files with make clean (GH6768)
- Bug with numpy < 1.7.2 when reading long strings from HDFStore (GH6166)
- Bug in DataFrame._reduce where non bool-like (0/1) integers were being coverted into bools. (GH6806)
- Regression from 0.13 with fillna and a Series on datetime-like (GH6344)
- Bug in adding np.timedelta64 to DatetimeIndex with timezone outputs incorrect results (GH6818)
- Bug in DataFrame.replace() where changing a dtype through replacement would only replace the first occurrence of a value (GH6689)
- Better error message when passing a frequency of ‘MS’ in Period construction (GH5332)
- Bug in Series.__unicode__ when max_rows=None and the Series has more than 1000 rows. (GH6863)
- Bug in groupby.get_group where a datetlike wasn’t always accepted (GH5267)
- Bug in groupBy.get_group created by TimeGrouper raises AttributeError (GH6914)
- Bug in DatetimeIndex.tz_localize and DatetimeIndex.tz_convert converting NaT incorrectly (GH5546)
- Bug in arithmetic operations affecting NaT (GH6873)
- Bug in Series.str.extract where the resulting Series from a single group match wasn’t renamed to the group name
- Bug in DataFrame.to_csv where setting index=False ignored the header kwarg (GH6186)
- Bug in DataFrame.plot and Series.plot, where the legend behave inconsistently when plotting to the same axes repeatedly (GH6678)
- Internal tests for patching __finalize__ / bug in merge not finalizing (GH6923, GH6927)
- accept TextFileReader in concat, which was affecting a common user idiom (GH6583)
- Bug in C parser with leading whitespace (GH3374)
- Bug in C parser with delim_whitespace=True and \r-delimited lines
- Bug in python parser with explicit multi-index in row following column header (GH6893)
- Bug in Series.rank and DataFrame.rank that caused small floats (<1e-13) to all receive the same rank (GH6886)
- Bug in DataFrame.apply with functions that used *args`` or **kwargs and returned an empty result (GH6952)
- Bug in sum/mean on 32-bit platforms on overflows (GH6915)
- Moved Panel.shift to NDFrame.slice_shift and fixed to respect multiple dtypes. (GH6959)
- Bug in enabling subplots=True in DataFrame.plot only has single column raises TypeError, and Series.plot raises AttributeError (GH6951)
- Bug in DataFrame.plot draws unnecessary axes when enabling subplots and kind=scatter (GH6951)
- Bug in read_csv from a filesystem with non-utf-8 encoding (GH6807)
- Bug in iloc when setting / aligning (GH6766)
- Bug causing UnicodeEncodeError when get_dummies called with unicode values and a prefix (GH6885)
- Bug in timeseries-with-frequency plot cursor display (GH5453)
- Bug surfaced in groupby.plot when using a Float64Index (GH7025)
- Stopped tests from failing if options data isn’t able to be downloaded from Yahoo (GH7034)
- Bug in parallel_coordinates and radviz where reordering of class column caused possible color/class mismatch (GH6956)
- Bug in radviz and andrews_curves where multiple values of ‘color’ were being passed to plotting method (GH6956)
- Bug in Float64Index.isin() where containing nan s would make indices claim that they contained all the things (GH7066).
- Bug in DataFrame.boxplot where it failed to use the axis passed as the ax argument (GH3578)
- Bug in the XlsxWriter and XlwtWriter implementations that resulted in datetime columns being formatted without the time (GH7075) were being passed to plotting method
- read_fwf() treats None in colspec like regular python slices. It now reads from the beginning or until the end of the line when colspec contains a None (previously raised a TypeError)
- Bug in cache coherence with chained indexing and slicing; add _is_view property to NDFrame to correctly predict views; mark is_copy on xs only if its an actual copy (and not a view) (GH7084)
- Bug in DatetimeIndex creation from string ndarray with dayfirst=True (GH5917)
- Bug in MultiIndex.from_arrays created from DatetimeIndex doesn’t preserve freq and tz (GH7090)
- Bug in unstack raises ValueError when MultiIndex contains PeriodIndex (GH4342)
- Bug in boxplot and hist draws unnecessary axes (GH6769)
- Regression in groupby.nth() for out-of-bounds indexers (GH6621)
- Bug in quantile with datetime values (GH6965)
- Bug in Dataframe.set_index, reindex and pivot don’t preserve DatetimeIndex and PeriodIndex attributes (GH3950, GH5878, GH6631)
- Bug in MultiIndex.get_level_values doesn’t preserve DatetimeIndex and PeriodIndex attributes (GH7092)
- Bug in Groupby doesn’t preserve tz (GH3950)
- Bug in PeriodIndex partial string slicing (GH6716)
- Bug in the HTML repr of a truncated Series or DataFrame not showing the class name with the large_repr set to ‘info’ (GH7105)
- Bug in DatetimeIndex specifying freq raises ValueError when passed value is too short (GH7098)
- Fixed a bug with the info repr not honoring the display.max_info_columns setting (GH6939)
- Bug PeriodIndex string slicing with out of bounds values (GH5407)
- Fixed a memory error in the hashtable implementation/factorizer on resizing of large tables (GH7157)
- Bug in isnull when applied to 0-dimensional object arrays (GH7176)
- Bug in query/eval where global constants were not looked up correctly (GH7178)
- Bug in recognizing out-of-bounds positional list indexers with iloc and a multi-axis tuple indexer (GH7189)
- Bug in setitem with a single value, multi-index and integer indices (GH7190, GH7218)
- Bug in expressions evaluation with reversed ops, showing in series-dataframe ops (GH7198, GH7192)
- Bug in multi-axis indexing with > 2 ndim and a multi-index (GH7199)
- Fix a bug where invalid eval/query operations would blow the stack (GH5198)
v0.13.1 (February 3, 2014)¶
This is a minor release from 0.13.0 and includes a small number of API changes, several new features, enhancements, and performance improvements along with a large number of bug fixes. We recommend that all users upgrade to this version.
Highlights include:
- Added infer_datetime_format keyword to read_csv/to_datetime to allow speedups for homogeneously formatted datetimes.
- Will intelligently limit display precision for datetime/timedelta formats.
- Enhanced Panel apply() method.
- Suggested tutorials in new Tutorials section.
- Our pandas ecosystem is growing, We now feature related projects in a new Pandas Ecosystem section.
- Much work has been taking place on improving the docs, and a new Contributing section has been added.
- Even though it may only be of interest to devs, we <3 our new CI status page: ScatterCI.
Warning
0.13.1 fixes a bug that was caused by a combination of having numpy < 1.8, and doing chained assignment on a string-like array. Please review the docs, chained indexing can have unexpected results and should generally be avoided.
This would previously segfault:
In [1]: df = DataFrame(dict(A = np.array(['foo','bar','bah','foo','bar'])))
In [2]: df['A'].iloc[0] = np.nan
In [3]: df
Out[3]:
A
0 NaN
1 bar
2 bah
3 foo
4 bar
The recommended way to do this type of assignment is:
In [4]: df = DataFrame(dict(A = np.array(['foo','bar','bah','foo','bar'])))
In [5]: df.ix[0,'A'] = np.nan
In [6]: df
Out[6]:
A
0 NaN
1 bar
2 bah
3 foo
4 bar
Output Formatting Enhancements¶
df.info() view now display dtype info per column (GH5682)
df.info() now honors the option max_info_rows, to disable null counts for large frames (GH5974)
In [7]: max_info_rows = pd.get_option('max_info_rows') In [8]: df = DataFrame(dict(A = np.random.randn(10), ...: B = np.random.randn(10), ...: C = date_range('20130101',periods=10))) ...: In [9]: df.iloc[3:6,[0,2]] = np.nan
# set to not display the null counts In [10]: pd.set_option('max_info_rows',0) In [11]: df.info() <class 'pandas.core.frame.DataFrame'> Int64Index: 10 entries, 0 to 9 Data columns (total 3 columns): A float64 B float64 C datetime64[ns] dtypes: datetime64[ns](1), float64(2) memory usage: 320.0 bytes
# this is the default (same as in 0.13.0) In [12]: pd.set_option('max_info_rows',max_info_rows) In [13]: df.info() <class 'pandas.core.frame.DataFrame'> Int64Index: 10 entries, 0 to 9 Data columns (total 3 columns): A 7 non-null float64 B 10 non-null float64 C 7 non-null datetime64[ns] dtypes: datetime64[ns](1), float64(2) memory usage: 320.0 bytes
Add show_dimensions display option for the new DataFrame repr to control whether the dimensions print.
In [14]: df = DataFrame([[1, 2], [3, 4]]) In [15]: pd.set_option('show_dimensions', False) In [16]: df Out[16]: 0 1 0 1 2 1 3 4 In [17]: pd.set_option('show_dimensions', True) In [18]: df Out[18]: 0 1 0 1 2 1 3 4 [2 rows x 2 columns]
The ArrayFormatter for datetime and timedelta64 now intelligently limit precision based on the values in the array (GH3401)
Previously output might look like:
age today diff 0 2001-01-01 00:00:00 2013-04-19 00:00:00 4491 days, 00:00:00 1 2004-06-01 00:00:00 2013-04-19 00:00:00 3244 days, 00:00:00
Now the output looks like:
In [19]: df = DataFrame([ Timestamp('20010101'), ....: Timestamp('20040601') ], columns=['age']) ....: In [20]: df['today'] = Timestamp('20130419') In [21]: df['diff'] = df['today']-df['age'] In [22]: df Out[22]: age today diff 0 2001-01-01 2013-04-19 4491 days 1 2004-06-01 2013-04-19 3244 days [2 rows x 3 columns]
API changes¶
Add -NaN and -nan to the default set of NA values (GH5952). See NA Values.
Added Series.str.get_dummies vectorized string method (GH6021), to extract dummy/indicator variables for separated string columns:
In [23]: s = Series(['a', 'a|b', np.nan, 'a|c']) In [24]: s.str.get_dummies(sep='|') Out[24]: a b c 0 1 0 0 1 1 1 0 2 0 0 0 3 1 0 1 [4 rows x 3 columns]
Added the NDFrame.equals() method to compare if two NDFrames are equal have equal axes, dtypes, and values. Added the array_equivalent function to compare if two ndarrays are equal. NaNs in identical locations are treated as equal. (GH5283) See also the docs for a motivating example.
In [25]: df = DataFrame({'col':['foo', 0, np.nan]}) In [26]: df2 = DataFrame({'col':[np.nan, 0, 'foo']}, index=[2,1,0]) In [27]: df.equals(df2) Out[27]: False In [28]: df.equals(df2.sort()) Out[28]: True In [29]: import pandas.core.common as com In [30]: com.array_equivalent(np.array([0, np.nan]), np.array([0, np.nan])) Out[30]: True In [31]: np.array_equal(np.array([0, np.nan]), np.array([0, np.nan])) Out[31]: False
DataFrame.apply will use the reduce argument to determine whether a Series or a DataFrame should be returned when the DataFrame is empty (GH6007).
Previously, calling DataFrame.apply an empty DataFrame would return either a DataFrame if there were no columns, or the function being applied would be called with an empty Series to guess whether a Series or DataFrame should be returned:
In [32]: def applied_func(col): ....: print("Apply function being called with: ", col) ....: return col.sum() ....: In [33]: empty = DataFrame(columns=['a', 'b']) In [34]: empty.apply(applied_func) ('Apply function being called with: ', Series([], dtype: float64)) Out[34]: a NaN b NaN dtype: float64
Now, when apply is called on an empty DataFrame: if the reduce argument is True a Series will returned, if it is False a DataFrame will be returned, and if it is None (the default) the function being applied will be called with an empty series to try and guess the return type.
In [35]: empty.apply(applied_func, reduce=True) Out[35]: a NaN b NaN dtype: float64 In [36]: empty.apply(applied_func, reduce=False) Out[36]: Empty DataFrame Columns: [a, b] Index: [] [0 rows x 2 columns]
Prior Version Deprecations/Changes¶
There are no announced changes in 0.13 or prior that are taking effect as of 0.13.1
Deprecations¶
There are no deprecations of prior behavior in 0.13.1
Enhancements¶
pd.read_csv and pd.to_datetime learned a new infer_datetime_format keyword which greatly improves parsing perf in many cases. Thanks to @lexual for suggesting and @danbirken for rapidly implementing. (GH5490, GH6021)
If parse_dates is enabled and this flag is set, pandas will attempt to infer the format of the datetime strings in the columns, and if it can be inferred, switch to a faster method of parsing them. In some cases this can increase the parsing speed by ~5-10x.
# Try to infer the format for the index column df = pd.read_csv('foo.csv', index_col=0, parse_dates=True, infer_datetime_format=True)
date_format and datetime_format keywords can now be specified when writing to excel files (GH4133)
MultiIndex.from_product convenience function for creating a MultiIndex from the cartesian product of a set of iterables (GH6055):
In [37]: shades = ['light', 'dark'] In [38]: colors = ['red', 'green', 'blue'] In [39]: MultiIndex.from_product([shades, colors], names=['shade', 'color']) Out[39]: MultiIndex(levels=[[u'dark', u'light'], [u'blue', u'green', u'red']], labels=[[1, 1, 1, 0, 0, 0], [2, 1, 0, 2, 1, 0]], names=[u'shade', u'color'])
Panel apply() will work on non-ufuncs. See the docs.
In [40]: import pandas.util.testing as tm In [41]: panel = tm.makePanel(5) In [42]: panel Out[42]: <class 'pandas.core.panel.Panel'> Dimensions: 3 (items) x 5 (major_axis) x 4 (minor_axis) Items axis: ItemA to ItemC Major_axis axis: 2000-01-03 00:00:00 to 2000-01-07 00:00:00 Minor_axis axis: A to D In [43]: panel['ItemA'] Out[43]: A B C D 2000-01-03 0.952478 -1.239072 -1.409432 -0.014752 2000-01-04 0.988138 0.139683 1.422986 1.272395 2000-01-05 -0.072608 -0.223019 -2.147855 -1.449567 2000-01-06 -0.550603 2.123692 -1.347533 -1.195524 2000-01-07 -0.938153 0.122273 0.363565 -0.591863 [5 rows x 4 columns]
Specifying an apply that operates on a Series (to return a single element)
In [44]: panel.apply(lambda x: x.dtype, axis='items') Out[44]: A B C D 2000-01-03 float64 float64 float64 float64 2000-01-04 float64 float64 float64 float64 2000-01-05 float64 float64 float64 float64 2000-01-06 float64 float64 float64 float64 2000-01-07 float64 float64 float64 float64 [5 rows x 4 columns]
A similar reduction type operation
In [45]: panel.apply(lambda x: x.sum(), axis='major_axis') Out[45]: ItemA ItemB ItemC A 0.379252 -3.696907 3.709335 B 0.923558 0.504242 4.656781 C -3.118269 -1.545718 3.188329 D -1.979310 -0.758060 -1.436483 [4 rows x 3 columns]
This is equivalent to
In [46]: panel.sum('major_axis') Out[46]: ItemA ItemB ItemC A 0.379252 -3.696907 3.709335 B 0.923558 0.504242 4.656781 C -3.118269 -1.545718 3.188329 D -1.979310 -0.758060 -1.436483 [4 rows x 3 columns]
A transformation operation that returns a Panel, but is computing the z-score across the major_axis
In [47]: result = panel.apply( ....: lambda x: (x-x.mean())/x.std(), ....: axis='major_axis') ....: In [48]: result Out[48]: <class 'pandas.core.panel.Panel'> Dimensions: 3 (items) x 5 (major_axis) x 4 (minor_axis) Items axis: ItemA to ItemC Major_axis axis: 2000-01-03 00:00:00 to 2000-01-07 00:00:00 Minor_axis axis: A to D In [49]: result['ItemA'] Out[49]: A B C D 2000-01-03 1.004994 -1.166509 -0.535027 0.350970 2000-01-04 1.045875 -0.036892 1.393532 1.536326 2000-01-05 -0.170198 -0.334055 -1.037810 -0.970374 2000-01-06 -0.718186 1.588611 -0.492880 -0.736422 2000-01-07 -1.162486 -0.051156 0.672185 -0.180500 [5 rows x 4 columns]
Panel apply() operating on cross-sectional slabs. (GH1148)
In [50]: f = lambda x: ((x.T-x.mean(1))/x.std(1)).T In [51]: result = panel.apply(f, axis = ['items','major_axis']) In [52]: result Out[52]: <class 'pandas.core.panel.Panel'> Dimensions: 4 (items) x 5 (major_axis) x 3 (minor_axis) Items axis: A to D Major_axis axis: 2000-01-03 00:00:00 to 2000-01-07 00:00:00 Minor_axis axis: ItemA to ItemC In [53]: result.loc[:,:,'ItemA'] Out[53]: A B C D 2000-01-03 0.116579 -0.667845 -1.151538 -0.157547 2000-01-04 0.650448 -1.114910 0.841527 0.760706 2000-01-05 -0.987433 -0.438897 -1.154468 -0.015033 2000-01-06 0.494000 1.060450 -0.775993 -1.140165 2000-01-07 -0.363770 0.013169 0.392036 -1.123913 [5 rows x 4 columns]
This is equivalent to the following
In [54]: result = Panel(dict([ (ax,f(panel.loc[:,:,ax])) ....: for ax in panel.minor_axis ])) ....: In [55]: result Out[55]: <class 'pandas.core.panel.Panel'> Dimensions: 4 (items) x 5 (major_axis) x 3 (minor_axis) Items axis: A to D Major_axis axis: 2000-01-03 00:00:00 to 2000-01-07 00:00:00 Minor_axis axis: ItemA to ItemC In [56]: result.loc[:,:,'ItemA'] Out[56]: A B C D 2000-01-03 0.116579 -0.667845 -1.151538 -0.157547 2000-01-04 0.650448 -1.114910 0.841527 0.760706 2000-01-05 -0.987433 -0.438897 -1.154468 -0.015033 2000-01-06 0.494000 1.060450 -0.775993 -1.140165 2000-01-07 -0.363770 0.013169 0.392036 -1.123913 [5 rows x 4 columns]
Performance¶
Performance improvements for 0.13.1
- Series datetime/timedelta binary operations (GH5801)
- DataFrame count/dropna for axis=1
- Series.str.contains now has a regex=False keyword which can be faster for plain (non-regex) string patterns. (GH5879)
- Series.str.extract (GH5944)
- dtypes/ftypes methods (GH5968)
- indexing with object dtypes (GH5968)
- DataFrame.apply (GH6013)
- Regression in JSON IO (GH5765)
- Index construction from Series (GH6150)
Experimental¶
There are no experimental changes in 0.13.1
Bug Fixes¶
See V0.13.1 Bug Fixes for an extensive list of bugs that have been fixed in 0.13.1.
See the full release notes or issue tracker on GitHub for a complete list of all API changes, Enhancements and Bug Fixes.
v0.13.0 (January 3, 2014)¶
This is a major release from 0.12.0 and includes a number of API changes, several new features and enhancements along with a large number of bug fixes.
Highlights include:
- support for a new index type Float64Index, and other Indexing enhancements
- HDFStore has a new string based syntax for query specification
- support for new methods of interpolation
- updated timedelta operations
- a new string manipulation method extract
- Nanosecond support for Offsets
- isin for DataFrames
Several experimental features are added, including:
- new eval/query methods for expression evaluation
- support for msgpack serialization
- an i/o interface to Google’s BigQuery
Their are several new or updated docs sections including:
- Comparison with SQL, which should be useful for those familiar with SQL but still learning pandas.
- Comparison with R, idiom translations from R to pandas.
- Enhancing Performance, ways to enhance pandas performance with eval/query.
Warning
In 0.13.0 Series has internally been refactored to no longer sub-class ndarray but instead subclass NDFrame, similar to the rest of the pandas containers. This should be a transparent change with only very limited API implications. See Internal Refactoring
API changes¶
read_excel now supports an integer in its sheetname argument giving the index of the sheet to read in (GH4301).
Text parser now treats anything that reads like inf (“inf”, “Inf”, “-Inf”, “iNf”, etc.) as infinity. (GH4220, GH4219), affecting read_table, read_csv, etc.
pandas now is Python 2/3 compatible without the need for 2to3 thanks to @jtratner. As a result, pandas now uses iterators more extensively. This also led to the introduction of substantive parts of the Benjamin Peterson’s six library into compat. (GH4384, GH4375, GH4372)
pandas.util.compat and pandas.util.py3compat have been merged into pandas.compat. pandas.compat now includes many functions allowing 2/3 compatibility. It contains both list and iterator versions of range, filter, map and zip, plus other necessary elements for Python 3 compatibility. lmap, lzip, lrange and lfilter all produce lists instead of iterators, for compatibility with numpy, subscripting and pandas constructors.(GH4384, GH4375, GH4372)
Series.get with negative indexers now returns the same as [] (GH4390)
Changes to how Index and MultiIndex handle metadata (levels, labels, and names) (GH4039):
# previously, you would have set levels or labels directly index.levels = [[1, 2, 3, 4], [1, 2, 4, 4]] # now, you use the set_levels or set_labels methods index = index.set_levels([[1, 2, 3, 4], [1, 2, 4, 4]]) # similarly, for names, you can rename the object # but setting names is not deprecated index = index.set_names(["bob", "cranberry"]) # and all methods take an inplace kwarg - but return None index.set_names(["bob", "cranberry"], inplace=True)
All division with NDFrame objects is now truedivision, regardless of the future import. This means that operating on pandas objects will by default use floating point division, and return a floating point dtype. You can use // and floordiv to do integer division.
