What’s New¶
These are new features and improvements of note in each release.
v0.8.0 (TBD June, 2012)¶
This is a major release from 0.7.3 and includes extensive work on the time series handling and processing infrastructure as well as a great deal of new functionality throughout the library. It includes over 700 commits from more than 20 distinct authors. Most pandas 0.7.3 and earlier users should not experience any issues upgrading, but due to the migration to the NumPy datetime64 dtype, there may be a number of bugs and incompatibilities lurking. Lingering incompatibilities will be fixed ASAP in a 0.8.1 release if necessary. See the full release notes or issue tracker on GitHub for a complete list.
Support for non-unique indexes¶
All objects can now work with non-unique indexes. Data alignment / join operations work according to SQL join semantics (including, if application, index duplication in many-to-many joins)
NumPy datetime64 dtype and 1.6 dependency¶
Time series data are now represented using NumPy’s datetime64 dtype; thus, pandas 0.8.0 now requires at least NumPy 1.6. It has been tested and verified to work with the development version (1.7+) of NumPy as well which includes some significant user-facing API changes. NumPy 1.6 also has a number of bugs having to do with nanosecond resolution data, so I recommend that you steer clear of NumPy 1.6’s datetime64 API functions (though limited as they are) and only interact with this data using the interface that pandas provides.
See the end of the 0.8.0 section for a “porting” guide listing potential issues for users migrating legacy codebases from pandas 0.7 or earlier to 0.8.0.
Bug fixes to the 0.7.x series for legacy NumPy < 1.6 users will be provided as they arise. There will be no more further development in 0.7.x beyond bug fixes.
Time series changes and improvements¶
Note
With this release, legacy scikits.timeseries users should be able to port their code to use pandas.
Note
See documentation for overview of pandas timeseries API.
- New datetime64 representation speeds up join operations and data alignment, reduces memory usage, and improve serialization / deserialization performance significantly over datetime.datetime
- High performance and flexible resample method for converting from high-to-low and low-to-high frequency. Supports interpolation, user-defined aggregation functions, and control over how the intervals and result labeling are defined. A suite of high performance Cython/C-based resampling functions (including Open-High-Low-Close) have also been implemented.
- Revamp of frequency aliases and support for frequency shortcuts like ‘15min’, or ‘1h30min’
- New DatetimeIndex class supports both fixed frequency and irregular time series. Replaces now deprecated DateRange class
- New PeriodIndex and Period classes for representing time spans and performing calendar logic, including the 12 fiscal quarterly frequencies <timeseries.quarterly>. This is a partial port of, and a substantial enhancement to, elements of the scikits.timeseries codebase. Support for conversion between PeriodIndex and DatetimeIndex
- New Timestamp data type subclasses datetime.datetime, providing the same interface while enabling working with nanosecond-resolution data. Also provides easy time zone conversions.
- Enhanced support for time zones. Add tz_convert and tz_lcoalize methods to TimeSeries and DataFrame. All timestamps are stored as UTC; Timestamps from DatetimeIndex objects with time zone set will be localized to localtime. Time zone conversions are therefore essentially free. User needs to know very little about pytz library now; only time zone names as as strings are required. Time zone-aware timestamps are equal if and only if their UTC timestamps match. Operations between time zone-aware time series with different time zones will result in a UTC-indexed time series.
- Time series string indexing conveniences / shortcuts: slice years, year and month, and index values with strings
- Enhanced time series plotting; adaptation of scikits.timeseries matplotlib-based plotting code
- New date_range, bdate_range, and period_range factory functions
- Robust frequency inference function infer_freq and inferred_freq property of DatetimeIndex, with option to infer frequency on construction of DatetimeIndex
- to_datetime function efficiently parses array of strings to DatetimeIndex. DatetimeIndex will parse array or list of strings to datetime64
- Optimized support for datetime64-dtype data in Series and DataFrame columns
- New NaT (Not-a-Time) type to represent NA in timestamp arrays
- Optimize Series.asof for looking up “as of” values for arrays of timestamps
- Milli, Micro, Nano date offset objects
- Can index time series with datetime.time objects to select all data at particular time of day (TimeSeries.at_time) or between two times (TimeSeries.between_time)
- Add tshift method for leading/lagging using the frequency (if any) of the index, as opposed to a naive lead/lag using shift
Other new features¶
- New cut and qcut functions (like R’s cut function) for computing a categorical variable from a continuous variable by binning values either into value-based (cut) or quantile-based (qcut) bins
- Rename Factor to Categorical and add a number of usability features
- Add limit argument to fillna/reindex
- More flexible multiple function application in GroupBy, and can pass list (name, function) tuples to get result in particular order with given names
- Add flexible replace method for efficiently substituting values
- Enhanced read_csv/read_table for reading time series data and converting multiple columns to dates
- Add comments option to parser functions: read_csv, etc.