Integer division
In [3]: arr = np.array([1, 2, 3, 4]) In [4]: arr2 = np.array([5, 3, 2, 1]) In [5]: arr / arr2 Out[5]: array([0, 0, 1, 4]) In [6]: Series(arr) // Series(arr2) Out[6]: 0 0 1 0 2 1 3 4 dtype: int64
True Division
In [7]: pd.Series(arr) / pd.Series(arr2) # no future import required Out[7]: 0 0.200000 1 0.666667 2 1.500000 3 4.000000 dtype: float64
Infer and downcast dtype if downcast='infer' is passed to fillna/ffill/bfill (GH4604)
__nonzero__ for all NDFrame objects, will now raise a ValueError, this reverts back to (GH1073, GH4633) behavior. See gotchas for a more detailed discussion.
This prevents doing boolean comparison on entire pandas objects, which is inherently ambiguous. These all will raise a ValueError.
if df: .... df1 and df2 s1 and s2
Added the .bool() method to NDFrame objects to facilitate evaluating of single-element boolean Series:
In [1]: Series([True]).bool() Out[1]: True In [2]: Series([False]).bool() Out[2]: False In [3]: DataFrame([[True]]).bool() Out[3]: True In [4]: DataFrame([[False]]).bool() Out[4]: False
All non-Index NDFrames (Series, DataFrame, Panel, Panel4D, SparsePanel, etc.), now support the entire set of arithmetic operators and arithmetic flex methods (add, sub, mul, etc.). SparsePanel does not support pow or mod with non-scalars. (GH3765)
Series and DataFrame now have a mode() method to calculate the statistical mode(s) by axis/Series. (GH5367)
Chained assignment will now by default warn if the user is assigning to a copy. This can be changed with the option mode.chained_assignment, allowed options are raise/warn/None. See the docs.
In [5]: dfc = DataFrame({'A':['aaa','bbb','ccc'],'B':[1,2,3]}) In [6]: pd.set_option('chained_assignment','warn')
The following warning / exception will show if this is attempted.
In [7]: dfc.loc[0]['A'] = 1111
Traceback (most recent call last) ... SettingWithCopyWarning: A value is trying to be set on a copy of a slice from a DataFrame. Try using .loc[row_index,col_indexer] = value instead
Here is the correct method of assignment.
In [8]: dfc.loc[0,'A'] = 11 In [9]: dfc Out[9]: A B 0 11 1 1 bbb 2 2 ccc 3 [3 rows x 2 columns]
- Panel.reindex has the following call signature Panel.reindex(items=None, major_axis=None, minor_axis=None, **kwargs)
to conform with other NDFrame objects. See Internal Refactoring for more information.
- Series.argmin and Series.argmax are now aliased to Series.idxmin and Series.idxmax. These return the index of the
min or max element respectively. Prior to 0.13.0 these would return the position of the min / max element. (GH6214)
Prior Version Deprecations/Changes¶
These were announced changes in 0.12 or prior that are taking effect as of 0.13.0
- Remove deprecated Factor (GH3650)
- Remove deprecated set_printoptions/reset_printoptions (GH3046)
- Remove deprecated _verbose_info (GH3215)
- Remove deprecated read_clipboard/to_clipboard/ExcelFile/ExcelWriter from pandas.io.parsers (GH3717) These are available as functions in the main pandas namespace (e.g. pd.read_clipboard)
- default for tupleize_cols is now False for both to_csv and read_csv. Fair warning in 0.12 (GH3604)
- default for display.max_seq_len is now 100 rather then None. This activates truncated display (”...”) of long sequences in various places. (GH3391)
Deprecations¶
Deprecated in 0.13.0
- deprecated iterkv, which will be removed in a future release (this was an alias of iteritems used to bypass 2to3‘s changes). (GH4384, GH4375, GH4372)
- deprecated the string method match, whose role is now performed more idiomatically by extract. In a future release, the default behavior of match will change to become analogous to contains, which returns a boolean indexer. (Their distinction is strictness: match relies on re.match while contains relies on re.search.) In this release, the deprecated behavior is the default, but the new behavior is available through the keyword argument as_indexer=True.
Indexing API Changes¶
Prior to 0.13, it was impossible to use a label indexer (.loc/.ix) to set a value that was not contained in the index of a particular axis. (GH2578). See the docs
In the Series case this is effectively an appending operation
In [10]: s = Series([1,2,3])
In [11]: s
Out[11]:
0 1
1 2
2 3
dtype: int64
In [12]: s[5] = 5.
In [13]: s
Out[13]:
0 1
1 2
2 3
5 5
dtype: float64
In [14]: dfi = DataFrame(np.arange(6).reshape(3,2),
....: columns=['A','B'])
....:
In [15]: dfi
Out[15]:
A B
0 0 1
1 2 3
2 4 5
[3 rows x 2 columns]
This would previously KeyError
In [16]: dfi.loc[:,'C'] = dfi.loc[:,'A']
In [17]: dfi
Out[17]:
A B C
0 0 1 0
1 2 3 2
2 4 5 4
[3 rows x 3 columns]
This is like an append operation.
In [18]: dfi.loc[3] = 5
In [19]: dfi
Out[19]:
A B C
0 0 1 0
1 2 3 2
2 4 5 4
3 5 5 5
[4 rows x 3 columns]
A Panel setting operation on an arbitrary axis aligns the input to the Panel
In [20]: p = pd.Panel(np.arange(16).reshape(2,4,2),
....: items=['Item1','Item2'],
....: major_axis=pd.date_range('2001/1/12',periods=4),
....: minor_axis=['A','B'],dtype='float64')
....:
In [21]: p
Out[21]:
<class 'pandas.core.panel.Panel'>
Dimensions: 2 (items) x 4 (major_axis) x 2 (minor_axis)
Items axis: Item1 to Item2
Major_axis axis: 2001-01-12 00:00:00 to 2001-01-15 00:00:00
Minor_axis axis: A to B
In [22]: p.loc[:,:,'C'] = Series([30,32],index=p.items)
In [23]: p
Out[23]:
<class 'pandas.core.panel.Panel'>
Dimensions: 2 (items) x 4 (major_axis) x 3 (minor_axis)
Items axis: Item1 to Item2
Major_axis axis: 2001-01-12 00:00:00 to 2001-01-15 00:00:00
Minor_axis axis: A to C
In [24]: p.loc[:,:,'C']
Out[24]:
Item1 Item2
2001-01-12 30 32
2001-01-13 30 32
2001-01-14 30 32
2001-01-15 30 32
[4 rows x 2 columns]
Float64Index API Change¶
Added a new index type, Float64Index. This will be automatically created when passing floating values in index creation. This enables a pure label-based slicing paradigm that makes [],ix,loc for scalar indexing and slicing work exactly the same. See the docs, (GH263)
Construction is by default for floating type values.
In [25]: index = Index([1.5, 2, 3, 4.5, 5]) In [26]: index Out[26]: Float64Index([1.5, 2.0, 3.0, 4.5, 5.0], dtype='float64') In [27]: s = Series(range(5),index=index) In [28]: s Out[28]: 1.5 0 2.0 1 3.0 2 4.5 3 5.0 4 dtype: int64
Scalar selection for [],.ix,.loc will always be label based. An integer will match an equal float index (e.g. 3 is equivalent to 3.0)
In [29]: s[3] Out[29]: 2 In [30]: s.ix[3] Out[30]: 2 In [31]: s.loc[3] Out[31]: 2
The only positional indexing is via iloc
In [32]: s.iloc[3] Out[32]: 3
A scalar index that is not found will raise KeyError
Slicing is ALWAYS on the values of the index, for [],ix,loc and ALWAYS positional with iloc
In [33]: s[2:4] Out[33]: 2 1 3 2 dtype: int64 In [34]: s.ix[2:4] Out[34]: 2 1 3 2 dtype: int64 In [35]: s.loc[2:4] Out[35]: 2 1 3 2 dtype: int64 In [36]: s.iloc[2:4] Out[36]: 3.0 2 4.5 3 dtype: int64
In float indexes, slicing using floats are allowed
In [37]: s[2.1:4.6] Out[37]: 3.0 2 4.5 3 dtype: int64 In [38]: s.loc[2.1:4.6] Out[38]: 3.0 2 4.5 3 dtype: int64
Indexing on other index types are preserved (and positional fallback for [],ix), with the exception, that floating point slicing on indexes on non Float64Index will now raise a TypeError.
In [1]: Series(range(5))[3.5] TypeError: the label [3.5] is not a proper indexer for this index type (Int64Index) In [1]: Series(range(5))[3.5:4.5] TypeError: the slice start [3.5] is not a proper indexer for this index type (Int64Index)
Using a scalar float indexer will be deprecated in a future version, but is allowed for now.
In [3]: Series(range(5))[3.0] Out[3]: 3
HDFStore API Changes¶
Query Format Changes. A much more string-like query format is now supported. See the docs.
In [39]: path = 'test.h5' In [40]: dfq = DataFrame(randn(10,4), ....: columns=list('ABCD'), ....: index=date_range('20130101',periods=10)) ....: In [41]: dfq.to_hdf(path,'dfq',format='table',data_columns=True)
Use boolean expressions, with in-line function evaluation.
In [42]: read_hdf(path,'dfq', ....: where="index>Timestamp('20130104') & columns=['A', 'B']") ....: Out[42]: A B 2013-01-05 -1.392054 1.153922 2013-01-06 -0.881047 0.295080 2013-01-07 -1.407085 0.126781 2013-01-08 -0.838843 0.553921 2013-01-09 1.529401 0.205455 2013-01-10 0.299071 1.076541 [6 rows x 2 columns]
Use an inline column reference
In [43]: read_hdf(path,'dfq', ....: where="A>0 or C>0") ....: Out[43]: A B C D 2013-01-01 1.126386 0.247112 0.121172 0.298984 2013-01-03 0.581073 2.763844 0.399325 0.668488 2013-01-04 -0.275774 0.500483 0.863065 -1.051628 2013-01-05 -1.392054 1.153922 1.181944 0.391371 2013-01-06 -0.881047 0.295080 1.863801 -1.712274 2013-01-07 -1.407085 0.126781 0.003760 -1.268994 2013-01-09 1.529401 0.205455 0.313013 0.866521 2013-01-10 0.299071 1.076541 0.363177 1.893680 [8 rows x 4 columns]
the format keyword now replaces the table keyword; allowed values are fixed(f) or table(t) the same defaults as prior < 0.13.0 remain, e.g. put implies fixed format and append implies table format. This default format can be set as an option by setting io.hdf.default_format.
In [44]: path = 'test.h5' In [45]: df = DataFrame(randn(10,2)) In [46]: df.to_hdf(path,'df_table',format='table') In [47]: df.to_hdf(path,'df_table2',append=True) In [48]: df.to_hdf(path,'df_fixed') In [49]: with get_store(path) as store: ....: print(store) ....: <class 'pandas.io.pytables.HDFStore'> File path: test.h5 /df_fixed frame (shape->[10,2]) /df_table frame_table (typ->appendable,nrows->10,ncols->2,indexers->[index]) /df_table2 frame_table (typ->appendable,nrows->10,ncols->2,indexers->[index])
Significant table writing performance improvements
handle a passed Series in table format (GH4330)
can now serialize a timedelta64[ns] dtype in a table (GH3577), See the docs.
added an is_open property to indicate if the underlying file handle is_open; a closed store will now report ‘CLOSED’ when viewing the store (rather than raising an error) (GH4409)
a close of a HDFStore now will close that instance of the HDFStore but will only close the actual file if the ref count (by PyTables) w.r.t. all of the open handles are 0. Essentially you have a local instance of HDFStore referenced by a variable. Once you close it, it will report closed. Other references (to the same file) will continue to operate until they themselves are closed. Performing an action on a closed file will raise ClosedFileError
In [50]: path = 'test.h5' In [51]: df = DataFrame(randn(10,2)) In [52]: store1 = HDFStore(path) In [53]: store2 = HDFStore(path) In [54]: store1.append('df',df) In [55]: store2.append('df2',df) In [56]: store1 Out[56]: <class 'pandas.io.pytables.HDFStore'> File path: test.h5 /df frame_table (typ->appendable,nrows->10,ncols->2,indexers->[index]) In [57]: store2 Out[57]: <class 'pandas.io.pytables.HDFStore'> File path: test.h5 /df frame_table (typ->appendable,nrows->10,ncols->2,indexers->[index]) /df2 frame_table (typ->appendable,nrows->10,ncols->2,indexers->[index]) In [58]: store1.close() In [59]: store2 Out[59]: <class 'pandas.io.pytables.HDFStore'> File path: test.h5 /df frame_table (typ->appendable,nrows->10,ncols->2,indexers->[index]) /df2 frame_table (typ->appendable,nrows->10,ncols->2,indexers->[index]) In [60]: store2.close() In [61]: store2 Out[61]: <class 'pandas.io.pytables.HDFStore'> File path: test.h5 File is CLOSED
removed the _quiet attribute, replace by a DuplicateWarning if retrieving duplicate rows from a table (GH4367)
removed the warn argument from open. Instead a PossibleDataLossError exception will be raised if you try to use mode='w' with an OPEN file handle (GH4367)
allow a passed locations array or mask as a where condition (GH4467). See the docs for an example.
add the keyword dropna=True to append to change whether ALL nan rows are not written to the store (default is True, ALL nan rows are NOT written), also settable via the option io.hdf.dropna_table (GH4625)
pass thru store creation arguments; can be used to support in-memory stores
DataFrame repr Changes¶
The HTML and plain text representations of DataFrame now show a truncated view of the table once it exceeds a certain size, rather than switching to the short info view (GH4886, GH5550). This makes the representation more consistent as small DataFrames get larger.
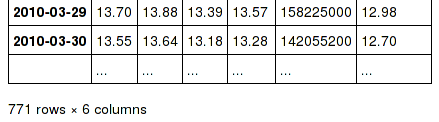
To get the info view, call DataFrame.info(). If you prefer the info view as the repr for large DataFrames, you can set this by running set_option('display.large_repr', 'info').
Enhancements¶
df.to_clipboard() learned a new excel keyword that let’s you paste df data directly into excel (enabled by default). (GH5070).
read_html now raises a URLError instead of catching and raising a ValueError (GH4303, GH4305)
Added a test for read_clipboard() and to_clipboard() (GH4282)
Clipboard functionality now works with PySide (GH4282)
Added a more informative error message when plot arguments contain overlapping color and style arguments (GH4402)
to_dict now takes records as a possible outtype. Returns an array of column-keyed dictionaries. (GH4936)
NaN handing in get_dummies (GH4446) with dummy_na
# previously, nan was erroneously counted as 2 here # now it is not counted at all In [62]: get_dummies([1, 2, np.nan]) Out[62]: 1 2 0 1 0 1 0 1 2 0 0 [3 rows x 2 columns] # unless requested In [63]: get_dummies([1, 2, np.nan], dummy_na=True) Out[63]: 1 2 NaN 0 1 0 0 1 0 1 0 2 0 0 1 [3 rows x 3 columns]
timedelta64[ns] operations. See the docs.
Warning
Most of these operations require numpy >= 1.7
Using the new top-level to_timedelta, you can convert a scalar or array from the standard timedelta format (produced by to_csv) into a timedelta type (np.timedelta64 in nanoseconds).
In [64]: to_timedelta('1 days 06:05:01.00003') Out[64]: Timedelta('1 days 06:05:01.000030') In [65]: to_timedelta('15.5us') Out[65]: Timedelta('0 days 00:00:00.000015') In [66]: to_timedelta(['1 days 06:05:01.00003','15.5us','nan']) Out[66]: TimedeltaIndex(['1 days 06:05:01.000030', '0 days 00:00:00.000015', NaT], dtype='timedelta64[ns]', freq=None) In [67]: to_timedelta(np.arange(5),unit='s') Out[67]: TimedeltaIndex(['00:00:00', '00:00:01', '00:00:02', '00:00:03', '00:00:04'], dtype='timedelta64[ns]', freq=None) In [68]: to_timedelta(np.arange(5),unit='d') Out[68]: TimedeltaIndex(['0 days', '1 days', '2 days', '3 days', '4 days'], dtype='timedelta64[ns]', freq=None)
A Series of dtype timedelta64[ns] can now be divided by another timedelta64[ns] object, or astyped to yield a float64 dtyped Series. This is frequency conversion. See the docs for the docs.
In [69]: from datetime import timedelta In [70]: td = Series(date_range('20130101',periods=4))-Series(date_range('20121201',periods=4)) In [71]: td[2] += np.timedelta64(timedelta(minutes=5,seconds=3)) In [72]: td[3] = np.nan In [73]: td Out[73]: 0 31 days 00:00:00 1 31 days 00:00:00 2 31 days 00:05:03 3 NaT dtype: timedelta64[ns] # to days In [74]: td / np.timedelta64(1,'D') Out[74]: 0 31.000000 1 31.000000 2 31.003507 3 NaN dtype: float64 In [75]: td.astype('timedelta64[D]') Out[75]: 0 31 1 31 2 31 3 NaN dtype: float64 # to seconds In [76]: td / np.timedelta64(1,'s') Out[76]: 0 2678400 1 2678400 2 2678703 3 NaN dtype: float64 In [77]: td.astype('timedelta64[s]') Out[77]: 0 2678400 1 2678400 2 2678703 3 NaN dtype: float64
Dividing or multiplying a timedelta64[ns] Series by an integer or integer Series
In [78]: td * -1 Out[78]: 0 -31 days +00:00:00 1 -31 days +00:00:00 2 -32 days +23:54:57 3 NaT dtype: timedelta64[ns] In [79]: td * Series([1,2,3,4]) Out[79]: 0 31 days 00:00:00 1 62 days 00:00:00 2 93 days 00:15:09 3 NaT dtype: timedelta64[ns]
Absolute DateOffset objects can act equivalently to timedeltas
In [80]: from pandas import offsets In [81]: td + offsets.Minute(5) + offsets.Milli(5) Out[81]: 0 31 days 00:05:00.005000 1 31 days 00:05:00.005000 2 31 days 00:10:03.005000 3 NaT dtype: timedelta64[ns]
Fillna is now supported for timedeltas
In [82]: td.fillna(0) Out[82]: 0 31 days 00:00:00 1 31 days 00:00:00 2 31 days 00:05:03 3 0 days 00:00:00 dtype: timedelta64[ns] In [83]: td.fillna(timedelta(days=1,seconds=5)) Out[83]: 0 31 days 00:00:00 1 31 days 00:00:00 2 31 days 00:05:03 3 1 days 00:00:05 dtype: timedelta64[ns]
You can do numeric reduction operations on timedeltas.
In [84]: td.mean() Out[84]: Timedelta('31 days 00:01:41') In [85]: td.quantile(.1) Out[85]: Timedelta('31 days 00:00:00')
plot(kind='kde') now accepts the optional parameters bw_method and ind, passed to scipy.stats.gaussian_kde() (for scipy >= 0.11.0) to set the bandwidth, and to gkde.evaluate() to specify the indices at which it is evaluated, respectively. See scipy docs. (GH4298)
DataFrame constructor now accepts a numpy masked record array (GH3478)
The new vectorized string method extract return regular expression matches more conveniently.
In [86]: Series(['a1', 'b2', 'c3']).str.extract('[ab](\d)') Out[86]: 0 1 1 2 2 NaN dtype: object
Elements that do not match return NaN. Extracting a regular expression with more than one group returns a DataFrame with one column per group.
In [87]: Series(['a1', 'b2', 'c3']).str.extract('([ab])(\d)') Out[87]: 0 1 0 a 1 1 b 2 2 NaN NaN [3 rows x 2 columns]
Elements that do not match return a row of NaN. Thus, a Series of messy strings can be converted into a like-indexed Series or DataFrame of cleaned-up or more useful strings, without necessitating get() to access tuples or re.match objects.
Named groups like
In [88]: Series(['a1', 'b2', 'c3']).str.extract( ....: '(?P<letter>[ab])(?P<digit>\d)') ....: Out[88]: letter digit 0 a 1 1 b 2 2 NaN NaN [3 rows x 2 columns]
and optional groups can also be used.
In [89]: Series(['a1', 'b2', '3']).str.extract( ....: '(?P<letter>[ab])?(?P<digit>\d)') ....: Out[89]: letter digit 0 a 1 1 b 2 2 NaN 3 [3 rows x 2 columns]
read_stata now accepts Stata 13 format (GH4291)
read_fwf now infers the column specifications from the first 100 rows of the file if the data has correctly separated and properly aligned columns using the delimiter provided to the function (GH4488).
support for nanosecond times as an offset
Warning
These operations require numpy >= 1.7
Period conversions in the range of seconds and below were reworked and extended up to nanoseconds. Periods in the nanosecond range are now available.
In [90]: date_range('2013-01-01', periods=5, freq='5N') Out[90]: DatetimeIndex(['2013-01-01', '2013-01-01', '2013-01-01', '2013-01-01', '2013-01-01'], dtype='datetime64[ns]', freq='5N')
or with frequency as offset
In [91]: date_range('2013-01-01', periods=5, freq=pd.offsets.Nano(5)) Out[91]: DatetimeIndex(['2013-01-01', '2013-01-01', '2013-01-01', '2013-01-01', '2013-01-01'], dtype='datetime64[ns]', freq='5N')
Timestamps can be modified in the nanosecond range
In [92]: t = Timestamp('20130101 09:01:02') In [93]: t + pd.datetools.Nano(123) Out[93]: Timestamp('2013-01-01 09:01:02.000000123')
A new method, isin for DataFrames, which plays nicely with boolean indexing. The argument to isin, what we’re comparing the DataFrame to, can be a DataFrame, Series, dict, or array of values. See the docs for more.
To get the rows where any of the conditions are met:
In [94]: dfi = DataFrame({'A': [1, 2, 3, 4], 'B': ['a', 'b', 'f', 'n']}) In [95]: dfi Out[95]: A B 0 1 a 1 2 b 2 3 f 3 4 n [4 rows x 2 columns] In [96]: other = DataFrame({'A': [1, 3, 3, 7], 'B': ['e', 'f', 'f', 'e']}) In [97]: mask = dfi.isin(other) In [98]: mask Out[98]: A B 0 True False 1 False False 2 True True 3 False False [4 rows x 2 columns] In [99]: dfi[mask.any(1)] Out[99]: A B 0 1 a 2 3 f [2 rows x 2 columns]
Series now supports a to_frame method to convert it to a single-column DataFrame (GH5164)
All R datasets listed here http://stat.ethz.ch/R-manual/R-devel/library/datasets/html/00Index.html can now be loaded into Pandas objects
# note that pandas.rpy was deprecated in v0.16.0 import pandas.rpy.common as com com.load_data('Titanic')
tz_localize can infer a fall daylight savings transition based on the structure of the unlocalized data (GH4230), see the docs
DatetimeIndex is now in the API documentation, see the docs
json_normalize() is a new method to allow you to create a flat table from semi-structured JSON data. See the docs (GH1067)
Added PySide support for the qtpandas DataFrameModel and DataFrameWidget.
Python csv parser now supports usecols (GH4335)
Frequencies gained several new offsets:
DataFrame has a new interpolate method, similar to Series (GH4434, GH1892)
In [100]: df = DataFrame({'A': [1, 2.1, np.nan, 4.7, 5.6, 6.8], .....: 'B': [.25, np.nan, np.nan, 4, 12.2, 14.4]}) .....: In [101]: df.interpolate() Out[101]: A B 0 1.0 0.25 1 2.1 1.50 2 3.4 2.75 3 4.7 4.00 4 5.6 12.20 5 6.8 14.40 [6 rows x 2 columns]
Additionally, the method argument to interpolate has been expanded to include 'nearest', 'zero', 'slinear', 'quadratic', 'cubic', 'barycentric', 'krogh', 'piecewise_polynomial', 'pchip', `polynomial`, 'spline' The new methods require scipy. Consult the Scipy reference guide and documentation for more information about when the various methods are appropriate. See the docs.
Interpolate now also accepts a limit keyword argument. This works similar to fillna‘s limit:
In [102]: ser = Series([1, 3, np.nan, np.nan, np.nan, 11]) In [103]: ser.interpolate(limit=2) Out[103]: 0 1 1 3 2 5 3 7 4 NaN 5 11 dtype: float64
Added wide_to_long panel data convenience function. See the docs.
In [104]: np.random.seed(123) In [105]: df = pd.DataFrame({"A1970" : {0 : "a", 1 : "b", 2 : "c"}, .....: "A1980" : {0 : "d", 1 : "e", 2 : "f"}, .....: "B1970" : {0 : 2.5, 1 : 1.2, 2 : .7}, .....: "B1980" : {0 : 3.2, 1 : 1.3, 2 : .1}, .....: "X" : dict(zip(range(3), np.random.randn(3))) .....: }) .....: In [106]: df["id"] = df.index In [107]: df Out[107]: A1970 A1980 B1970 B1980 X id 0 a d 2.5 3.2 -1.085631 0 1 b e 1.2 1.3 0.997345 1 2 c f 0.7 0.1 0.282978 2 [3 rows x 6 columns] In [108]: wide_to_long(df, ["A", "B"], i="id", j="year") Out[108]: X A B id year 0 1970 -1.085631 a 2.5 1 1970 0.997345 b 1.2 2 1970 0.282978 c 0.7 0 1980 -1.085631 d 3.2 1 1980 0.997345 e 1.3 2 1980 0.282978 f 0.1 [6 rows x 3 columns]
- to_csv now takes a date_format keyword argument that specifies how output datetime objects should be formatted. Datetimes encountered in the index, columns, and values will all have this formatting applied. (GH4313)
- DataFrame.plot will scatter plot x versus y by passing kind='scatter' (GH2215)
- Added support for Google Analytics v3 API segment IDs that also supports v2 IDs. (GH5271)
Experimental¶
The new eval() function implements expression evaluation using numexpr behind the scenes. This results in large speedups for complicated expressions involving large DataFrames/Series. For example,
In [109]: nrows, ncols = 20000, 100 In [110]: df1, df2, df3, df4 = [DataFrame(randn(nrows, ncols)) .....: for _ in range(4)] .....:
# eval with NumExpr backend In [111]: %timeit pd.eval('df1 + df2 + df3 + df4') 100 loops, best of 3: 14.9 ms per loop
# pure Python evaluation In [112]: %timeit df1 + df2 + df3 + df4 10 loops, best of 3: 24.4 ms per loop
For more details, see the the docs
Similar to pandas.eval, DataFrame has a new DataFrame.eval method that evaluates an expression in the context of the DataFrame. For example,
In [113]: df = DataFrame(randn(10, 2), columns=['a', 'b']) In [114]: df.eval('a + b') Out[114]: 0 -0.685204 1 1.589745 2 0.325441 3 -1.784153 4 -0.432893 5 0.171850 6 1.895919 7 3.065587 8 -0.092759 9 1.391365 dtype: float64
query() method has been added that allows you to select elements of a DataFrame using a natural query syntax nearly identical to Python syntax. For example,
In [115]: n = 20 In [116]: df = DataFrame(np.random.randint(n, size=(n, 3)), columns=['a', 'b', 'c']) In [117]: df.query('a < b < c') Out[117]: a b c 11 1 5 8 15 8 16 19 [2 rows x 3 columns]
selects all the rows of df where a < b < c evaluates to True. For more details see the the docs.
pd.read_msgpack() and pd.to_msgpack() are now a supported method of serialization of arbitrary pandas (and python objects) in a lightweight portable binary format. See the docs
Warning
Since this is an EXPERIMENTAL LIBRARY, the storage format may not be stable until a future release.
In [118]: df = DataFrame(np.random.rand(5,2),columns=list('AB')) In [119]: df.to_msgpack('foo.msg') In [120]: pd.read_msgpack('foo.msg') Out[120]: A B 0 0.251082 0.017357 1 0.347915 0.929879 2 0.546233 0.203368 3 0.064942 0.031722 4 0.355309 0.524575 [5 rows x 2 columns] In [121]: s = Series(np.random.rand(5),index=date_range('20130101',periods=5)) In [122]: pd.to_msgpack('foo.msg', df, s) In [123]: pd.read_msgpack('foo.msg') Out[123]: [ A B 0 0.251082 0.017357 1 0.347915 0.929879 2 0.546233 0.203368 3 0.064942 0.031722 4 0.355309 0.524575 [5 rows x 2 columns], 2013-01-01 0.022321 2013-01-02 0.227025 2013-01-03 0.383282 2013-01-04 0.193225 2013-01-05 0.110977 Freq: D, dtype: float64]
You can pass iterator=True to iterator over the unpacked results
In [124]: for o in pd.read_msgpack('foo.msg',iterator=True): .....: print o .....: A B 0 0.251082 0.017357 1 0.347915 0.929879 2 0.546233 0.203368 3 0.064942 0.031722 4 0.355309 0.524575 [5 rows x 2 columns] 2013-01-01 0.022321 2013-01-02 0.227025 2013-01-03 0.383282 2013-01-04 0.193225 2013-01-05 0.110977 Freq: D, dtype: float64
pandas.io.gbq provides a simple way to extract from, and load data into, Google’s BigQuery Data Sets by way of pandas DataFrames. BigQuery is a high performance SQL-like database service, useful for performing ad-hoc queries against extremely large datasets. See the docs
from pandas.io import gbq # A query to select the average monthly temperatures in the # in the year 2000 across the USA. The dataset, # publicata:samples.gsod, is available on all BigQuery accounts, # and is based on NOAA gsod data. query = """SELECT station_number as STATION, month as MONTH, AVG(mean_temp) as MEAN_TEMP FROM publicdata:samples.gsod WHERE YEAR = 2000 GROUP BY STATION, MONTH ORDER BY STATION, MONTH ASC""" # Fetch the result set for this query # Your Google BigQuery Project ID # To find this, see your dashboard: # https://code.google.com/apis/console/b/0/?noredirect projectid = xxxxxxxxx; df = gbq.read_gbq(query, project_id = projectid) # Use pandas to process and reshape the dataset df2 = df.pivot(index='STATION', columns='MONTH', values='MEAN_TEMP') df3 = pandas.concat([df2.min(), df2.mean(), df2.max()], axis=1,keys=["Min Tem", "Mean Temp", "Max Temp"])
The resulting DataFrame is:
> df3 Min Tem Mean Temp Max Temp MONTH 1 -53.336667 39.827892 89.770968 2 -49.837500 43.685219 93.437932 3 -77.926087 48.708355 96.099998 4 -82.892858 55.070087 97.317240 5 -92.378261 61.428117 102.042856 6 -77.703334 65.858888 102.900000 7 -87.821428 68.169663 106.510714 8 -89.431999 68.614215 105.500000 9 -86.611112 63.436935 107.142856 10 -78.209677 56.880838 92.103333 11 -50.125000 48.861228 94.996428 12 -50.332258 42.286879 94.396774
Warning
To use this module, you will need a BigQuery account. See <https://cloud.google.com/products/big-query> for details.
As of 10/10/13, there is a bug in Google’s API preventing result sets from being larger than 100,000 rows. A patch is scheduled for the week of 10/14/13.
Internal Refactoring¶
In 0.13.0 there is a major refactor primarily to subclass Series from NDFrame, which is the base class currently for DataFrame and Panel, to unify methods and behaviors. Series formerly subclassed directly from ndarray. (GH4080, GH3862, GH816)
Warning
There are two potential incompatibilities from < 0.13.0
Using certain numpy functions would previously return a Series if passed a Series as an argument. This seems only to affect np.ones_like, np.empty_like, np.diff and np.where. These now return ndarrays.
In [125]: s = Series([1,2,3,4])
Numpy Usage
In [126]: np.ones_like(s) Out[126]: array([1, 1, 1, 1], dtype=int64) In [127]: np.diff(s) Out[127]: array([1, 1, 1], dtype=int64) In [128]: np.where(s>1,s,np.nan) Out[128]: array([ nan, 2., 3., 4.])
Pandonic Usage
In [129]: Series(1,index=s.index) Out[129]: 0 1 1 1 2 1 3 1 dtype: int64 In [130]: s.diff() Out[130]: 0 NaN 1 1 2 1 3 1 dtype: float64 In [131]: s.where(s>1) Out[131]: 0 NaN 1 2 2 3 3 4 dtype: float64
Passing a Series directly to a cython function expecting an ndarray type will no long work directly, you must pass Series.values, See Enhancing Performance
Series(0.5) would previously return the scalar 0.5, instead this will return a 1-element Series
This change breaks rpy2<=2.3.8. an Issue has been opened against rpy2 and a workaround is detailed in GH5698. Thanks @JanSchulz.
Pickle compatibility is preserved for pickles created prior to 0.13. These must be unpickled with pd.read_pickle, see Pickling.
Refactor of series.py/frame.py/panel.py to move common code to generic.py
- added _setup_axes to created generic NDFrame structures
- moved methods
- from_axes,_wrap_array,axes,ix,loc,iloc,shape,empty,swapaxes,transpose,pop
- __iter__,keys,__contains__,__len__,__neg__,__invert__
- convert_objects,as_blocks,as_matrix,values
- __getstate__,__setstate__ (compat remains in frame/panel)
- __getattr__,__setattr__
- _indexed_same,reindex_like,align,where,mask
- fillna,replace (Series replace is now consistent with DataFrame)
- filter (also added axis argument to selectively filter on a different axis)
- reindex,reindex_axis,take
- truncate (moved to become part of NDFrame)
These are API changes which make Panel more consistent with DataFrame
- swapaxes on a Panel with the same axes specified now return a copy
- support attribute access for setting
- filter supports the same API as the original DataFrame filter
Reindex called with no arguments will now return a copy of the input object
TimeSeries is now an alias for Series. the property is_time_series can be used to distinguish (if desired)
Refactor of Sparse objects to use BlockManager
- Created a new block type in internals, SparseBlock, which can hold multi-dtypes and is non-consolidatable. SparseSeries and SparseDataFrame now inherit more methods from there hierarchy (Series/DataFrame), and no longer inherit from SparseArray (which instead is the object of the SparseBlock)
- Sparse suite now supports integration with non-sparse data. Non-float sparse data is supportable (partially implemented)
- Operations on sparse structures within DataFrames should preserve sparseness, merging type operations will convert to dense (and back to sparse), so might be somewhat inefficient
- enable setitem on SparseSeries for boolean/integer/slices
- SparsePanels implementation is unchanged (e.g. not using BlockManager, needs work)
added ftypes method to Series/DataFrame, similar to dtypes, but indicates if the underlying is sparse/dense (as well as the dtype)
All NDFrame objects can now use __finalize__() to specify various values to propagate to new objects from an existing one (e.g. name in Series will follow more automatically now)
Internal type checking is now done via a suite of generated classes, allowing isinstance(value, klass) without having to directly import the klass, courtesy of @jtratner
Bug in Series update where the parent frame is not updating its cache based on changes (GH4080) or types (GH3217), fillna (GH3386)
Refactor Series.reindex to core/generic.py (GH4604, GH4618), allow method= in reindexing on a Series to work
Series.copy no longer accepts the order parameter and is now consistent with NDFrame copy
Refactor rename methods to core/generic.py; fixes Series.rename for (GH4605), and adds rename with the same signature for Panel
Refactor clip methods to core/generic.py (GH4798)
Refactor of _get_numeric_data/_get_bool_data to core/generic.py, allowing Series/Panel functionality
Series (for index) / Panel (for items) now allow attribute access to its elements (GH1903)
In [132]: s = Series([1,2,3],index=list('abc')) In [133]: s.b Out[133]: 2 In [134]: s.a = 5 In [135]: s Out[135]: a 5 b 2 c 3 dtype: int64
Bug Fixes¶
See V0.13.0 Bug Fixes for an extensive list of bugs that have been fixed in 0.13.0.
See the full release notes or issue tracker on GitHub for a complete list of all API changes, Enhancements and Bug Fixes.
v0.12.0 (July 24, 2013)¶
This is a major release from 0.11.0 and includes several new features and enhancements along with a large number of bug fixes.
Highlights include a consistent I/O API naming scheme, routines to read html, write multi-indexes to csv files, read & write STATA data files, read & write JSON format files, Python 3 support for HDFStore, filtering of groupby expressions via filter, and a revamped replace routine that accepts regular expressions.
API changes¶
The I/O API is now much more consistent with a set of top level reader functions accessed like pd.read_csv() that generally return a pandas object.
- read_csv
- read_excel
- read_hdf
- read_sql
- read_json
- read_html
- read_stata
- read_clipboard
The corresponding writer functions are object methods that are accessed like df.to_csv()
- to_csv
- to_excel
- to_hdf
- to_sql
- to_json
- to_html
- to_stata
- to_clipboard
Fix modulo and integer division on Series,DataFrames to act similary to float dtypes to return np.nan or np.inf as appropriate (GH3590). This correct a numpy bug that treats integer and float dtypes differently.
In [1]: p = DataFrame({ 'first' : [4,5,8], 'second' : [0,0,3] }) In [2]: p % 0 Out[2]: first second 0 NaN NaN 1 NaN NaN 2 NaN NaN [3 rows x 2 columns] In [3]: p % p Out[3]: first second 0 0 NaN 1 0 NaN 2 0 0 [3 rows x 2 columns] In [4]: p / p Out[4]: first second 0 1 NaN 1 1 NaN 2 1 1 [3 rows x 2 columns] In [5]: p / 0 Out[5]: first second 0 inf NaN 1 inf NaN 2 inf inf [3 rows x 2 columns]Add squeeze keyword to groupby to allow reduction from DataFrame -> Series if groups are unique. This is a Regression from 0.10.1. We are reverting back to the prior behavior. This means groupby will return the same shaped objects whether the groups are unique or not. Revert this issue (GH2893) with (GH3596).
In [6]: df2 = DataFrame([{"val1": 1, "val2" : 20}, {"val1":1, "val2": 19}, ...: {"val1":1, "val2": 27}, {"val1":1, "val2": 12}]) ...: In [7]: def func(dataf): ...: return dataf["val2"] - dataf["val2"].mean() ...: # squeezing the result frame to a series (because we have unique groups) In [8]: df2.groupby("val1", squeeze=True).apply(func) Out[8]: 0 0.5 1 -0.5 2 7.5 3 -7.5 Name: 1, dtype: float64 # no squeezing (the default, and behavior in 0.10.1) In [9]: df2.groupby("val1").apply(func) Out[9]: val2 0 1 2 3 val1 1 0.5 -0.5 7.5 -7.5 [1 rows x 4 columns]Raise on iloc when boolean indexing with a label based indexer mask e.g. a boolean Series, even with integer labels, will raise. Since iloc is purely positional based, the labels on the Series are not alignable (GH3631)
This case is rarely used, and there are plently of alternatives. This preserves the iloc API to be purely positional based.
In [10]: df = DataFrame(lrange(5), list('ABCDE'), columns=['a']) In [11]: mask = (df.a%2 == 0) In [12]: mask Out[12]: A True B False C True D False E True Name: a, dtype: bool # this is what you should use In [13]: df.loc[mask] Out[13]: a A 0 C 2 E 4 [3 rows x 1 columns] # this will work as well In [14]: df.iloc[mask.values] Out[14]: a A 0 C 2 E 4 [3 rows x 1 columns]df.iloc[mask] will raise a ValueError
The raise_on_error argument to plotting functions is removed. Instead, plotting functions raise a TypeError when the dtype of the object is object to remind you to avoid object arrays whenever possible and thus you should cast to an appropriate numeric dtype if you need to plot something.
Add colormap keyword to DataFrame plotting methods. Accepts either a matplotlib colormap object (ie, matplotlib.cm.jet) or a string name of such an object (ie, ‘jet’). The colormap is sampled to select the color for each column. Please see Colormaps for more information. (GH3860)
DataFrame.interpolate() is now deprecated. Please use DataFrame.fillna() and DataFrame.replace() instead. (GH3582, GH3675, GH3676)
the method and axis arguments of DataFrame.replace() are deprecated
DataFrame.replace ‘s infer_types parameter is removed and now performs conversion by default. (GH3907)
Add the keyword allow_duplicates to DataFrame.insert to allow a duplicate column to be inserted if True, default is False (same as prior to 0.12) (GH3679)
IO api
added top-level function read_excel to replace the following, The original API is deprecated and will be removed in a future version
from pandas.io.parsers import ExcelFile xls = ExcelFile('path_to_file.xls') xls.parse('Sheet1', index_col=None, na_values=['NA'])With
import pandas as pd pd.read_excel('path_to_file.xls', 'Sheet1', index_col=None, na_values=['NA'])added top-level function read_sql that is equivalent to the following
from pandas.io.sql import read_frame read_frame(....)DataFrame.to_html and DataFrame.to_latex now accept a path for their first argument (GH3702)
Do not allow astypes on datetime64[ns] except to object, and timedelta64[ns] to object/int (GH3425)
The behavior of datetime64 dtypes has changed with respect to certain so-called reduction operations (GH3726). The following operations now raise a TypeError when perfomed on a Series and return an empty Series when performed on a DataFrame similar to performing these operations on, for example, a DataFrame of slice objects:
- sum, prod, mean, std, var, skew, kurt, corr, and cov
read_html now defaults to None when reading, and falls back on bs4 + html5lib when lxml fails to parse. a list of parsers to try until success is also valid
The internal pandas class hierarchy has changed (slightly). The previous PandasObject now is called PandasContainer and a new PandasObject has become the baseclass for PandasContainer as well as Index, Categorical, GroupBy, SparseList, and SparseArray (+ their base classes). Currently, PandasObject provides string methods (from StringMixin). (GH4090, GH4092)
New StringMixin that, given a __unicode__ method, gets python 2 and python 3 compatible string methods (__str__, __bytes__, and __repr__). Plus string safety throughout. Now employed in many places throughout the pandas library. (GH4090, GH4092)
I/O Enhancements¶
pd.read_html() can now parse HTML strings, files or urls and return DataFrames, courtesy of @cpcloud. (GH3477, GH3605, GH3606, GH3616). It works with a single parser backend: BeautifulSoup4 + html5lib See the docs
You can use pd.read_html() to read the output from DataFrame.to_html() like so
In [15]: df = DataFrame({'a': range(3), 'b': list('abc')}) In [16]: print(df) a b 0 0 a 1 1 b 2 2 c [3 rows x 2 columns] In [17]: html = df.to_html() In [18]: alist = pd.read_html(html, index_col=0) In [19]: print(df == alist[0]) a b 0 True True 1 True True 2 True True [3 rows x 2 columns]Note that alist here is a Python list so pd.read_html() and DataFrame.to_html() are not inverses.
- pd.read_html() no longer performs hard conversion of date strings (GH3656).
Warning
You may have to install an older version of BeautifulSoup4, See the installation docs
Added module for reading and writing Stata files: pandas.io.stata (GH1512) accessable via read_stata top-level function for reading, and to_stata DataFrame method for writing, See the docs
Added module for reading and writing json format files: pandas.io.json accessable via read_json top-level function for reading, and to_json DataFrame method for writing, See the docs various issues (GH1226, GH3804, GH3876, GH3867, GH1305)
MultiIndex column support for reading and writing csv format files
The header option in read_csv now accepts a list of the rows from which to read the index.
The option, tupleize_cols can now be specified in both to_csv and read_csv, to provide compatiblity for the pre 0.12 behavior of writing and reading MultIndex columns via a list of tuples. The default in 0.12 is to write lists of tuples and not interpret list of tuples as a MultiIndex column.
Note: The default behavior in 0.12 remains unchanged from prior versions, but starting with 0.13, the default to write and read MultiIndex columns will be in the new format. (GH3571, GH1651, GH3141)
If an index_col is not specified (e.g. you don’t have an index, or wrote it with df.to_csv(..., index=False), then any names on the columns index will be lost.