- Add :ref`dayfirst <io.dayfirst>` option to parser functions for parsing international DD/MM/YYYY dates
- Allow the user to specify the CSV reader dialect to control quoting etc.
- Handling thousands separators in read_csv to improve integer parsing.
- Enable unstacking of multiple levels in one shot. Alleviate pivot_table bugs (empty columns being introduced)
- Move to klib-based hash tables for indexing; better performance and less memory usage than Python’s dict
- Add first, last, min, max, and prod optimized GroupBy functions
- New ordered_merge function
- Add flexible comparison instance methods eq, ne, lt, gt, etc. to DataFrame, Series
- Improve scatter_matrix plotting function and add histogram or kernel density estimates to diagonal
- Add ‘kde’ plot option for density plots
- Support for converting DataFrame to R data.frame through rpy2
- Improved support for complex numbers in Series and DataFrame
- Add pct_change method to all data structures
- Add max_colwidth configuration option for DataFrame console output
- Interpolate Series values using index values
- Can select multiple columns from GroupBy
- Add update methods to Series/DataFrame for updating values in place
- Add any and ``all method to DataFrame
New plotting methods¶
Series.plot now supports a secondary_y option:
In [1239]: plt.figure()
Out[1239]: <matplotlib.figure.Figure at 0x124502d90>
In [1240]: fx['FR'].plot(style='g')
---------------------------------------------------------------------------
NameError Traceback (most recent call last)
<ipython-input-1240-3bfc79e08766> in <module>()
----> 1 fx['FR'].plot(style='g')
NameError: name 'fx' is not defined
In [1241]: fx['IT'].plot(style='k--', secondary_y=True)
---------------------------------------------------------------------------
NameError Traceback (most recent call last)
<ipython-input-1241-d77d6aa06988> in <module>()
----> 1 fx['IT'].plot(style='k--', secondary_y=True)
NameError: name 'fx' is not defined
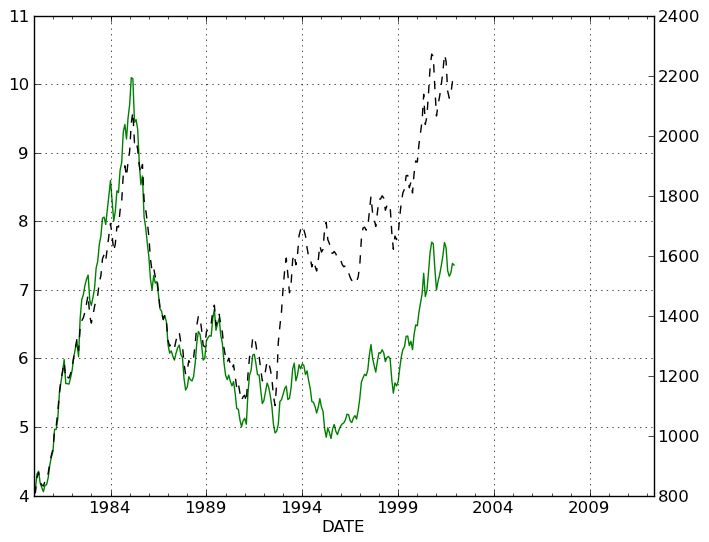
Vytautas Jancauskas, the 2012 GSOC participant, has added many new plot types. For example, 'kde' is a new option:
In [1242]: s = Series(np.concatenate((np.random.randn(1000),
......: np.random.randn(1000) * 0.5 + 3)))
......:
In [1243]: plt.figure()
Out[1243]: <matplotlib.figure.Figure at 0x124521790>
In [1244]: s.hist(normed=True, alpha=0.2)
Out[1244]: <matplotlib.axes.AxesSubplot at 0x124521e50>
In [1245]: s.plot(kind='kde')
Out[1245]: <matplotlib.axes.AxesSubplot at 0x124521e50>
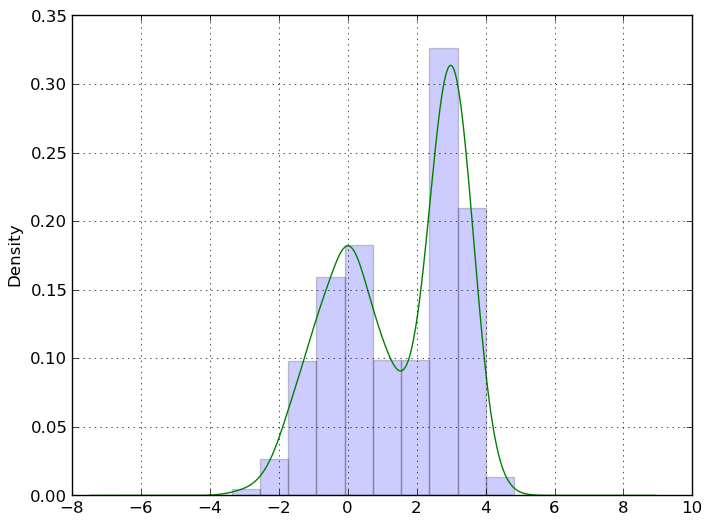
See the plotting page for much more.