In [20]: from pandas.util.testing import makeCustomDataframe as mkdf In [21]: df = mkdf(5,3,r_idx_nlevels=2,c_idx_nlevels=4) In [22]: df.to_csv('mi.csv',tupleize_cols=False) In [23]: print(open('mi.csv').read()) C0,,C_l0_g0,C_l0_g1,C_l0_g2 C1,,C_l1_g0,C_l1_g1,C_l1_g2 C2,,C_l2_g0,C_l2_g1,C_l2_g2 C3,,C_l3_g0,C_l3_g1,C_l3_g2 R0,R1,,, R_l0_g0,R_l1_g0,R0C0,R0C1,R0C2 R_l0_g1,R_l1_g1,R1C0,R1C1,R1C2 R_l0_g2,R_l1_g2,R2C0,R2C1,R2C2 R_l0_g3,R_l1_g3,R3C0,R3C1,R3C2 R_l0_g4,R_l1_g4,R4C0,R4C1,R4C2 In [24]: pd.read_csv('mi.csv',header=[0,1,2,3],index_col=[0,1],tupleize_cols=False) Out[24]: C0 C_l0_g0 C_l0_g1 C_l0_g2 C1 C_l1_g0 C_l1_g1 C_l1_g2 C2 C_l2_g0 C_l2_g1 C_l2_g2 C3 C_l3_g0 C_l3_g1 C_l3_g2 R0 R1 R_l0_g0 R_l1_g0 R0C0 R0C1 R0C2 R_l0_g1 R_l1_g1 R1C0 R1C1 R1C2 R_l0_g2 R_l1_g2 R2C0 R2C1 R2C2 R_l0_g3 R_l1_g3 R3C0 R3C1 R3C2 R_l0_g4 R_l1_g4 R4C0 R4C1 R4C2 [5 rows x 3 columns]Support for HDFStore (via PyTables 3.0.0) on Python3
Iterator support via read_hdf that automatically opens and closes the store when iteration is finished. This is only for tables
In [25]: path = 'store_iterator.h5' In [26]: DataFrame(randn(10,2)).to_hdf(path,'df',table=True) In [27]: for df in read_hdf(path,'df', chunksize=3): ....: print df ....: 0 1 0 0.713216 -0.778461 1 -0.661062 0.862877 2 0.344342 0.149565 0 1 3 -0.626968 -0.875772 4 -0.930687 -0.218983 5 0.949965 -0.442354 0 1 6 -0.402985 1.111358 7 -0.241527 -0.670477 8 0.049355 0.632633 0 1 9 -1.502767 -1.225492read_csv will now throw a more informative error message when a file contains no columns, e.g., all newline characters
Other Enhancements¶
DataFrame.replace() now allows regular expressions on contained Series with object dtype. See the examples section in the regular docs Replacing via String Expression
For example you can do
In [25]: df = DataFrame({'a': list('ab..'), 'b': [1, 2, 3, 4]}) In [26]: df.replace(regex=r'\s*\.\s*', value=np.nan) Out[26]: a b 0 a 1 1 b 2 2 NaN 3 3 NaN 4 [4 rows x 2 columns]to replace all occurrences of the string '.' with zero or more instances of surrounding whitespace with NaN.
Regular string replacement still works as expected. For example, you can do
In [27]: df.replace('.', np.nan) Out[27]: a b 0 a 1 1 b 2 2 NaN 3 3 NaN 4 [4 rows x 2 columns]to replace all occurrences of the string '.' with NaN.
pd.melt() now accepts the optional parameters var_name and value_name to specify custom column names of the returned DataFrame.
pd.set_option() now allows N option, value pairs (GH3667).
Let’s say that we had an option 'a.b' and another option 'b.c'. We can set them at the same time:
In [28]: pd.get_option('a.b') Out[28]: 2 In [29]: pd.get_option('b.c') Out[29]: 3 In [30]: pd.set_option('a.b', 1, 'b.c', 4) In [31]: pd.get_option('a.b') Out[31]: 1 In [32]: pd.get_option('b.c') Out[32]: 4The filter method for group objects returns a subset of the original object. Suppose we want to take only elements that belong to groups with a group sum greater than 2.
In [33]: sf = Series([1, 1, 2, 3, 3, 3]) In [34]: sf.groupby(sf).filter(lambda x: x.sum() > 2) Out[34]: 3 3 4 3 5 3 dtype: int64The argument of filter must a function that, applied to the group as a whole, returns True or False.
Another useful operation is filtering out elements that belong to groups with only a couple members.
In [35]: dff = DataFrame({'A': np.arange(8), 'B': list('aabbbbcc')}) In [36]: dff.groupby('B').filter(lambda x: len(x) > 2) Out[36]: A B 2 2 b 3 3 b 4 4 b 5 5 b [4 rows x 2 columns]Alternatively, instead of dropping the offending groups, we can return a like-indexed objects where the groups that do not pass the filter are filled with NaNs.
In [37]: dff.groupby('B').filter(lambda x: len(x) > 2, dropna=False) Out[37]: A B 0 NaN NaN 1 NaN NaN 2 2 b 3 3 b 4 4 b 5 5 b 6 NaN NaN 7 NaN NaN [8 rows x 2 columns]Series and DataFrame hist methods now take a figsize argument (GH3834)
DatetimeIndexes no longer try to convert mixed-integer indexes during join operations (GH3877)
Timestamp.min and Timestamp.max now represent valid Timestamp instances instead of the default datetime.min and datetime.max (respectively), thanks @SleepingPills
read_html now raises when no tables are found and BeautifulSoup==4.2.0 is detected (GH4214)
Experimental Features¶
Added experimental CustomBusinessDay class to support DateOffsets with custom holiday calendars and custom weekmasks. (GH2301)
Note
This uses the numpy.busdaycalendar API introduced in Numpy 1.7 and therefore requires Numpy 1.7.0 or newer.
In [38]: from pandas.tseries.offsets import CustomBusinessDay In [39]: from datetime import datetime # As an interesting example, let's look at Egypt where # a Friday-Saturday weekend is observed. In [40]: weekmask_egypt = 'Sun Mon Tue Wed Thu' # They also observe International Workers' Day so let's # add that for a couple of years In [41]: holidays = ['2012-05-01', datetime(2013, 5, 1), np.datetime64('2014-05-01')] In [42]: bday_egypt = CustomBusinessDay(holidays=holidays, weekmask=weekmask_egypt) In [43]: dt = datetime(2013, 4, 30) In [44]: print(dt + 2 * bday_egypt) 2013-05-05 00:00:00 In [45]: dts = date_range(dt, periods=5, freq=bday_egypt) In [46]: print(Series(dts.weekday, dts).map(Series('Mon Tue Wed Thu Fri Sat Sun'.split()))) 2013-04-30 Tue 2013-05-02 Thu 2013-05-05 Sun 2013-05-06 Mon 2013-05-07 Tue Freq: C, dtype: object
Bug Fixes¶
Plotting functions now raise a TypeError before trying to plot anything if the associated objects have have a dtype of object (GH1818, GH3572, GH3911, GH3912), but they will try to convert object arrays to numeric arrays if possible so that you can still plot, for example, an object array with floats. This happens before any drawing takes place which elimnates any spurious plots from showing up.
fillna methods now raise a TypeError if the value parameter is a list or tuple.
Series.str now supports iteration (GH3638). You can iterate over the individual elements of each string in the Series. Each iteration yields yields a Series with either a single character at each index of the original Series or NaN. For example,
In [47]: strs = 'go', 'bow', 'joe', 'slow' In [48]: ds = Series(strs) In [49]: for s in ds.str: ....: print(s) ....: 0 g 1 b 2 j 3 s dtype: object 0 o 1 o 2 o 3 l dtype: object 0 NaN 1 w 2 e 3 o dtype: object 0 NaN 1 NaN 2 NaN 3 w dtype: object In [50]: s Out[50]: 0 NaN 1 NaN 2 NaN 3 w dtype: object In [51]: s.dropna().values.item() == 'w' Out[51]: TrueThe last element yielded by the iterator will be a Series containing the last element of the longest string in the Series with all other elements being NaN. Here since 'slow' is the longest string and there are no other strings with the same length 'w' is the only non-null string in the yielded Series.
HDFStore
- will retain index attributes (freq,tz,name) on recreation (GH3499)
- will warn with a AttributeConflictWarning if you are attempting to append an index with a different frequency than the existing, or attempting to append an index with a different name than the existing
- support datelike columns with a timezone as data_columns (GH2852)
Non-unique index support clarified (GH3468).
- Fix assigning a new index to a duplicate index in a DataFrame would fail (GH3468)
- Fix construction of a DataFrame with a duplicate index
- ref_locs support to allow duplicative indices across dtypes, allows iget support to always find the index (even across dtypes) (GH2194)
- applymap on a DataFrame with a non-unique index now works (removed warning) (GH2786), and fix (GH3230)
- Fix to_csv to handle non-unique columns (GH3495)
- Duplicate indexes with getitem will return items in the correct order (GH3455, GH3457) and handle missing elements like unique indices (GH3561)
- Duplicate indexes with and empty DataFrame.from_records will return a correct frame (GH3562)
- Concat to produce a non-unique columns when duplicates are across dtypes is fixed (GH3602)
- Allow insert/delete to non-unique columns (GH3679)
- Non-unique indexing with a slice via loc and friends fixed (GH3659)
- Allow insert/delete to non-unique columns (GH3679)
- Extend reindex to correctly deal with non-unique indices (GH3679)
- DataFrame.itertuples() now works with frames with duplicate column names (GH3873)
- Bug in non-unique indexing via iloc (GH4017); added takeable argument to reindex for location-based taking
- Allow non-unique indexing in series via .ix/.loc and __getitem__ (GH4246)
- Fixed non-unique indexing memory allocation issue with .ix/.loc (GH4280)
DataFrame.from_records did not accept empty recarrays (GH3682)
read_html now correctly skips tests (GH3741)
Fixed a bug where DataFrame.replace with a compiled regular expression in the to_replace argument wasn’t working (GH3907)
Improved network test decorator to catch IOError (and therefore URLError as well). Added with_connectivity_check decorator to allow explicitly checking a website as a proxy for seeing if there is network connectivity. Plus, new optional_args decorator factory for decorators. (GH3910, GH3914)
Fixed testing issue where too many sockets where open thus leading to a connection reset issue (GH3982, GH3985, GH4028, GH4054)
Fixed failing tests in test_yahoo, test_google where symbols were not retrieved but were being accessed (GH3982, GH3985, GH4028, GH4054)
Series.hist will now take the figure from the current environment if one is not passed
Fixed bug where a 1xN DataFrame would barf on a 1xN mask (GH4071)
Fixed running of tox under python3 where the pickle import was getting rewritten in an incompatible way (GH4062, GH4063)
Fixed bug where sharex and sharey were not being passed to grouped_hist (GH4089)
Fixed bug in DataFrame.replace where a nested dict wasn’t being iterated over when regex=False (GH4115)
Fixed bug in the parsing of microseconds when using the format argument in to_datetime (GH4152)
Fixed bug in PandasAutoDateLocator where invert_xaxis triggered incorrectly MilliSecondLocator (GH3990)
Fixed bug in plotting that wasn’t raising on invalid colormap for matplotlib 1.1.1 (GH4215)
Fixed the legend displaying in DataFrame.plot(kind='kde') (GH4216)
Fixed bug where Index slices weren’t carrying the name attribute (GH4226)
Fixed bug in initializing DatetimeIndex with an array of strings in a certain time zone (GH4229)
Fixed bug where html5lib wasn’t being properly skipped (GH4265)
Fixed bug where get_data_famafrench wasn’t using the correct file edges (GH4281)
See the full release notes or issue tracker on GitHub for a complete list.
v0.11.0 (April 22, 2013)¶
This is a major release from 0.10.1 and includes many new features and enhancements along with a large number of bug fixes. The methods of Selecting Data have had quite a number of additions, and Dtype support is now full-fledged. There are also a number of important API changes that long-time pandas users should pay close attention to.
There is a new section in the documentation, 10 Minutes to Pandas, primarily geared to new users.
There is a new section in the documentation, Cookbook, a collection of useful recipes in pandas (and that we want contributions!).
There are several libraries that are now Recommended Dependencies
Selection Choices¶
Starting in 0.11.0, object selection has had a number of user-requested additions in order to support more explicit location based indexing. Pandas now supports three types of multi-axis indexing.
.loc is strictly label based, will raise KeyError when the items are not found, allowed inputs are:
- A single label, e.g. 5 or 'a', (note that 5 is interpreted as a label of the index. This use is not an integer position along the index)
- A list or array of labels ['a', 'b', 'c']
- A slice object with labels 'a':'f', (note that contrary to usual python slices, both the start and the stop are included!)
- A boolean array
See more at Selection by Label
.iloc is strictly integer position based (from 0 to length-1 of the axis), will raise IndexError when the requested indicies are out of bounds. Allowed inputs are:
- An integer e.g. 5
- A list or array of integers [4, 3, 0]
- A slice object with ints 1:7
- A boolean array
See more at Selection by Position
.ix supports mixed integer and label based access. It is primarily label based, but will fallback to integer positional access. .ix is the most general and will support any of the inputs to .loc and .iloc, as well as support for floating point label schemes. .ix is especially useful when dealing with mixed positional and label based hierarchial indexes.
As using integer slices with .ix have different behavior depending on whether the slice is interpreted as position based or label based, it’s usually better to be explicit and use .iloc or .loc.
See more at Advanced Indexing and Advanced Hierarchical.
Selection Deprecations¶
Starting in version 0.11.0, these methods may be deprecated in future versions.
- irow
- icol
- iget_value
See the section Selection by Position for substitutes.
Dtypes¶
Numeric dtypes will propagate and can coexist in DataFrames. If a dtype is passed (either directly via the dtype keyword, a passed ndarray, or a passed Series, then it will be preserved in DataFrame operations. Furthermore, different numeric dtypes will NOT be combined. The following example will give you a taste.
In [1]: df1 = DataFrame(randn(8, 1), columns = ['A'], dtype = 'float32')
In [2]: df1
Out[2]:
A
0 1.392665
1 -0.123497
2 -0.402761
3 -0.246604
4 -0.288433
5 -0.763434
6 2.069526
7 -1.203569
[8 rows x 1 columns]
In [3]: df1.dtypes
Out[3]:
A float32
dtype: object
In [4]: df2 = DataFrame(dict( A = Series(randn(8),dtype='float16'),
...: B = Series(randn(8)),
...: C = Series(randn(8),dtype='uint8') ))
...:
In [5]: df2
Out[5]:
A B C
0 0.591797 -0.038605 0
1 0.841309 -0.460478 1
2 -0.500977 -0.310458 0
3 -0.816406 0.866493 254
4 -0.207031 0.245972 0
5 -0.664062 0.319442 1
6 0.580566 1.378512 1
7 -0.965820 0.292502 255
[8 rows x 3 columns]
In [6]: df2.dtypes
Out[6]:
A float16
B float64
C uint8
dtype: object
# here you get some upcasting
In [7]: df3 = df1.reindex_like(df2).fillna(value=0.0) + df2
In [8]: df3
Out[8]:
A B C
0 1.984462 -0.038605 0
1 0.717812 -0.460478 1
2 -0.903737 -0.310458 0
3 -1.063011 0.866493 254
4 -0.495465 0.245972 0
5 -1.427497 0.319442 1
6 2.650092 1.378512 1
7 -2.169390 0.292502 255
[8 rows x 3 columns]
In [9]: df3.dtypes
Out[9]:
A float32
B float64
C float64
dtype: object
Dtype Conversion¶
This is lower-common-denomicator upcasting, meaning you get the dtype which can accomodate all of the types
In [10]: df3.values.dtype
Out[10]: dtype('float64')
Conversion
In [11]: df3.astype('float32').dtypes
Out[11]:
A float32
B float32
C float32
dtype: object
Mixed Conversion
In [12]: df3['D'] = '1.'
In [13]: df3['E'] = '1'
In [14]: df3.convert_objects(convert_numeric=True).dtypes
Out[14]:
A float32
B float64
C float64
D float64
E int64
dtype: object
# same, but specific dtype conversion
In [15]: df3['D'] = df3['D'].astype('float16')
In [16]: df3['E'] = df3['E'].astype('int32')
In [17]: df3.dtypes
Out[17]:
A float32
B float64
C float64
D float16
E int32
dtype: object
Forcing Date coercion (and setting NaT when not datelike)
In [18]: from datetime import datetime
In [19]: s = Series([datetime(2001,1,1,0,0), 'foo', 1.0, 1,
....: Timestamp('20010104'), '20010105'],dtype='O')
....:
In [20]: s.convert_objects(convert_dates='coerce')
Out[20]:
0 2001-01-01
1 NaT
2 NaT
3 NaT
4 2001-01-04
5 2001-01-05
dtype: datetime64[ns]
Dtype Gotchas¶
Platform Gotchas
Starting in 0.11.0, construction of DataFrame/Series will use default dtypes of int64 and float64, regardless of platform. This is not an apparent change from earlier versions of pandas. If you specify dtypes, they WILL be respected, however (GH2837)
The following will all result in int64 dtypes
In [21]: DataFrame([1,2],columns=['a']).dtypes
Out[21]:
a int64
dtype: object
In [22]: DataFrame({'a' : [1,2] }).dtypes
Out[22]:
a int64
dtype: object
In [23]: DataFrame({'a' : 1 }, index=range(2)).dtypes
Out[23]:
a int64
dtype: object
Keep in mind that DataFrame(np.array([1,2])) WILL result in int32 on 32-bit platforms!
Upcasting Gotchas
Performing indexing operations on integer type data can easily upcast the data. The dtype of the input data will be preserved in cases where nans are not introduced.
In [24]: dfi = df3.astype('int32')
In [25]: dfi['D'] = dfi['D'].astype('int64')
In [26]: dfi
Out[26]:
A B C D E
0 1 0 0 1 1
1 0 0 1 1 1
2 0 0 0 1 1
3 -1 0 254 1 1
4 0 0 0 1 1
5 -1 0 1 1 1
6 2 1 1 1 1
7 -2 0 255 1 1
[8 rows x 5 columns]
In [27]: dfi.dtypes
Out[27]:
A int32
B int32
C int32
D int64
E int32
dtype: object
In [28]: casted = dfi[dfi>0]
In [29]: casted
Out[29]:
A B C D E
0 1 NaN NaN 1 1
1 NaN NaN 1 1 1
2 NaN NaN NaN 1 1
3 NaN NaN 254 1 1
4 NaN NaN NaN 1 1
5 NaN NaN 1 1 1
6 2 1 1 1 1
7 NaN NaN 255 1 1
[8 rows x 5 columns]
In [30]: casted.dtypes
Out[30]:
A float64
B float64
C float64
D int64
E int32
dtype: object
While float dtypes are unchanged.
In [31]: df4 = df3.copy()
In [32]: df4['A'] = df4['A'].astype('float32')
In [33]: df4.dtypes
Out[33]:
A float32
B float64
C float64
D float16
E int32
dtype: object
In [34]: casted = df4[df4>0]
In [35]: casted
Out[35]:
A B C D E
0 1.984462 NaN NaN 1 1
1 0.717812 NaN 1 1 1
2 NaN NaN NaN 1 1
3 NaN 0.866493 254 1 1
4 NaN 0.245972 NaN 1 1
5 NaN 0.319442 1 1 1
6 2.650092 1.378512 1 1 1
7 NaN 0.292502 255 1 1
[8 rows x 5 columns]
In [36]: casted.dtypes
Out[36]:
A float32
B float64
C float64
D float16
E int32
dtype: object
Datetimes Conversion¶
Datetime64[ns] columns in a DataFrame (or a Series) allow the use of np.nan to indicate a nan value, in addition to the traditional NaT, or not-a-time. This allows convenient nan setting in a generic way. Furthermore datetime64[ns] columns are created by default, when passed datetimelike objects (this change was introduced in 0.10.1) (GH2809, GH2810)
In [37]: df = DataFrame(randn(6,2),date_range('20010102',periods=6),columns=['A','B'])
In [38]: df['timestamp'] = Timestamp('20010103')
In [39]: df
Out[39]:
A B timestamp
2001-01-02 1.023958 0.660103 2001-01-03
2001-01-03 1.236475 -2.170629 2001-01-03
2001-01-04 -0.270630 -1.685677 2001-01-03
2001-01-05 -0.440747 -0.115070 2001-01-03
2001-01-06 -0.632102 -0.585977 2001-01-03
2001-01-07 -1.444787 -0.201135 2001-01-03
[6 rows x 3 columns]
# datetime64[ns] out of the box
In [40]: df.get_dtype_counts()
Out[40]:
datetime64[ns] 1
float64 2
dtype: int64
# use the traditional nan, which is mapped to NaT internally
In [41]: df.ix[2:4,['A','timestamp']] = np.nan
In [42]: df
Out[42]:
A B timestamp
2001-01-02 1.023958 0.660103 2001-01-03
2001-01-03 1.236475 -2.170629 2001-01-03
2001-01-04 NaN -1.685677 NaT
2001-01-05 NaN -0.115070 NaT
2001-01-06 -0.632102 -0.585977 2001-01-03
2001-01-07 -1.444787 -0.201135 2001-01-03
[6 rows x 3 columns]
Astype conversion on datetime64[ns] to object, implicity converts NaT to np.nan
In [43]: import datetime
In [44]: s = Series([datetime.datetime(2001, 1, 2, 0, 0) for i in range(3)])
In [45]: s.dtype
Out[45]: dtype('<M8[ns]')
In [46]: s[1] = np.nan
In [47]: s
Out[47]:
0 2001-01-02
1 NaT
2 2001-01-02
dtype: datetime64[ns]
In [48]: s.dtype
Out[48]: dtype('<M8[ns]')
In [49]: s = s.astype('O')
In [50]: s
Out[50]:
0 2001-01-02 00:00:00
1 NaT
2 2001-01-02 00:00:00
dtype: object
In [51]: s.dtype
Out[51]: dtype('O')
API changes¶
- Added to_series() method to indicies, to facilitate the creation of indexers (GH3275)
- HDFStore
- added the method select_column to select a single column from a table as a Series.
- deprecated the unique method, can be replicated by select_column(key,column).unique()
- min_itemsize parameter to append will now automatically create data_columns for passed keys
Enhancements¶
Improved performance of df.to_csv() by up to 10x in some cases. (GH3059)
Numexpr is now a Recommended Dependencies, to accelerate certain types of numerical and boolean operations
Bottleneck is now a Recommended Dependencies, to accelerate certain types of nan operations
HDFStore
support read_hdf/to_hdf API similar to read_csv/to_csv
In [52]: df = DataFrame(dict(A=lrange(5), B=lrange(5))) In [53]: df.to_hdf('store.h5','table',append=True) In [54]: read_hdf('store.h5', 'table', where = ['index>2']) Out[54]: A B 3 3 3 4 4 4 [2 rows x 2 columns]provide dotted attribute access to get from stores, e.g. store.df == store['df']
new keywords iterator=boolean, and chunksize=number_in_a_chunk are provided to support iteration on select and select_as_multiple (GH3076)
You can now select timestamps from an unordered timeseries similarly to an ordered timeseries (GH2437)
You can now select with a string from a DataFrame with a datelike index, in a similar way to a Series (GH3070)
In [55]: idx = date_range("2001-10-1", periods=5, freq='M') In [56]: ts = Series(np.random.rand(len(idx)),index=idx) In [57]: ts['2001'] Out[57]: 2001-10-31 0.663256 2001-11-30 0.079126 2001-12-31 0.587699 Freq: M, dtype: float64 In [58]: df = DataFrame(dict(A = ts)) In [59]: df['2001'] Out[59]: A 2001-10-31 0.663256 2001-11-30 0.079126 2001-12-31 0.587699 [3 rows x 1 columns]Squeeze to possibly remove length 1 dimensions from an object.
In [60]: p = Panel(randn(3,4,4),items=['ItemA','ItemB','ItemC'], ....: major_axis=date_range('20010102',periods=4), ....: minor_axis=['A','B','C','D']) ....: In [61]: p Out[61]: <class 'pandas.core.panel.Panel'> Dimensions: 3 (items) x 4 (major_axis) x 4 (minor_axis) Items axis: ItemA to ItemC Major_axis axis: 2001-01-02 00:00:00 to 2001-01-05 00:00:00 Minor_axis axis: A to D In [62]: p.reindex(items=['ItemA']).squeeze() Out[62]: A B C D 2001-01-02 -1.203403 0.425882 -0.436045 -0.982462 2001-01-03 0.348090 -0.969649 0.121731 0.202798 2001-01-04 1.215695 -0.218549 -0.631381 -0.337116 2001-01-05 0.404238 0.907213 -0.865657 0.483186 [4 rows x 4 columns] In [63]: p.reindex(items=['ItemA'],minor=['B']).squeeze() Out[63]: 2001-01-02 0.425882 2001-01-03 -0.969649 2001-01-04 -0.218549 2001-01-05 0.907213 Freq: D, Name: B, dtype: float64In pd.io.data.Options,
- Fix bug when trying to fetch data for the current month when already past expiry.
- Now using lxml to scrape html instead of BeautifulSoup (lxml was faster).
- New instance variables for calls and puts are automatically created when a method that creates them is called. This works for current month where the instance variables are simply calls and puts. Also works for future expiry months and save the instance variable as callsMMYY or putsMMYY, where MMYY are, respectively, the month and year of the option’s expiry.
- Options.get_near_stock_price now allows the user to specify the month for which to get relevant options data.
- Options.get_forward_data now has optional kwargs near and above_below. This allows the user to specify if they would like to only return forward looking data for options near the current stock price. This just obtains the data from Options.get_near_stock_price instead of Options.get_xxx_data() (GH2758).
Cursor coordinate information is now displayed in time-series plots.
added option display.max_seq_items to control the number of elements printed per sequence pprinting it. (GH2979)
added option display.chop_threshold to control display of small numerical values. (GH2739)
added option display.max_info_rows to prevent verbose_info from being calculated for frames above 1M rows (configurable). (GH2807, GH2918)
value_counts() now accepts a “normalize” argument, for normalized histograms. (GH2710).
DataFrame.from_records now accepts not only dicts but any instance of the collections.Mapping ABC.
added option display.mpl_style providing a sleeker visual style for plots. Based on https://gist.github.com/huyng/816622 (GH3075).
Treat boolean values as integers (values 1 and 0) for numeric operations. (GH2641)
to_html() now accepts an optional “escape” argument to control reserved HTML character escaping (enabled by default) and escapes &, in addition to < and >. (GH2919)
See the full release notes or issue tracker on GitHub for a complete list.
v0.10.1 (January 22, 2013)¶
This is a minor release from 0.10.0 and includes new features, enhancements, and bug fixes. In particular, there is substantial new HDFStore functionality contributed by Jeff Reback.
An undesired API breakage with functions taking the inplace option has been reverted and deprecation warnings added.
API changes¶
- Functions taking an inplace option return the calling object as before. A deprecation message has been added
- Groupby aggregations Max/Min no longer exclude non-numeric data (GH2700)
- Resampling an empty DataFrame now returns an empty DataFrame instead of raising an exception (GH2640)
- The file reader will now raise an exception when NA values are found in an explicitly specified integer column instead of converting the column to float (GH2631)
- DatetimeIndex.unique now returns a DatetimeIndex with the same name and
- timezone instead of an array (GH2563)
New features¶
- MySQL support for database (contribution from Dan Allan)
HDFStore¶
You may need to upgrade your existing data files. Please visit the compatibility section in the main docs.
You can designate (and index) certain columns that you want to be able to perform queries on a table, by passing a list to data_columns
In [1]: store = HDFStore('store.h5')
In [2]: df = DataFrame(randn(8, 3), index=date_range('1/1/2000', periods=8),
...: columns=['A', 'B', 'C'])
...:
In [3]: df['string'] = 'foo'
In [4]: df.ix[4:6,'string'] = np.nan
In [5]: df.ix[7:9,'string'] = 'bar'
In [6]: df['string2'] = 'cool'
In [7]: df
Out[7]:
A B C string string2
2000-01-01 1.885136 -0.183873 2.550850 foo cool
2000-01-02 0.180759 -1.117089 0.061462 foo cool
2000-01-03 -0.294467 -0.591411 -0.876691 foo cool
2000-01-04 3.127110 1.451130 0.045152 foo cool
2000-01-05 -0.242846 1.195819 1.533294 NaN cool
2000-01-06 0.820521 -0.281201 1.651561 NaN cool
2000-01-07 -0.034086 0.252394 -0.498772 foo cool
2000-01-08 -2.290958 -1.601262 -0.256718 bar cool
[8 rows x 5 columns]
# on-disk operations
In [8]: store.append('df', df, data_columns = ['B','C','string','string2'])
In [9]: store.select('df',[ 'B > 0', 'string == foo' ])
Out[9]:
Empty DataFrame
Columns: [A, B, C, string, string2]
Index: []
[0 rows x 5 columns]
# this is in-memory version of this type of selection
In [10]: df[(df.B > 0) & (df.string == 'foo')]
Out[10]:
A B C string string2
2000-01-04 3.127110 1.451130 0.045152 foo cool
2000-01-07 -0.034086 0.252394 -0.498772 foo cool
[2 rows x 5 columns]
Retrieving unique values in an indexable or data column.
# note that this is deprecated as of 0.14.0
# can be replicated by: store.select_column('df','index').unique()
store.unique('df','index')
store.unique('df','string')
You can now store datetime64 in data columns
In [11]: df_mixed = df.copy()
In [12]: df_mixed['datetime64'] = Timestamp('20010102')
In [13]: df_mixed.ix[3:4,['A','B']] = np.nan
In [14]: store.append('df_mixed', df_mixed)
In [15]: df_mixed1 = store.select('df_mixed')
In [16]: df_mixed1
Out[16]:
A B C string string2 datetime64
2000-01-01 1.885136 -0.183873 2.550850 foo cool 2001-01-02
2000-01-02 0.180759 -1.117089 0.061462 foo cool 2001-01-02
2000-01-03 -0.294467 -0.591411 -0.876691 foo cool 2001-01-02
2000-01-04 NaN NaN 0.045152 foo cool 2001-01-02
2000-01-05 -0.242846 1.195819 1.533294 NaN cool 2001-01-02
2000-01-06 0.820521 -0.281201 1.651561 NaN cool 2001-01-02
2000-01-07 -0.034086 0.252394 -0.498772 foo cool 2001-01-02
2000-01-08 -2.290958 -1.601262 -0.256718 bar cool 2001-01-02
[8 rows x 6 columns]
In [17]: df_mixed1.get_dtype_counts()
Out[17]:
datetime64[ns] 1
float64 3
object 2
dtype: int64
You can pass columns keyword to select to filter a list of the return columns, this is equivalent to passing a Term('columns',list_of_columns_to_filter)
In [18]: store.select('df',columns = ['A','B'])
Out[18]:
A B
2000-01-01 1.885136 -0.183873
2000-01-02 0.180759 -1.117089
2000-01-03 -0.294467 -0.591411
2000-01-04 3.127110 1.451130
2000-01-05 -0.242846 1.195819
2000-01-06 0.820521 -0.281201
2000-01-07 -0.034086 0.252394
2000-01-08 -2.290958 -1.601262
[8 rows x 2 columns]
HDFStore now serializes multi-index dataframes when appending tables.
In [19]: index = MultiIndex(levels=[['foo', 'bar', 'baz', 'qux'],
....: ['one', 'two', 'three']],
....: labels=[[0, 0, 0, 1, 1, 2, 2, 3, 3, 3],
....: [0, 1, 2, 0, 1, 1, 2, 0, 1, 2]],
....: names=['foo', 'bar'])
....:
In [20]: df = DataFrame(np.random.randn(10, 3), index=index,
....: columns=['A', 'B', 'C'])
....:
In [21]: df
Out[21]:
A B C
foo bar
foo one 0.239369 0.174122 -1.131794
two -1.948006 0.980347 -0.674429
three -0.361633 -0.761218 1.768215
bar one 0.152288 -0.862613 -0.210968
two -0.859278 1.498195 0.462413
baz two -0.647604 1.511487 -0.727189
three -0.342928 -0.007364 1.427674
qux one 0.104020 2.052171 -1.230963
two -0.019240 -1.713238 0.838912
three -0.637855 0.215109 -1.515362
[10 rows x 3 columns]
In [22]: store.append('mi',df)
In [23]: store.select('mi')
Out[23]:
A B C
foo bar
foo one 0.239369 0.174122 -1.131794
two -1.948006 0.980347 -0.674429
three -0.361633 -0.761218 1.768215
bar one 0.152288 -0.862613 -0.210968
two -0.859278 1.498195 0.462413
baz two -0.647604 1.511487 -0.727189
three -0.342928 -0.007364 1.427674
qux one 0.104020 2.052171 -1.230963
two -0.019240 -1.713238 0.838912
three -0.637855 0.215109 -1.515362
[10 rows x 3 columns]
# the levels are automatically included as data columns
In [24]: store.select('mi', Term('foo=bar'))
Out[24]:
Empty DataFrame
Columns: [A, B, C]
Index: []
[0 rows x 3 columns]
Multi-table creation via append_to_multiple and selection via select_as_multiple can create/select from multiple tables and return a combined result, by using where on a selector table.
In [25]: df_mt = DataFrame(randn(8, 6), index=date_range('1/1/2000', periods=8),
....: columns=['A', 'B', 'C', 'D', 'E', 'F'])
....:
In [26]: df_mt['foo'] = 'bar'
# you can also create the tables individually
In [27]: store.append_to_multiple({ 'df1_mt' : ['A','B'], 'df2_mt' : None }, df_mt, selector = 'df1_mt')
In [28]: store
Out[28]:
<class 'pandas.io.pytables.HDFStore'>
File path: store.h5
/df frame_table (typ->appendable,nrows->8,ncols->5,indexers->[index],dc->[B,C,string,string2])
/df1_mt frame_table (typ->appendable,nrows->8,ncols->2,indexers->[index],dc->[A,B])
/df2_mt frame_table (typ->appendable,nrows->8,ncols->5,indexers->[index])
/df_mixed frame_table (typ->appendable,nrows->8,ncols->6,indexers->[index])
/mi frame_table (typ->appendable_multi,nrows->10,ncols->5,indexers->[index],dc->[bar,foo])
# indiviual tables were created
In [29]: store.select('df1_mt')
Out[29]:
A B
2000-01-01 1.586924 -0.447974
2000-01-02 -0.102206 0.870302
2000-01-03 1.249874 1.458210
2000-01-04 -0.616293 0.150468
2000-01-05 -0.431163 0.016640
2000-01-06 0.800353 -0.451572
2000-01-07 1.239198 0.185437
2000-01-08 -0.040863 0.290110
[8 rows x 2 columns]
In [30]: store.select('df2_mt')
Out[30]:
C D E F foo
2000-01-01 -1.573998 0.630925 -0.071659 -1.277640 bar
2000-01-02 1.275280 -1.199212 1.060780 1.673018 bar
2000-01-03 -0.710542 0.825392 1.557329 1.993441 bar
2000-01-04 0.132104 0.580923 -0.128750 1.445964 bar
2000-01-05 0.904578 -1.645852 -0.688741 0.228006 bar
2000-01-06 0.831767 0.228760 0.932498 -2.200069 bar
2000-01-07 -0.540770 -0.370038 1.298390 1.662964 bar
2000-01-08 -0.096145 1.717830 -0.462446 -0.112019 bar
[8 rows x 5 columns]
# as a multiple
In [31]: store.select_as_multiple(['df1_mt','df2_mt'], where = [ 'A>0','B>0' ], selector = 'df1_mt')
Out[31]:
A B C D E F foo
2000-01-03 1.249874 1.458210 -0.710542 0.825392 1.557329 1.993441 bar
2000-01-07 1.239198 0.185437 -0.540770 -0.370038 1.298390 1.662964 bar
[2 rows x 7 columns]
Enhancements
- HDFStore now can read native PyTables table format tables
- You can pass nan_rep = 'my_nan_rep' to append, to change the default nan representation on disk (which converts to/from np.nan), this defaults to nan.
- You can pass index to append. This defaults to True. This will automagically create indicies on the indexables and data columns of the table
- You can pass chunksize=an integer to append, to change the writing chunksize (default is 50000). This will signficantly lower your memory usage on writing.
- You can pass expectedrows=an integer to the first append, to set the TOTAL number of expectedrows that PyTables will expected. This will optimize read/write performance.
- Select now supports passing start and stop to provide selection space limiting in selection.
- Greatly improved ISO8601 (e.g., yyyy-mm-dd) date parsing for file parsers (GH2698)
- Allow DataFrame.merge to handle combinatorial sizes too large for 64-bit integer (GH2690)
- Series now has unary negation (-series) and inversion (~series) operators (GH2686)
- DataFrame.plot now includes a logx parameter to change the x-axis to log scale (GH2327)
- Series arithmetic operators can now handle constant and ndarray input (GH2574)
- ExcelFile now takes a kind argument to specify the file type (GH2613)
- A faster implementation for Series.str methods (GH2602)
Bug Fixes
- HDFStore tables can now store float32 types correctly (cannot be mixed with float64 however)
- Fixed Google Analytics prefix when specifying request segment (GH2713).
- Function to reset Google Analytics token store so users can recover from improperly setup client secrets (GH2687).
- Fixed groupby bug resulting in segfault when passing in MultiIndex (GH2706)
- Fixed bug where passing a Series with datetime64 values into to_datetime results in bogus output values (GH2699)
- Fixed bug in pattern in HDFStore expressions when pattern is not a valid regex (GH2694)
- Fixed performance issues while aggregating boolean data (GH2692)
- When given a boolean mask key and a Series of new values, Series __setitem__ will now align the incoming values with the original Series (GH2686)
- Fixed MemoryError caused by performing counting sort on sorting MultiIndex levels with a very large number of combinatorial values (GH2684)
- Fixed bug that causes plotting to fail when the index is a DatetimeIndex with a fixed-offset timezone (GH2683)
- Corrected businessday subtraction logic when the offset is more than 5 bdays and the starting date is on a weekend (GH2680)
- Fixed C file parser behavior when the file has more columns than data (GH2668)
- Fixed file reader bug that misaligned columns with data in the presence of an implicit column and a specified usecols value
- DataFrames with numerical or datetime indices are now sorted prior to plotting (GH2609)
- Fixed DataFrame.from_records error when passed columns, index, but empty records (GH2633)
- Several bug fixed for Series operations when dtype is datetime64 (GH2689, GH2629, GH2626)
See the full release notes or issue tracker on GitHub for a complete list.
v0.10.0 (December 17, 2012)¶
This is a major release from 0.9.1 and includes many new features and enhancements along with a large number of bug fixes. There are also a number of important API changes that long-time pandas users should pay close attention to.
File parsing new features¶
The delimited file parsing engine (the guts of read_csv and read_table) has been rewritten from the ground up and now uses a fraction the amount of memory while parsing, while being 40% or more faster in most use cases (in some cases much faster).
There are also many new features:
- Much-improved Unicode handling via the encoding option.
- Column filtering (usecols)
- Dtype specification (dtype argument)
- Ability to specify strings to be recognized as True/False
- Ability to yield NumPy record arrays (as_recarray)
- High performance delim_whitespace option
- Decimal format (e.g. European format) specification
- Easier CSV dialect options: escapechar, lineterminator, quotechar, etc.
- More robust handling of many exceptional kinds of files observed in the wild
API changes¶
Deprecated DataFrame BINOP TimeSeries special case behavior
The default behavior of binary operations between a DataFrame and a Series has always been to align on the DataFrame’s columns and broadcast down the rows, except in the special case that the DataFrame contains time series. Since there are now method for each binary operator enabling you to specify how you want to broadcast, we are phasing out this special case (Zen of Python: Special cases aren’t special enough to break the rules). Here’s what I’m talking about:
In [1]: import pandas as pd
In [2]: df = pd.DataFrame(np.random.randn(6, 4),
...: index=pd.date_range('1/1/2000', periods=6))
...:
In [3]: df
Out[3]:
0 1 2 3
2000-01-01 -0.134024 -0.205969 1.348944 -1.198246
2000-01-02 -1.626124 0.982041 0.059493 -0.460111
2000-01-03 -1.565401 -0.025706 0.942864 2.502156
2000-01-04 -0.302741 0.261551 -0.066342 0.897097
2000-01-05 0.268766 -1.225092 0.582752 -1.490764
2000-01-06 -0.639757 -0.952750 -0.892402 0.505987
[6 rows x 4 columns]
# deprecated now
In [4]: df - df[0]
Out[4]:
2000-01-01 00:00:00 2000-01-02 00:00:00 2000-01-03 00:00:00 \
2000-01-01 NaN NaN NaN
2000-01-02 NaN NaN NaN
2000-01-03 NaN NaN NaN
2000-01-04 NaN NaN NaN
2000-01-05 NaN NaN NaN
2000-01-06 NaN NaN NaN
2000-01-04 00:00:00 2000-01-05 00:00:00 2000-01-06 00:00:00 0 \
2000-01-01 NaN NaN NaN NaN
2000-01-02 NaN NaN NaN NaN
2000-01-03 NaN NaN NaN NaN
2000-01-04 NaN NaN NaN NaN
2000-01-05 NaN NaN NaN NaN
2000-01-06 NaN NaN NaN NaN
1 2 3
2000-01-01 NaN NaN NaN
2000-01-02 NaN NaN NaN
2000-01-03 NaN NaN NaN
2000-01-04 NaN NaN NaN
2000-01-05 NaN NaN NaN
2000-01-06 NaN NaN NaN
[6 rows x 10 columns]
# Change your code to
In [5]: df.sub(df[0], axis=0) # align on axis 0 (rows)
Out[5]:
0 1 2 3
2000-01-01 0 -0.071946 1.482967 -1.064223
2000-01-02 0 2.608165 1.685618 1.166013
2000-01-03 0 1.539695 2.508265 4.067556
2000-01-04 0 0.564293 0.236399 1.199839
2000-01-05 0 -1.493857 0.313986 -1.759530
2000-01-06 0 -0.312993 -0.252645 1.145744
[6 rows x 4 columns]
You will get a deprecation warning in the 0.10.x series, and the deprecated functionality will be removed in 0.11 or later.
Altered resample default behavior
The default time series resample binning behavior of daily D and higher frequencies has been changed to closed='left', label='left'. Lower nfrequencies are unaffected. The prior defaults were causing a great deal of confusion for users, especially resampling data to daily frequency (which labeled the aggregated group with the end of the interval: the next day).
Note:
In [6]: dates = pd.date_range('1/1/2000', '1/5/2000', freq='4h')
In [7]: series = Series(np.arange(len(dates)), index=dates)
In [8]: series
Out[8]:
2000-01-01 00:00:00 0
2000-01-01 04:00:00 1
2000-01-01 08:00:00 2
2000-01-01 12:00:00 3
2000-01-01 16:00:00 4
2000-01-01 20:00:00 5
2000-01-02 00:00:00 6
..
2000-01-04 00:00:00 18
2000-01-04 04:00:00 19
2000-01-04 08:00:00 20
2000-01-04 12:00:00 21
2000-01-04 16:00:00 22
2000-01-04 20:00:00 23
2000-01-05 00:00:00 24
Freq: 4H, dtype: int32
In [9]: series.resample('D', how='sum')
Out[9]:
2000-01-01 15
2000-01-02 51
2000-01-03 87
2000-01-04 123
2000-01-05 24
Freq: D, dtype: int32
# old behavior
In [10]: series.resample('D', how='sum', closed='right', label='right')
Out[10]:
2000-01-01 0
2000-01-02 21
2000-01-03 57
2000-01-04 93
2000-01-05 129
Freq: D, dtype: int32
- Infinity and negative infinity are no longer treated as NA by isnull and notnull. That they every were was a relic of early pandas. This behavior can be re-enabled globally by the mode.use_inf_as_null option:
In [11]: s = pd.Series([1.5, np.inf, 3.4, -np.inf])
In [12]: pd.isnull(s)
Out[12]:
0 False
1 False
2 False
3 False
dtype: bool
In [13]: s.fillna(0)
Out[13]:
0 1.500000
1 inf
2 3.400000
3 -inf
dtype: float64
In [14]: pd.set_option('use_inf_as_null', True)
In [15]: pd.isnull(s)
Out[15]:
0 False
1 True
2 False
3 True
dtype: bool
In [16]: s.fillna(0)
Out[16]:
0 1.5
1 0.0
2 3.4
3 0.0
dtype: float64
In [17]: pd.reset_option('use_inf_as_null')
- Methods with the inplace option now all return None instead of the calling object. E.g. code written like df = df.fillna(0, inplace=True) may stop working. To fix, simply delete the unnecessary variable assignment.
- pandas.merge no longer sorts the group keys (sort=False) by default. This was done for performance reasons: the group-key sorting is often one of the more expensive parts of the computation and is often unnecessary.
- The default column names for a file with no header have been changed to the integers 0 through N - 1. This is to create consistency with the DataFrame constructor with no columns specified. The v0.9.0 behavior (names X0, X1, ...) can be reproduced by specifying prefix='X':
In [18]: data= 'a,b,c\n1,Yes,2\n3,No,4'
In [19]: print(data)
a,b,c
1,Yes,2
3,No,4
In [20]: pd.read_csv(StringIO(data), header=None)
Out[20]:
0 1 2
0 a b c
1 1 Yes 2
2 3 No 4
[3 rows x 3 columns]
In [21]: pd.read_csv(StringIO(data), header=None, prefix='X')
Out[21]:
X0 X1 X2
0 a b c
1 1 Yes 2
2 3 No 4
[3 rows x 3 columns]
- Values like 'Yes' and 'No' are not interpreted as boolean by default, though this can be controlled by new true_values and false_values arguments:
In [22]: print(data)
a,b,c
1,Yes,2
3,No,4
In [23]: pd.read_csv(StringIO(data))
Out[23]:
a b c
0 1 Yes 2
1 3 No 4
[2 rows x 3 columns]
In [24]: pd.read_csv(StringIO(data), true_values=['Yes'], false_values=['No'])
Out[24]:
a b c
0 1 True 2
1 3 False 4
[2 rows x 3 columns]
- The file parsers will not recognize non-string values arising from a converter function as NA if passed in the na_values argument. It’s better to do post-processing using the replace function instead.
- Calling fillna on Series or DataFrame with no arguments is no longer valid code. You must either specify a fill value or an interpolation method:
In [25]: s = Series([np.nan, 1., 2., np.nan, 4])
In [26]: s
Out[26]:
0 NaN
1 1
2 2
3 NaN
4 4
dtype: float64
In [27]: s.fillna(0)
Out[27]:
0 0
1 1
2 2
3 0
4 4
dtype: float64
In [28]: s.fillna(method='pad')
Out[28]:
0 NaN
1 1
2 2
3 2
4 4
dtype: float64
Convenience methods ffill and bfill have been added:
In [29]: s.ffill()
Out[29]:
0 NaN
1 1
2 2
3 2
4 4
dtype: float64
Series.apply will now operate on a returned value from the applied function, that is itself a series, and possibly upcast the result to a DataFrame
In [30]: def f(x): ....: return Series([ x, x**2 ], index = ['x', 'x^2']) ....: In [31]: s = Series(np.random.rand(5)) In [32]: s Out[32]: 0 0.717478 1 0.815199 2 0.452478 3 0.848385 4 0.235477 dtype: float64 In [33]: s.apply(f) Out[33]: x x^2 0 0.717478 0.514775 1 0.815199 0.664550 2 0.452478 0.204737 3 0.848385 0.719757 4 0.235477 0.055449 [5 rows x 2 columns]
New API functions for working with pandas options (GH2097):
- get_option / set_option - get/set the value of an option. Partial names are accepted. - reset_option - reset one or more options to their default value. Partial names are accepted. - describe_option - print a description of one or more options. When called with no arguments. print all registered options.
Note: set_printoptions/ reset_printoptions are now deprecated (but functioning), the print options now live under “display.XYZ”. For example:
In [34]: get_option("display.max_rows") Out[34]: 15
to_string() methods now always return unicode strings (GH2224).
New features¶
Wide DataFrame Printing¶
Instead of printing the summary information, pandas now splits the string representation across multiple rows by default:
In [35]: wide_frame = DataFrame(randn(5, 16))
In [36]: wide_frame
Out[36]:
0 1 2 3 4 5 6 \
0 -0.681624 0.191356 1.180274 -0.834179 0.703043 0.166568 -0.583599
1 0.441522 -0.316864 -0.017062 1.570114 -0.360875 -0.880096 0.235532
2 -0.412451 -0.462580 0.422194 0.288403 -0.487393 -0.777639 0.055865
3 -0.277255 1.331263 0.585174 -0.568825 -0.719412 1.191340 -0.456362
4 -1.642511 0.432560 1.218080 -0.564705 -0.581790 0.286071 0.048725
7 8 9 10 11 12 13 \
0 -1.201796 -1.422811 -0.882554 1.209871 -0.941235 0.863067 -0.336232
1 0.207232 -1.983857 -1.702547 -1.621234 -0.906840 1.014601 -0.475108
2 1.383381 0.085638 0.246392 0.965887 0.246354 -0.727728 -0.094414
3 0.089931 0.776079 0.752889 -1.195795 -1.425911 -0.548829 0.774225
4 1.002440 1.276582 0.054399 0.241963 -0.471786 0.314510 -0.059986
14 15
0 -0.976847 0.033862
1 -0.358944 1.262942
2 -0.276854 0.158399
3 0.740501 1.510263
4 -2.069319 -1.115104
[5 rows x 16 columns]
The old behavior of printing out summary information can be achieved via the ‘expand_frame_repr’ print option:
In [37]: pd.set_option('expand_frame_repr', False)
In [38]: wide_frame
Out[38]:
0 1 2 3 4 5 6 7 8 9 10 11 12 13 14 15
0 -0.681624 0.191356 1.180274 -0.834179 0.703043 0.166568 -0.583599 -1.201796 -1.422811 -0.882554 1.209871 -0.941235 0.863067 -0.336232 -0.976847 0.033862
1 0.441522 -0.316864 -0.017062 1.570114 -0.360875 -0.880096 0.235532 0.207232 -1.983857 -1.702547 -1.621234 -0.906840 1.014601 -0.475108 -0.358944 1.262942
2 -0.412451 -0.462580 0.422194 0.288403 -0.487393 -0.777639 0.055865 1.383381 0.085638 0.246392 0.965887 0.246354 -0.727728 -0.094414 -0.276854 0.158399
3 -0.277255 1.331263 0.585174 -0.568825 -0.719412 1.191340 -0.456362 0.089931 0.776079 0.752889 -1.195795 -1.425911 -0.548829 0.774225 0.740501 1.510263
4 -1.642511 0.432560 1.218080 -0.564705 -0.581790 0.286071 0.048725 1.002440 1.276582 0.054399 0.241963 -0.471786 0.314510 -0.059986 -2.069319 -1.115104
[5 rows x 16 columns]
The width of each line can be changed via ‘line_width’ (80 by default):
In [39]: pd.set_option('line_width', 40)
line_width has been deprecated, use display.width instead (currently both are
identical)
In [40]: wide_frame
Out[40]:
0 1 2 \
0 -0.681624 0.191356 1.180274
1 0.441522 -0.316864 -0.017062
2 -0.412451 -0.462580 0.422194
3 -0.277255 1.331263 0.585174
4 -1.642511 0.432560 1.218080
3 4 5 \
0 -0.834179 0.703043 0.166568
1 1.570114 -0.360875 -0.880096
2 0.288403 -0.487393 -0.777639
3 -0.568825 -0.719412 1.191340
4 -0.564705 -0.581790 0.286071
6 7 8 \
0 -0.583599 -1.201796 -1.422811
1 0.235532 0.207232 -1.983857
2 0.055865 1.383381 0.085638
3 -0.456362 0.089931 0.776079
4 0.048725 1.002440 1.276582
9 10 11 \
0 -0.882554 1.209871 -0.941235
1 -1.702547 -1.621234 -0.906840
2 0.246392 0.965887 0.246354
3 0.752889 -1.195795 -1.425911
4 0.054399 0.241963 -0.471786
12 13 14 \
0 0.863067 -0.336232 -0.976847
1 1.014601 -0.475108 -0.358944
2 -0.727728 -0.094414 -0.276854
3 -0.548829 0.774225 0.740501
4 0.314510 -0.059986 -2.069319
15
0 0.033862
1 1.262942
2 0.158399
3 1.510263
4 -1.115104
[5 rows x 16 columns]
Updated PyTables Support¶
Docs for PyTables Table format & several enhancements to the api. Here is a taste of what to expect.
In [41]: store = HDFStore('store.h5')
In [42]: df = DataFrame(randn(8, 3), index=date_range('1/1/2000', periods=8),
....: columns=['A', 'B', 'C'])
....:
In [43]: df
Out[43]:
A B C
2000-01-01 -0.369325 -1.502617 -0.376280
2000-01-02 0.511936 -0.116412 -0.625256
2000-01-03 -0.550627 1.261433 -0.552429
2000-01-04 1.695803 -1.025917 -0.910942
2000-01-05 0.426805 -0.131749 0.432600
2000-01-06 0.044671 -0.341265 1.844536
2000-01-07 -2.036047 0.000830 -0.955697
2000-01-08 -0.898872 -0.725411 0.059904
[8 rows x 3 columns]
# appending data frames
In [44]: df1 = df[0:4]
In [45]: df2 = df[4:]
In [46]: store.append('df', df1)
In [47]: store.append('df', df2)
In [48]: store
Out[48]:
<class 'pandas.io.pytables.HDFStore'>
File path: store.h5
/df frame_table (typ->appendable,nrows->8,ncols->3,indexers->[index])
# selecting the entire store
In [49]: store.select('df')
Out[49]:
A B C
2000-01-01 -0.369325 -1.502617 -0.376280
2000-01-02 0.511936 -0.116412 -0.625256
2000-01-03 -0.550627 1.261433 -0.552429
2000-01-04 1.695803 -1.025917 -0.910942
2000-01-05 0.426805 -0.131749 0.432600
2000-01-06 0.044671 -0.341265 1.844536
2000-01-07 -2.036047 0.000830 -0.955697
2000-01-08 -0.898872 -0.725411 0.059904
[8 rows x 3 columns]
In [50]: wp = Panel(randn(2, 5, 4), items=['Item1', 'Item2'],
....: major_axis=date_range('1/1/2000', periods=5),
....: minor_axis=['A', 'B', 'C', 'D'])
....:
In [51]: wp
Out[51]:
<class 'pandas.core.panel.Panel'>
Dimensions: 2 (items) x 5 (major_axis) x 4 (minor_axis)
Items axis: Item1 to Item2
Major_axis axis: 2000-01-01 00:00:00 to 2000-01-05 00:00:00
Minor_axis axis: A to D
# storing a panel
In [52]: store.append('wp',wp)
# selecting via A QUERY
In [53]: store.select('wp',
....: [ Term('major_axis>20000102'), Term('minor_axis', '=', ['A','B']) ])
....:
Out[53]:
<class 'pandas.core.panel.Panel'>
Dimensions: 2 (items) x 3 (major_axis) x 2 (minor_axis)
Items axis: Item1 to Item2
Major_axis axis: 2000-01-03 00:00:00 to 2000-01-05 00:00:00
Minor_axis axis: A to B
# removing data from tables
In [54]: store.remove('wp', Term('major_axis>20000103'))
Out[54]: 8
In [55]: store.select('wp')
Out[55]:
<class 'pandas.core.panel.Panel'>
Dimensions: 2 (items) x 3 (major_axis) x 4 (minor_axis)
Items axis: Item1 to Item2
Major_axis axis: 2000-01-01 00:00:00 to 2000-01-03 00:00:00
Minor_axis axis: A to D
# deleting a store
In [56]: del store['df']
In [57]: store
Out[57]:
<class 'pandas.io.pytables.HDFStore'>
File path: store.h5
/wp wide_table (typ->appendable,nrows->12,ncols->2,indexers->[major_axis,minor_axis])
Enhancements
added ability to hierarchical keys
In [58]: store.put('foo/bar/bah', df) In [59]: store.append('food/orange', df) In [60]: store.append('food/apple', df) In [61]: store Out[61]: <class 'pandas.io.pytables.HDFStore'> File path: store.h5 /foo/bar/bah frame (shape->[8,3]) /food/apple frame_table (typ->appendable,nrows->8,ncols->3,indexers->[index]) /food/orange frame_table (typ->appendable,nrows->8,ncols->3,indexers->[index]) /wp wide_table (typ->appendable,nrows->12,ncols->2,indexers->[major_axis,minor_axis]) # remove all nodes under this level In [62]: store.remove('food') In [63]: store Out[63]: <class 'pandas.io.pytables.HDFStore'> File path: store.h5 /foo/bar/bah frame (shape->[8,3]) /wp wide_table (typ->appendable,nrows->12,ncols->2,indexers->[major_axis,minor_axis])
added mixed-dtype support!
In [64]: df['string'] = 'string' In [65]: df['int'] = 1 In [66]: store.append('df',df) In [67]: df1 = store.select('df') In [68]: df1 Out[68]: A B C string int 2000-01-01 -0.369325 -1.502617 -0.376280 string 1 2000-01-02 0.511936 -0.116412 -0.625256 string 1 2000-01-03 -0.550627 1.261433 -0.552429 string 1 2000-01-04 1.695803 -1.025917 -0.910942 string 1 2000-01-05 0.426805 -0.131749 0.432600 string 1 2000-01-06 0.044671 -0.341265 1.844536 string 1 2000-01-07 -2.036047 0.000830 -0.955697 string 1 2000-01-08 -0.898872 -0.725411 0.059904 string 1 [8 rows x 5 columns] In [69]: df1.get_dtype_counts() Out[69]: float64 3 int64 1 object 1 dtype: int64
performance improvments on table writing
support for arbitrarily indexed dimensions
SparseSeries now has a density property (GH2384)
enable Series.str.strip/lstrip/rstrip methods to take an input argument to strip arbitrary characters (GH2411)
implement value_vars in melt to limit values to certain columns and add melt to pandas namespace (GH2412)
Bug Fixes
- added Term method of specifying where conditions (GH1996).
- del store['df'] now call store.remove('df') for store deletion
- deleting of consecutive rows is much faster than before
- min_itemsize parameter can be specified in table creation to force a minimum size for indexing columns (the previous implementation would set the column size based on the first append)
- indexing support via create_table_index (requires PyTables >= 2.3) (GH698).
- appending on a store would fail if the table was not first created via put
- fixed issue with missing attributes after loading a pickled dataframe (GH2431)
- minor change to select and remove: require a table ONLY if where is also provided (and not None)
Compatibility
0.10 of HDFStore is backwards compatible for reading tables created in a prior version of pandas, however, query terms using the prior (undocumented) methodology are unsupported. You must read in the entire file and write it out using the new format to take advantage of the updates.
N Dimensional Panels (Experimental)¶
Adding experimental support for Panel4D and factory functions to create n-dimensional named panels. Docs for NDim. Here is a taste of what to expect.
In [70]: p4d = Panel4D(randn(2, 2, 5, 4), ....: labels=['Label1','Label2'], ....: items=['Item1', 'Item2'], ....: major_axis=date_range('1/1/2000', periods=5), ....: minor_axis=['A', 'B', 'C', 'D']) ....: In [71]: p4d Out[71]: <class 'pandas.core.panelnd.Panel4D'> Dimensions: 2 (labels) x 2 (items) x 5 (major_axis) x 4 (minor_axis) Labels axis: Label1 to Label2 Items axis: Item1 to Item2 Major_axis axis: 2000-01-01 00:00:00 to 2000-01-05 00:00:00 Minor_axis axis: A to D
See the full release notes or issue tracker on GitHub for a complete list.
v0.9.1 (November 14, 2012)¶
This is a bugfix release from 0.9.0 and includes several new features and enhancements along with a large number of bug fixes. The new features include by-column sort order for DataFrame and Series, improved NA handling for the rank method, masking functions for DataFrame, and intraday time-series filtering for DataFrame.
New features¶
Series.sort, DataFrame.sort, and DataFrame.sort_index can now be specified in a per-column manner to support multiple sort orders (GH928)
In [1]: df = DataFrame(np.random.randint(0, 2, (6, 3)), columns=['A', 'B', 'C']) In [2]: df.sort(['A', 'B'], ascending=[1, 0]) Out[2]: A B C 0 0 1 0 2 0 0 1 1 1 1 1 5 1 1 0 3 1 0 0 4 1 0 1 [6 rows x 3 columns]DataFrame.rank now supports additional argument values for the na_option parameter so missing values can be assigned either the largest or the smallest rank (GH1508, GH2159)
In [3]: df = DataFrame(np.random.randn(6, 3), columns=['A', 'B', 'C']) In [4]: df.ix[2:4] = np.nan In [5]: df.rank() Out[5]: A B C 0 3 2 1 1 1 3 3 2 NaN NaN NaN 3 NaN NaN NaN 4 NaN NaN NaN 5 2 1 2 [6 rows x 3 columns] In [6]: df.rank(na_option='top') Out[6]: A B C 0 6 5 4 1 4 6 6 2 2 2 2 3 2 2 2 4 2 2 2 5 5 4 5 [6 rows x 3 columns] In [7]: df.rank(na_option='bottom') Out[7]: A B C 0 3 2 1 1 1 3 3 2 5 5 5 3 5 5 5 4 5 5 5 5 2 1 2 [6 rows x 3 columns]DataFrame has new where and mask methods to select values according to a given boolean mask (GH2109, GH2151)
DataFrame currently supports slicing via a boolean vector the same length as the DataFrame (inside the []). The returned DataFrame has the same number of columns as the original, but is sliced on its index.
In [8]: df = DataFrame(np.random.randn(5, 3), columns = ['A','B','C']) In [9]: df Out[9]: A B C 0 -0.187239 -1.703664 0.613136 1 -0.948528 0.505346 0.017228 2 -2.391256 1.207381 0.853174 3 0.124213 -0.625597 -1.211224 4 -0.476548 0.649425 0.004610 [5 rows x 3 columns] In [10]: df[df['A'] > 0] Out[10]: A B C 3 0.124213 -0.625597 -1.211224 [1 rows x 3 columns]If a DataFrame is sliced with a DataFrame based boolean condition (with the same size as the original DataFrame), then a DataFrame the same size (index and columns) as the original is returned, with elements that do not meet the boolean condition as NaN. This is accomplished via the new method DataFrame.where. In addition, where takes an optional other argument for replacement.
In [11]: df[df>0] Out[11]: A B C 0 NaN NaN 0.613136 1 NaN 0.505346 0.017228 2 NaN 1.207381 0.853174 3 0.124213 NaN NaN 4 NaN 0.649425 0.004610 [5 rows x 3 columns] In [12]: df.where(df>0) Out[12]: A B C 0 NaN NaN 0.613136 1 NaN 0.505346 0.017228 2 NaN 1.207381 0.853174 3 0.124213 NaN NaN 4 NaN 0.649425 0.004610 [5 rows x 3 columns] In [13]: df.where(df>0,-df) Out[13]: A B C 0 0.187239 1.703664 0.613136 1 0.948528 0.505346 0.017228 2 2.391256 1.207381 0.853174 3 0.124213 0.625597 1.211224 4 0.476548 0.649425 0.004610 [5 rows x 3 columns]Furthermore, where now aligns the input boolean condition (ndarray or DataFrame), such that partial selection with setting is possible. This is analagous to partial setting via .ix (but on the contents rather than the axis labels)
In [14]: df2 = df.copy() In [15]: df2[ df2[1:4] > 0 ] = 3 In [16]: df2 Out[16]: A B C 0 -0.187239 -1.703664 0.613136 1 -0.948528 3.000000 3.000000 2 -2.391256 3.000000 3.000000 3 3.000000 -0.625597 -1.211224 4 -0.476548 0.649425 0.004610 [5 rows x 3 columns]DataFrame.mask is the inverse boolean operation of where.
In [17]: df.mask(df<=0) Out[17]: A B C 0 NaN NaN 0.613136 1 NaN 0.505346 0.017228 2 NaN 1.207381 0.853174 3 0.124213 NaN NaN 4 NaN 0.649425 0.004610 [5 rows x 3 columns]Enable referencing of Excel columns by their column names (GH1936)
In [18]: xl = ExcelFile('data/test.xls') In [19]: xl.parse('Sheet1', index_col=0, parse_dates=True, ....: parse_cols='A:D') ....: Out[19]: A B C 2000-01-03 0.980269 3.685731 -0.364217 2000-01-04 1.047916 -0.041232 -0.161812 2000-01-05 0.498581 0.731168 -0.537677 2000-01-06 1.120202 1.567621 0.003641 2000-01-07 -0.487094 0.571455 -1.611639 2000-01-10 0.836649 0.246462 0.588543 2000-01-11 -0.157161 1.340307 1.195778 [7 rows x 3 columns]Added option to disable pandas-style tick locators and formatters using series.plot(x_compat=True) or pandas.plot_params[‘x_compat’] = True (GH2205)
Existing TimeSeries methods at_time and between_time were added to DataFrame (GH2149)
DataFrame.dot can now accept ndarrays (GH2042)
DataFrame.drop now supports non-unique indexes (GH2101)
Panel.shift now supports negative periods (GH2164)
DataFrame now support unary ~ operator (GH2110)
API changes¶
Upsampling data with a PeriodIndex will result in a higher frequency TimeSeries that spans the original time window
In [20]: prng = period_range('2012Q1', periods=2, freq='Q') In [21]: s = Series(np.random.randn(len(prng)), prng) In [22]: s.resample('M') Out[22]: 2012-01 -0.508759 2012-02 NaN 2012-03 NaN 2012-04 -0.599515 2012-05 NaN 2012-06 NaN Freq: M, dtype: float64Period.end_time now returns the last nanosecond in the time interval (GH2124, GH2125, GH1764)
In [23]: p = Period('2012') In [24]: p.end_time Out[24]: Timestamp('2012-12-31 23:59:59.999999999')File parsers no longer coerce to float or bool for columns that have custom converters specified (GH2184)
In [25]: data = 'A,B,C\n00001,001,5\n00002,002,6' In [26]: read_csv(StringIO(data), converters={'A' : lambda x: x.strip()}) Out[26]: A B C 0 00001 1 5 1 00002 2 6 [2 rows x 3 columns]
See the full release notes or issue tracker on GitHub for a complete list.
v0.9.0 (October 7, 2012)¶
This is a major release from 0.8.1 and includes several new features and enhancements along with a large number of bug fixes. New features include vectorized unicode encoding/decoding for Series.str, to_latex method to DataFrame, more flexible parsing of boolean values, and enabling the download of options data from Yahoo! Finance.
New features¶
- Add encode and decode for unicode handling to vectorized string processing methods in Series.str (GH1706)
- Add DataFrame.to_latex method (GH1735)
- Add convenient expanding window equivalents of all rolling_* ops (GH1785)
- Add Options class to pandas.io.data for fetching options data from Yahoo! Finance (GH1748, GH1739)
- More flexible parsing of boolean values (Yes, No, TRUE, FALSE, etc) (GH1691, GH1295)
- Add level parameter to Series.reset_index
- TimeSeries.between_time can now select times across midnight (GH1871)
- Series constructor can now handle generator as input (GH1679)
- DataFrame.dropna can now take multiple axes (tuple/list) as input (GH924)
- Enable skip_footer parameter in ExcelFile.parse (GH1843)
API changes¶
- The default column names when header=None and no columns names passed to functions like read_csv has changed to be more Pythonic and amenable to attribute access:
In [1]: data = '0,0,1\n1,1,0\n0,1,0'
In [2]: df = read_csv(StringIO(data), header=None)
In [3]: df
Out[3]:
0 1 2
0 0 0 1
1 1 1 0
2 0 1 0
[3 rows x 3 columns]
- Creating a Series from another Series, passing an index, will cause reindexing to happen inside rather than treating the Series like an ndarray. Technically improper usages like Series(df[col1], index=df[col2]) that worked before “by accident” (this was never intended) will lead to all NA Series in some cases. To be perfectly clear:
In [4]: s1 = Series([1, 2, 3])
In [5]: s1
Out[5]:
0 1
1 2
2 3
dtype: int64
In [6]: s2 = Series(s1, index=['foo', 'bar', 'baz'])
In [7]: s2
Out[7]:
foo NaN
bar NaN
baz NaN
dtype: float64
- Deprecated day_of_year API removed from PeriodIndex, use dayofyear (GH1723)
- Don’t modify NumPy suppress printoption to True at import time
- The internal HDF5 data arrangement for DataFrames has been transposed. Legacy files will still be readable by HDFStore (GH1834, GH1824)
- Legacy cruft removed: pandas.stats.misc.quantileTS
- Use ISO8601 format for Period repr: monthly, daily, and on down (GH1776)
- Empty DataFrame columns are now created as object dtype. This will prevent a class of TypeErrors that was occurring in code where the dtype of a column would depend on the presence of data or not (e.g. a SQL query having results) (GH1783)
- Setting parts of DataFrame/Panel using ix now aligns input Series/DataFrame (GH1630)
- first and last methods in GroupBy no longer drop non-numeric columns (GH1809)
- Resolved inconsistencies in specifying custom NA values in text parser. na_values of type dict no longer override default NAs unless keep_default_na is set to false explicitly (GH1657)
- DataFrame.dot will not do data alignment, and also work with Series (GH1915)
See the full release notes or issue tracker on GitHub for a complete list.
v0.8.1 (July 22, 2012)¶
This release includes a few new features, performance enhancements, and over 30 bug fixes from 0.8.0. New features include notably NA friendly string processing functionality and a series of new plot types and options.
New features¶
- Add vectorized string processing methods accessible via Series.str (GH620)
- Add option to disable adjustment in EWMA (GH1584)
- Radviz plot (GH1566)
- Parallel coordinates plot
- Bootstrap plot
- Per column styles and secondary y-axis plotting (GH1559)
- New datetime converters millisecond plotting (GH1599)
- Add option to disable “sparse” display of hierarchical indexes (GH1538)
- Series/DataFrame’s set_index method can append levels to an existing Index/MultiIndex (GH1569, GH1577)
Performance improvements¶
- Improved implementation of rolling min and max (thanks to Bottleneck !)
- Add accelerated 'median' GroupBy option (GH1358)
- Significantly improve the performance of parsing ISO8601-format date strings with DatetimeIndex or to_datetime (GH1571)
- Improve the performance of GroupBy on single-key aggregations and use with Categorical types
- Significant datetime parsing performance improvments
v0.8.0 (June 29, 2012)¶
This is a major release from 0.7.3 and includes extensive work on the time series handling and processing infrastructure as well as a great deal of new functionality throughout the library. It includes over 700 commits from more than 20 distinct authors. Most pandas 0.7.3 and earlier users should not experience any issues upgrading, but due to the migration to the NumPy datetime64 dtype, there may be a number of bugs and incompatibilities lurking. Lingering incompatibilities will be fixed ASAP in a 0.8.1 release if necessary. See the full release notes or issue tracker on GitHub for a complete list.
Support for non-unique indexes¶
All objects can now work with non-unique indexes. Data alignment / join operations work according to SQL join semantics (including, if application, index duplication in many-to-many joins)
NumPy datetime64 dtype and 1.6 dependency¶
Time series data are now represented using NumPy’s datetime64 dtype; thus, pandas 0.8.0 now requires at least NumPy 1.6. It has been tested and verified to work with the development version (1.7+) of NumPy as well which includes some significant user-facing API changes. NumPy 1.6 also has a number of bugs having to do with nanosecond resolution data, so I recommend that you steer clear of NumPy 1.6’s datetime64 API functions (though limited as they are) and only interact with this data using the interface that pandas provides.
See the end of the 0.8.0 section for a “porting” guide listing potential issues for users migrating legacy codebases from pandas 0.7 or earlier to 0.8.0.
Bug fixes to the 0.7.x series for legacy NumPy < 1.6 users will be provided as they arise. There will be no more further development in 0.7.x beyond bug fixes.
Time series changes and improvements¶
Note
With this release, legacy scikits.timeseries users should be able to port their code to use pandas.
Note
See documentation for overview of pandas timeseries API.
- New datetime64 representation speeds up join operations and data alignment, reduces memory usage, and improve serialization / deserialization performance significantly over datetime.datetime
- High performance and flexible resample method for converting from high-to-low and low-to-high frequency. Supports interpolation, user-defined aggregation functions, and control over how the intervals and result labeling are defined. A suite of high performance Cython/C-based resampling functions (including Open-High-Low-Close) have also been implemented.
- Revamp of frequency aliases and support for frequency shortcuts like ‘15min’, or ‘1h30min’
- New DatetimeIndex class supports both fixed frequency and irregular time series. Replaces now deprecated DateRange class
- New PeriodIndex and Period classes for representing time spans and performing calendar logic, including the 12 fiscal quarterly frequencies <timeseries.quarterly>. This is a partial port of, and a substantial enhancement to, elements of the scikits.timeseries codebase. Support for conversion between PeriodIndex and DatetimeIndex
- New Timestamp data type subclasses datetime.datetime, providing the same interface while enabling working with nanosecond-resolution data. Also provides easy time zone conversions.
- Enhanced support for time zones. Add tz_convert and tz_lcoalize methods to TimeSeries and DataFrame. All timestamps are stored as UTC; Timestamps from DatetimeIndex objects with time zone set will be localized to localtime. Time zone conversions are therefore essentially free. User needs to know very little about pytz library now; only time zone names as as strings are required. Time zone-aware timestamps are equal if and only if their UTC timestamps match. Operations between time zone-aware time series with different time zones will result in a UTC-indexed time series.
- Time series string indexing conveniences / shortcuts: slice years, year and month, and index values with strings
- Enhanced time series plotting; adaptation of scikits.timeseries matplotlib-based plotting code
- New date_range, bdate_range, and period_range factory functions
- Robust frequency inference function infer_freq and inferred_freq property of DatetimeIndex, with option to infer frequency on construction of DatetimeIndex
- to_datetime function efficiently parses array of strings to DatetimeIndex. DatetimeIndex will parse array or list of strings to datetime64
- Optimized support for datetime64-dtype data in Series and DataFrame columns
- New NaT (Not-a-Time) type to represent NA in timestamp arrays
- Optimize Series.asof for looking up “as of” values for arrays of timestamps
- Milli, Micro, Nano date offset objects
- Can index time series with datetime.time objects to select all data at particular time of day (TimeSeries.at_time) or between two times (TimeSeries.between_time)
- Add tshift method for leading/lagging using the frequency (if any) of the index, as opposed to a naive lead/lag using shift
Other new features¶
- New cut and qcut functions (like R’s cut function) for computing a categorical variable from a continuous variable by binning values either into value-based (cut) or quantile-based (qcut) bins
- Rename Factor to Categorical and add a number of usability features
- Add limit argument to fillna/reindex
- More flexible multiple function application in GroupBy, and can pass list (name, function) tuples to get result in particular order with given names
- Add flexible replace method for efficiently substituting values
- Enhanced read_csv/read_table for reading time series data and converting multiple columns to dates
- Add comments option to parser functions: read_csv, etc.
- Add :ref`dayfirst <io.dayfirst>` option to parser functions for parsing international DD/MM/YYYY dates
- Allow the user to specify the CSV reader dialect to control quoting etc.
- Handling thousands separators in read_csv to improve integer parsing.
- Enable unstacking of multiple levels in one shot. Alleviate pivot_table bugs (empty columns being introduced)
- Move to klib-based hash tables for indexing; better performance and less memory usage than Python’s dict
- Add first, last, min, max, and prod optimized GroupBy functions
- New ordered_merge function
- Add flexible comparison instance methods eq, ne, lt, gt, etc. to DataFrame, Series
- Improve scatter_matrix plotting function and add histogram or kernel density estimates to diagonal
- Add ‘kde’ plot option for density plots
- Support for converting DataFrame to R data.frame through rpy2
- Improved support for complex numbers in Series and DataFrame
- Add pct_change method to all data structures
- Add max_colwidth configuration option for DataFrame console output
- Interpolate Series values using index values
- Can select multiple columns from GroupBy
- Add update methods to Series/DataFrame for updating values in place
- Add any and all method to DataFrame
New plotting methods¶
Series.plot now supports a secondary_y option:
In [1]: plt.figure()
Out[1]: <matplotlib.figure.Figure at 0x9f964fcc>
In [2]: fx['FR'].plot(style='g')
Out[2]: <matplotlib.axes._subplots.AxesSubplot at 0x9f946fcc>
In [3]: fx['IT'].plot(style='k--', secondary_y=True)
Out[3]: <matplotlib.axes._subplots.AxesSubplot at 0x9f96444c>

Vytautas Jancauskas, the 2012 GSOC participant, has added many new plot types. For example, 'kde' is a new option:
In [4]: s = Series(np.concatenate((np.random.randn(1000),
...: np.random.randn(1000) * 0.5 + 3)))
...:
In [5]: plt.figure()
Out[5]: <matplotlib.figure.Figure at 0x9f69fdec>
In [6]: s.hist(normed=True, alpha=0.2)
Out[6]: <matplotlib.axes._subplots.AxesSubplot at 0x9e45f5ac>
In [7]: s.plot(kind='kde')
Out[7]: <matplotlib.axes._subplots.AxesSubplot at 0x9e45f5ac>

See the plotting page for much more.
Other API changes¶
- Deprecation of offset, time_rule, and timeRule arguments names in time series functions. Warnings will be printed until pandas 0.9 or 1.0.
Potential porting issues for pandas <= 0.7.3 users¶
The major change that may affect you in pandas 0.8.0 is that time series indexes use NumPy’s datetime64 data type instead of dtype=object arrays of Python’s built-in datetime.datetime objects. DateRange has been replaced by DatetimeIndex but otherwise behaved identically. But, if you have code that converts DateRange or Index objects that used to contain datetime.datetime values to plain NumPy arrays, you may have bugs lurking with code using scalar values because you are handing control over to NumPy:
In [8]: import datetime
In [9]: rng = date_range('1/1/2000', periods=10)
In [10]: rng[5]
Out[10]: Timestamp('2000-01-06 00:00:00', offset='D')
In [11]: isinstance(rng[5], datetime.datetime)
Out[11]: True
In [12]: rng_asarray = np.asarray(rng)
In [13]: scalar_val = rng_asarray[5]
In [14]: type(scalar_val)
Out[14]: numpy.datetime64
pandas’s Timestamp object is a subclass of datetime.datetime that has nanosecond support (the nanosecond field store the nanosecond value between 0 and 999). It should substitute directly into any code that used datetime.datetime values before. Thus, I recommend not casting DatetimeIndex to regular NumPy arrays.
If you have code that requires an array of datetime.datetime objects, you have a couple of options. First, the asobject property of DatetimeIndex produces an array of Timestamp objects:
In [15]: stamp_array = rng.asobject
In [16]: stamp_array
Out[16]:
Index([2000-01-01 00:00:00, 2000-01-02 00:00:00, 2000-01-03 00:00:00,
2000-01-04 00:00:00, 2000-01-05 00:00:00, 2000-01-06 00:00:00,
2000-01-07 00:00:00, 2000-01-08 00:00:00, 2000-01-09 00:00:00,
2000-01-10 00:00:00],
dtype='object')
In [17]: stamp_array[5]
Out[17]: Timestamp('2000-01-06 00:00:00', offset='D')
To get an array of proper datetime.datetime objects, use the to_pydatetime method:
In [18]: dt_array = rng.to_pydatetime()
In [19]: dt_array
Out[19]:
array([datetime.datetime(2000, 1, 1, 0, 0),
datetime.datetime(2000, 1, 2, 0, 0),
datetime.datetime(2000, 1, 3, 0, 0),
datetime.datetime(2000, 1, 4, 0, 0),
datetime.datetime(2000, 1, 5, 0, 0),
datetime.datetime(2000, 1, 6, 0, 0),
datetime.datetime(2000, 1, 7, 0, 0),
datetime.datetime(2000, 1, 8, 0, 0),
datetime.datetime(2000, 1, 9, 0, 0),
datetime.datetime(2000, 1, 10, 0, 0)], dtype=object)
In [20]: dt_array[5]
Out[20]: datetime.datetime(2000, 1, 6, 0, 0)
matplotlib knows how to handle datetime.datetime but not Timestamp objects. While I recommend that you plot time series using TimeSeries.plot, you can either use to_pydatetime or register a converter for the Timestamp type. See matplotlib documentation for more on this.
Warning
There are bugs in the user-facing API with the nanosecond datetime64 unit in NumPy 1.6. In particular, the string version of the array shows garbage values, and conversion to dtype=object is similarly broken.
In [21]: rng = date_range('1/1/2000', periods=10)
In [22]: rng
Out[22]:
DatetimeIndex(['2000-01-01', '2000-01-02', '2000-01-03', '2000-01-04',
'2000-01-05', '2000-01-06', '2000-01-07', '2000-01-08',
'2000-01-09', '2000-01-10'],
dtype='datetime64[ns]', freq='D')
In [23]: np.asarray(rng)
Out[23]:
array(['2000-01-01T01:00:00.000000000+0100',
'2000-01-02T01:00:00.000000000+0100',
'2000-01-03T01:00:00.000000000+0100',
'2000-01-04T01:00:00.000000000+0100',
'2000-01-05T01:00:00.000000000+0100',
'2000-01-06T01:00:00.000000000+0100',
'2000-01-07T01:00:00.000000000+0100',
'2000-01-08T01:00:00.000000000+0100',
'2000-01-09T01:00:00.000000000+0100',
'2000-01-10T01:00:00.000000000+0100'], dtype='datetime64[ns]')
In [24]: converted = np.asarray(rng, dtype=object)
In [25]: converted[5]
Out[25]: 947116800000000000L
Trust me: don’t panic. If you are using NumPy 1.6 and restrict your interaction with datetime64 values to pandas’s API you will be just fine. There is nothing wrong with the data-type (a 64-bit integer internally); all of the important data processing happens in pandas and is heavily tested. I strongly recommend that you do not work directly with datetime64 arrays in NumPy 1.6 and only use the pandas API.
Support for non-unique indexes: In the latter case, you may have code inside a try:... catch: block that failed due to the index not being unique. In many cases it will no longer fail (some method like append still check for uniqueness unless disabled). However, all is not lost: you can inspect index.is_unique and raise an exception explicitly if it is False or go to a different code branch.
v.0.7.3 (April 12, 2012)¶
This is a minor release from 0.7.2 and fixes many minor bugs and adds a number of nice new features. There are also a couple of API changes to note; these should not affect very many users, and we are inclined to call them “bug fixes” even though they do constitute a change in behavior. See the full release notes or issue tracker on GitHub for a complete list.
New features¶
- New fixed width file reader, read_fwf
- New scatter_matrix function for making a scatter plot matrix
from pandas.tools.plotting import scatter_matrix
scatter_matrix(df, alpha=0.2)
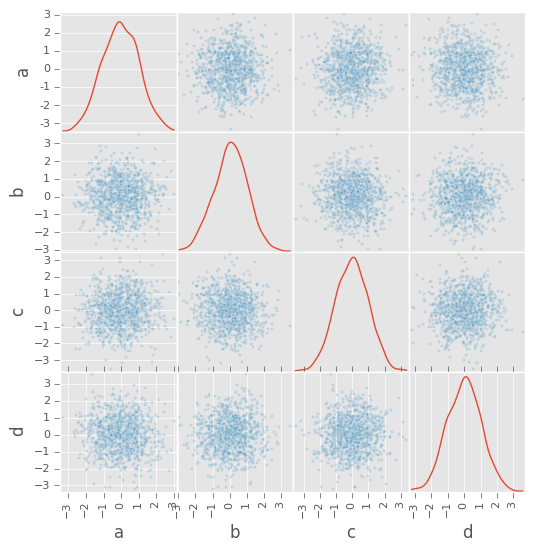
- Add stacked argument to Series and DataFrame’s plot method for stacked bar plots.
df.plot(kind='bar', stacked=True)
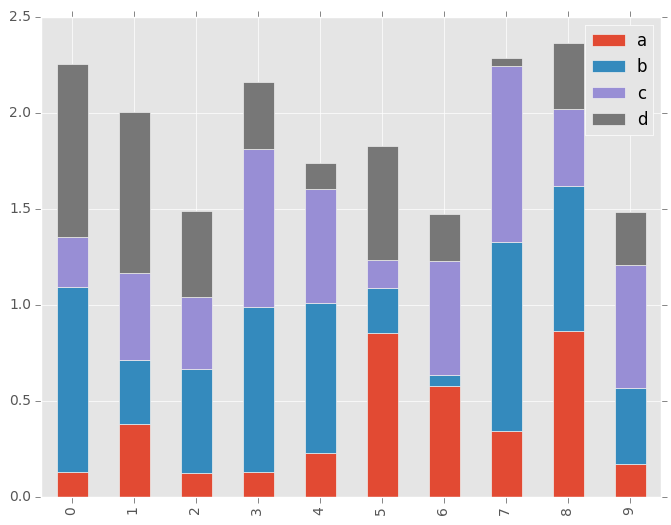
df.plot(kind='barh', stacked=True)
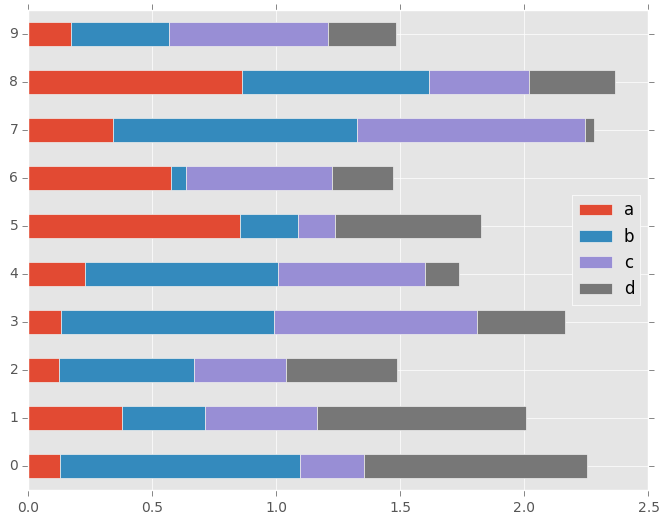
- Add log x and y scaling options to DataFrame.plot and Series.plot
- Add kurt methods to Series and DataFrame for computing kurtosis
NA Boolean Comparison API Change¶
Reverted some changes to how NA values (represented typically as NaN or None) are handled in non-numeric Series:
In [1]: series = Series(['Steve', np.nan, 'Joe'])
In [2]: series == 'Steve'
Out[2]:
0 True
1 False
2 False
dtype: bool
In [3]: series != 'Steve'
Out[3]:
0 False
1 True
2 True
dtype: bool
In comparisons, NA / NaN will always come through as False except with != which is True. Be very careful with boolean arithmetic, especially negation, in the presence of NA data. You may wish to add an explicit NA filter into boolean array operations if you are worried about this:
In [4]: mask = series == 'Steve'
In [5]: series[mask & series.notnull()]
Out[5]:
0 Steve
dtype: object
While propagating NA in comparisons may seem like the right behavior to some users (and you could argue on purely technical grounds that this is the right thing to do), the evaluation was made that propagating NA everywhere, including in numerical arrays, would cause a large amount of problems for users. Thus, a “practicality beats purity” approach was taken. This issue may be revisited at some point in the future.
Other API Changes¶
When calling apply on a grouped Series, the return value will also be a Series, to be more consistent with the groupby behavior with DataFrame:
In [6]: df = DataFrame({'A' : ['foo', 'bar', 'foo', 'bar',
...: 'foo', 'bar', 'foo', 'foo'],
...: 'B' : ['one', 'one', 'two', 'three',
...: 'two', 'two', 'one', 'three'],
...: 'C' : np.random.randn(8), 'D' : np.random.randn(8)})
...:
In [7]: df
Out[7]:
A B C D
0 foo one -0.032419 0.237288
1 bar one -1.581534 0.514679
2 foo two -0.912061 -1.488101
3 bar three 0.209500 1.018514
4 foo two -0.675890 -1.488840
5 bar two 0.055228 -1.355434
6 foo one -1.079181 0.661979
7 foo three -1.631882 0.708958
[8 rows x 4 columns]
In [8]: grouped = df.groupby('A')['C']
In [9]: grouped.describe()
Out[9]:
A
bar count 3.000000
mean -0.438936
std 0.992521
min -1.581534
25% -0.763153
50% 0.055228
75% 0.132364
...
foo mean -0.866287
std 0.584196
min -1.631882
25% -1.079181
50% -0.912061
75% -0.675890
max -0.032419
dtype: float64
In [10]: grouped.apply(lambda x: x.order()[-2:]) # top 2 values
Out[10]:
A
bar 5 0.055228
3 0.209500
foo 4 -0.675890
0 -0.032419
dtype: float64
v.0.7.2 (March 16, 2012)¶
This release targets bugs in 0.7.1, and adds a few minor features.
New features¶
- Add additional tie-breaking methods in DataFrame.rank (GH874)
- Add ascending parameter to rank in Series, DataFrame (GH875)
- Add coerce_float option to DataFrame.from_records (GH893)
- Add sort_columns parameter to allow unsorted plots (GH918)
- Enable column access via attributes on GroupBy (GH882)
- Can pass dict of values to DataFrame.fillna (GH661)
- Can select multiple hierarchical groups by passing list of values in .ix (GH134)
- Add axis option to DataFrame.fillna (GH174)
- Add level keyword to drop for dropping values from a level (GH159)
v.0.7.1 (February 29, 2012)¶
This release includes a few new features and addresses over a dozen bugs in 0.7.0.
New features¶
- Add to_clipboard function to pandas namespace for writing objects to the system clipboard (GH774)
- Add itertuples method to DataFrame for iterating through the rows of a dataframe as tuples (GH818)
- Add ability to pass fill_value and method to DataFrame and Series align method (GH806, GH807)
- Add fill_value option to reindex, align methods (GH784)
- Enable concat to produce DataFrame from Series (GH787)
- Add between method to Series (GH802)
- Add HTML representation hook to DataFrame for the IPython HTML notebook (GH773)
- Support for reading Excel 2007 XML documents using openpyxl
v.0.7.0 (February 9, 2012)¶
New features¶
- New unified merge function for efficiently performing full gamut of database / relational-algebra operations. Refactored existing join methods to use the new infrastructure, resulting in substantial performance gains (GH220, GH249, GH267)
- New unified concatenation function for concatenating Series, DataFrame or Panel objects along an axis. Can form union or intersection of the other axes. Improves performance of Series.append and DataFrame.append (GH468, GH479, GH273)
- Can pass multiple DataFrames to DataFrame.append to concatenate (stack) and multiple Series to Series.append too
- Can pass list of dicts (e.g., a list of JSON objects) to DataFrame constructor (GH526)
- You can now set multiple columns in a DataFrame via __getitem__, useful for transformation (GH342)
- Handle differently-indexed output values in DataFrame.apply (GH498)
In [1]: df = DataFrame(randn(10, 4))
In [2]: df.apply(lambda x: x.describe())
Out[2]:
0 1 2 3
count 10.000000 10.000000 10.000000 10.000000
mean 0.058434 0.008207 0.449898 -0.064109
std 0.959629 1.126010 0.784723 0.650405
min -1.819334 -1.607906 -1.275249 -1.200953
25% -0.365166 -0.973095 0.100811 -0.369865
50% 0.116057 0.179112 0.718606 -0.057095
75% 0.616168 0.807868 0.851809 0.237069
max 1.387310 1.521442 1.437656 1.051356
[8 rows x 4 columns]
- Add reorder_levels method to Series and DataFrame (GH534)
- Add dict-like get function to DataFrame and Panel (GH521)
- Add DataFrame.iterrows method for efficiently iterating through the rows of a DataFrame
- Add DataFrame.to_panel with code adapted from LongPanel.to_long
- Add reindex_axis method added to DataFrame
- Add level option to binary arithmetic functions on DataFrame and Series
- Add level option to the reindex and align methods on Series and DataFrame for broadcasting values across a level (GH542, GH552, others)
- Add attribute-based item access to Panel and add IPython completion (GH563)
- Add logy option to Series.plot for log-scaling on the Y axis
- Add index and header options to DataFrame.to_string
- Can pass multiple DataFrames to DataFrame.join to join on index (GH115)
- Can pass multiple Panels to Panel.join (GH115)
- Added justify argument to DataFrame.to_string to allow different alignment of column headers
- Add sort option to GroupBy to allow disabling sorting of the group keys for potential speedups (GH595)
- Can pass MaskedArray to Series constructor (GH563)
- Add Panel item access via attributes and IPython completion (GH554)
- Implement DataFrame.lookup, fancy-indexing analogue for retrieving values given a sequence of row and column labels (GH338)
- Can pass a list of functions to aggregate with groupby on a DataFrame, yielding an aggregated result with hierarchical columns (GH166)
- Can call cummin and cummax on Series and DataFrame to get cumulative minimum and maximum, respectively (GH647)
- value_range added as utility function to get min and max of a dataframe (GH288)
- Added encoding argument to read_csv, read_table, to_csv and from_csv for non-ascii text (GH717)
- Added abs method to pandas objects
- Added crosstab function for easily computing frequency tables
- Added isin method to index objects
- Added level argument to xs method of DataFrame.
API Changes to integer indexing¶
One of the potentially riskiest API changes in 0.7.0, but also one of the most important, was a complete review of how integer indexes are handled with regard to label-based indexing. Here is an example:
In [3]: s = Series(randn(10), index=range(0, 20, 2))
In [4]: s
Out[4]:
0 0.041867
2 1.503116
4 -0.841265
6 -1.578003
8 -0.273728
10 1.755240
12 -0.705788
14 -0.351950
16 1.507974
18 0.419219
dtype: float64
In [5]: s[0]
Out[5]: 0.041867372914288915
In [6]: s[2]
Out[6]: 1.5031163945003796
In [7]: s[4]
Out[7]: -0.84126511615728994
This is all exactly identical to the behavior before. However, if you ask for a key not contained in the Series, in versions 0.6.1 and prior, Series would fall back on a location-based lookup. This now raises a KeyError:
In [2]: s[1]
KeyError: 1
This change also has the same impact on DataFrame:
In [3]: df = DataFrame(randn(8, 4), index=range(0, 16, 2))
In [4]: df
0 1 2 3
0 0.88427 0.3363 -0.1787 0.03162
2 0.14451 -0.1415 0.2504 0.58374
4 -1.44779 -0.9186 -1.4996 0.27163
6 -0.26598 -2.4184 -0.2658 0.11503
8 -0.58776 0.3144 -0.8566 0.61941
10 0.10940 -0.7175 -1.0108 0.47990
12 -1.16919 -0.3087 -0.6049 -0.43544
14 -0.07337 0.3410 0.0424 -0.16037
In [5]: df.ix[3]
KeyError: 3
In order to support purely integer-based indexing, the following methods have been added:
Method | Description |
---|---|
Series.iget_value(i) | Retrieve value stored at location i |
Series.iget(i) | Alias for iget_value |
DataFrame.irow(i) | Retrieve the i-th row |
DataFrame.icol(j) | Retrieve the j-th column |
DataFrame.iget_value(i, j) | Retrieve the value at row i and column j |
API tweaks regarding label-based slicing¶
Label-based slicing using ix now requires that the index be sorted (monotonic) unless both the start and endpoint are contained in the index:
In [8]: s = Series(randn(6), index=list('gmkaec'))
In [9]: s
Out[9]:
g 0.647633
m -0.147670
k -0.759803
a -0.757308
e -1.921164
c -1.093529
dtype: float64
Then this is OK:
In [10]: s.ix['k':'e']
Out[10]:
k -0.759803
a -0.757308
e -1.921164
dtype: float64
But this is not:
In [12]: s.ix['b':'h']
KeyError 'b'
If the index had been sorted, the “range selection” would have been possible:
In [11]: s2 = s.sort_index()
In [12]: s2
Out[12]:
a -0.757308
c -1.093529
e -1.921164
g 0.647633
k -0.759803
m -0.147670
dtype: float64
In [13]: s2.ix['b':'h']
Out[13]:
c -1.093529
e -1.921164
g 0.647633
dtype: float64
Changes to Series [] operator¶
As as notational convenience, you can pass a sequence of labels or a label slice to a Series when getting and setting values via [] (i.e. the __getitem__ and __setitem__ methods). The behavior will be the same as passing similar input to ix except in the case of integer indexing:
In [14]: s = Series(randn(6), index=list('acegkm'))
In [15]: s
Out[15]:
a -0.592157
c -0.715074
e -0.616193
g -0.335468
k -0.392051
m -0.189537
dtype: float64
In [16]: s[['m', 'a', 'c', 'e']]
Out[16]:
m -0.189537
a -0.592157
c -0.715074
e -0.616193
dtype: float64
In [17]: s['b':'l']
Out[17]:
c -0.715074
e -0.616193
g -0.335468
k -0.392051
dtype: float64
In [18]: s['c':'k']
Out[18]:
c -0.715074
e -0.616193
g -0.335468
k -0.392051
dtype: float64
In the case of integer indexes, the behavior will be exactly as before (shadowing ndarray):
In [19]: s = Series(randn(6), index=range(0, 12, 2))
In [20]: s[[4, 0, 2]]
Out[20]:
4 0.319635
0 0.886170
2 -1.125894
dtype: float64
In [21]: s[1:5]
Out[21]:
2 -1.125894
4 0.319635
6 0.998222
8 0.091743
dtype: float64
If you wish to do indexing with sequences and slicing on an integer index with label semantics, use ix.
Other API Changes¶
- The deprecated LongPanel class has been completely removed
- If Series.sort is called on a column of a DataFrame, an exception will now be raised. Before it was possible to accidentally mutate a DataFrame’s column by doing df[col].sort() instead of the side-effect free method df[col].order() (GH316)
- Miscellaneous renames and deprecations which will (harmlessly) raise FutureWarning
- drop added as an optional parameter to DataFrame.reset_index (GH699)
Performance improvements¶
- Cythonized GroupBy aggregations no longer presort the data, thus achieving a significant speedup (GH93). GroupBy aggregations with Python functions significantly sped up by clever manipulation of the ndarray data type in Cython (GH496).
- Better error message in DataFrame constructor when passed column labels don’t match data (GH497)
- Substantially improve performance of multi-GroupBy aggregation when a Python function is passed, reuse ndarray object in Cython (GH496)
- Can store objects indexed by tuples and floats in HDFStore (GH492)
- Don’t print length by default in Series.to_string, add length option (GH489)
- Improve Cython code for multi-groupby to aggregate without having to sort the data (GH93)
- Improve MultiIndex reindexing speed by storing tuples in the MultiIndex, test for backwards unpickling compatibility
- Improve column reindexing performance by using specialized Cython take function
- Further performance tweaking of Series.__getitem__ for standard use cases
- Avoid Index dict creation in some cases (i.e. when getting slices, etc.), regression from prior versions
- Friendlier error message in setup.py if NumPy not installed
- Use common set of NA-handling operations (sum, mean, etc.) in Panel class also (GH536)
- Default name assignment when calling reset_index on DataFrame with a regular (non-hierarchical) index (GH476)
- Use Cythonized groupers when possible in Series/DataFrame stat ops with level parameter passed (GH545)
- Ported skiplist data structure to C to speed up rolling_median by about 5-10x in most typical use cases (GH374)
v.0.6.1 (December 13, 2011)¶
New features¶
- Can append single rows (as Series) to a DataFrame
- Add Spearman and Kendall rank correlation options to Series.corr and DataFrame.corr (GH428)
- Added get_value and set_value methods to Series, DataFrame, and Panel for very low-overhead access (>2x faster in many cases) to scalar elements (GH437, GH438). set_value is capable of producing an enlarged object.
- Add PyQt table widget to sandbox (GH435)
- DataFrame.align can accept Series arguments and an axis option (GH461)
- Implement new SparseArray and SparseList data structures. SparseSeries now derives from SparseArray (GH463)
- Better console printing options (GH453)
- Implement fast data ranking for Series and DataFrame, fast versions of scipy.stats.rankdata (GH428)
- Implement DataFrame.from_items alternate constructor (GH444)
- DataFrame.convert_objects method for inferring better dtypes for object columns (GH302)
- Add rolling_corr_pairwise function for computing Panel of correlation matrices (GH189)
- Add margins option to pivot_table for computing subgroup aggregates (GH114)
- Add Series.from_csv function (GH482)
- Can pass DataFrame/DataFrame and DataFrame/Series to rolling_corr/rolling_cov (GH #462)
- MultiIndex.get_level_values can accept the level name
Performance improvements¶
- Improve memory usage of DataFrame.describe (do not copy data unnecessarily) (PR #425)
- Optimize scalar value lookups in the general case by 25% or more in Series and DataFrame
- Fix performance regression in cross-sectional count in DataFrame, affecting DataFrame.dropna speed
- Column deletion in DataFrame copies no data (computes views on blocks) (GH #158)
v.0.6.0 (November 25, 2011)¶
New Features¶
- Added melt function to pandas.core.reshape
- Added level parameter to group by level in Series and DataFrame descriptive statistics (GH313)
- Added head and tail methods to Series, analogous to to DataFrame (GH296)
- Added Series.isin function which checks if each value is contained in a passed sequence (GH289)
- Added float_format option to Series.to_string
- Added skip_footer (GH291) and converters (GH343) options to read_csv and read_table
- Added drop_duplicates and duplicated functions for removing duplicate DataFrame rows and checking for duplicate rows, respectively (GH319)
- Implemented operators ‘&’, ‘|’, ‘^’, ‘-‘ on DataFrame (GH347)
- Added Series.mad, mean absolute deviation
- Added QuarterEnd DateOffset (GH321)
- Added dot to DataFrame (GH65)
- Added orient option to Panel.from_dict (GH359, GH301)
- Added orient option to DataFrame.from_dict
- Added passing list of tuples or list of lists to DataFrame.from_records (GH357)
- Added multiple levels to groupby (GH103)
- Allow multiple columns in by argument of DataFrame.sort_index (GH92, GH362)
- Added fast get_value and put_value methods to DataFrame (GH360)
- Added cov instance methods to Series and DataFrame (GH194, GH362)
- Added kind='bar' option to DataFrame.plot (GH348)
- Added idxmin and idxmax to Series and DataFrame (GH286)
- Added read_clipboard function to parse DataFrame from clipboard (GH300)
- Added nunique function to Series for counting unique elements (GH297)
- Made DataFrame constructor use Series name if no columns passed (GH373)
- Support regular expressions in read_table/read_csv (GH364)
- Added DataFrame.to_html for writing DataFrame to HTML (GH387)
- Added support for MaskedArray data in DataFrame, masked values converted to NaN (GH396)
- Added DataFrame.boxplot function (GH368)
- Can pass extra args, kwds to DataFrame.apply (GH376)
- Implement DataFrame.join with vector on argument (GH312)
- Added legend boolean flag to DataFrame.plot (GH324)
- Can pass multiple levels to stack and unstack (GH370)
- Can pass multiple values columns to pivot_table (GH381)
- Use Series name in GroupBy for result index (GH363)
- Added raw option to DataFrame.apply for performance if only need ndarray (GH309)
- Added proper, tested weighted least squares to standard and panel OLS (GH303)
Performance Enhancements¶
- VBENCH Cythonized cache_readonly, resulting in substantial micro-performance enhancements throughout the codebase (GH361)
- VBENCH Special Cython matrix iterator for applying arbitrary reduction operations with 3-5x better performance than np.apply_along_axis (GH309)
- VBENCH Improved performance of MultiIndex.from_tuples
- VBENCH Special Cython matrix iterator for applying arbitrary reduction operations
- VBENCH + DOCUMENT Add raw option to DataFrame.apply for getting better performance when
- VBENCH Faster cythonized count by level in Series and DataFrame (GH341)
- VBENCH? Significant GroupBy performance enhancement with multiple keys with many “empty” combinations
- VBENCH New Cython vectorized function map_infer speeds up Series.apply and Series.map significantly when passed elementwise Python function, motivated by (GH355)
- VBENCH Significantly improved performance of Series.order, which also makes np.unique called on a Series faster (GH327)
- VBENCH Vastly improved performance of GroupBy on axes with a MultiIndex (GH299)
v.0.5.0 (October 24, 2011)¶
New Features¶
- Added DataFrame.align method with standard join options
- Added parse_dates option to read_csv and read_table methods to optionally try to parse dates in the index columns
- Added nrows, chunksize, and iterator arguments to read_csv and read_table. The last two return a new TextParser class capable of lazily iterating through chunks of a flat file (GH242)
- Added ability to join on multiple columns in DataFrame.join (GH214)
- Added private _get_duplicates function to Index for identifying duplicate values more easily (ENH5c)
- Added column attribute access to DataFrame.
- Added Python tab completion hook for DataFrame columns. (GH233, GH230)
- Implemented Series.describe for Series containing objects (GH241)
- Added inner join option to DataFrame.join when joining on key(s) (GH248)
- Implemented selecting DataFrame columns by passing a list to __getitem__ (GH253)
- Implemented & and | to intersect / union Index objects, respectively (GH261)
- Added pivot_table convenience function to pandas namespace (GH234)
- Implemented Panel.rename_axis function (GH243)
- DataFrame will show index level names in console output (GH334)
- Implemented Panel.take
- Added set_eng_float_format for alternate DataFrame floating point string formatting (ENH61)
- Added convenience set_index function for creating a DataFrame index from its existing columns
- Implemented groupby hierarchical index level name (GH223)
- Added support for different delimiters in DataFrame.to_csv (GH244)
- TODO: DOCS ABOUT TAKE METHODS
Performance Enhancements¶
- VBENCH Major performance improvements in file parsing functions read_csv and read_table
- VBENCH Added Cython function for converting tuples to ndarray very fast. Speeds up many MultiIndex-related operations
- VBENCH Refactored merging / joining code into a tidy class and disabled unnecessary computations in the float/object case, thus getting about 10% better performance (GH211)
- VBENCH Improved speed of DataFrame.xs on mixed-type DataFrame objects by about 5x, regression from 0.3.0 (GH215)
- VBENCH With new DataFrame.align method, speeding up binary operations between differently-indexed DataFrame objects by 10-25%.
- VBENCH Significantly sped up conversion of nested dict into DataFrame (GH212)
- VBENCH Significantly speed up DataFrame __repr__ and count on large mixed-type DataFrame objects
v.0.4.3 through v0.4.1 (September 25 - October 9, 2011)¶
New Features¶
- Added Python 3 support using 2to3 (GH200)
- Added name attribute to Series, now prints as part of Series.__repr__
- Added instance methods isnull and notnull to Series (GH209, GH203)
- Added Series.align method for aligning two series with choice of join method (ENH56)
- Added method get_level_values to MultiIndex (GH188)
- Set values in mixed-type DataFrame objects via .ix indexing attribute (GH135)
- Added new DataFrame methods get_dtype_counts and property dtypes (ENHdc)
- Added ignore_index option to DataFrame.append to stack DataFrames (ENH1b)
- read_csv tries to sniff delimiters using csv.Sniffer (GH146)
- read_csv can read multiple columns into a MultiIndex; DataFrame’s to_csv method writes out a corresponding MultiIndex (GH151)
- DataFrame.rename has a new copy parameter to rename a DataFrame in place (ENHed)
- Enable unstacking by name (GH142)
- Enable sortlevel to work by level (GH141)
Performance Enhancements¶
- Altered binary operations on differently-indexed SparseSeries objects to use the integer-based (dense) alignment logic which is faster with a larger number of blocks (GH205)
- Wrote faster Cython data alignment / merging routines resulting in substantial speed increases
- Improved performance of isnull and notnull, a regression from v0.3.0 (GH187)
- Refactored code related to DataFrame.join so that intermediate aligned copies of the data in each DataFrame argument do not need to be created. Substantial performance increases result (GH176)
- Substantially improved performance of generic Index.intersection and Index.union
- Implemented BlockManager.take resulting in significantly faster take performance on mixed-type DataFrame objects (GH104)
- Improved performance of Series.sort_index
- Significant groupby performance enhancement: removed unnecessary integrity checks in DataFrame internals that were slowing down slicing operations to retrieve groups
- Optimized _ensure_index function resulting in performance savings in type-checking Index objects
- Wrote fast time series merging / joining methods in Cython. Will be integrated later into DataFrame.join and related functions