Other API changes¶
- Deprecation of offset, time_rule, and timeRule arguments names in time series functions. Warnings will be printed until pandas 0.9 or 1.0.
Potential porting issues for pandas <= 0.7.3 users¶
The major change that may affect you in pandas 0.8.0 is that time series indexes use NumPy’s datetime64 data type instead of dtype=object arrays of Python’s built-in datetime.datetime objects. DateRange has been replaced by DatetimeIndex but otherwise behaved identically. But, if you have code that converts DateRange or Index objects that used to contain datetime.datetime values to plain NumPy arrays, you may have bugs lurking with code using scalar values because you are handing control over to NumPy:
In [1246]: import datetime
In [1247]: rng = date_range('1/1/2000', periods=10)
In [1248]: rng[5]
Out[1248]: <Timestamp: 2000-01-06 00:00:00>
In [1249]: isinstance(rng[5], datetime.datetime)
Out[1249]: True
In [1250]: rng_asarray = np.asarray(rng)
In [1251]: scalar_val = rng_asarray[5]
In [1252]: type(scalar_val)
Out[1252]: numpy.datetime64
pandas’s Timestamp object is a subclass of datetime.datetime that has nanosecond support (the nanosecond field store the nanosecond value between 0 and 999). It should substitute directly into any code that used datetime.datetime values before. Thus, I recommend not casting DatetimeIndex to regular NumPy arrays.
If you have code that requires an array of datetime.datetime objects, you have a couple of options. First, the asobject property of DatetimeIndex produces an array of Timestamp objects:
In [1253]: stamp_array = rng.asobject
In [1254]: stamp_array
Out[1254]:
Index([2000-01-01 00:00:00, 2000-01-02 00:00:00, 2000-01-03 00:00:00,
2000-01-04 00:00:00, 2000-01-05 00:00:00, 2000-01-06 00:00:00,
2000-01-07 00:00:00, 2000-01-08 00:00:00, 2000-01-09 00:00:00,
2000-01-10 00:00:00], dtype=object)
In [1255]: stamp_array[5]
Out[1255]: <Timestamp: 2000-01-06 00:00:00>
To get an array of proper datetime.datetime objects, use the to_pydatetime method:
In [1256]: dt_array = rng.to_pydatetime()
In [1257]: dt_array
Out[1257]:
array([2000-01-01 00:00:00, 2000-01-02 00:00:00, 2000-01-03 00:00:00,
2000-01-04 00:00:00, 2000-01-05 00:00:00, 2000-01-06 00:00:00,
2000-01-07 00:00:00, 2000-01-08 00:00:00, 2000-01-09 00:00:00,
2000-01-10 00:00:00], dtype=object)
In [1258]: dt_array[5]
Out[1258]: datetime.datetime(2000, 1, 6, 0, 0)
matplotlib knows how to handle datetime.datetime but not Timestamp objects. While I recommend that you plot time series using TimeSeries.plot, you can either use to_pydatetime or register a converter for the Timestamp type. See matplotlib documentation for more on this.
Warning
There are bugs in the user-facing API with the nanosecond datetime64 unit in NumPy 1.6. In particular, the string version of the array shows garbage values, and conversion to dtype=object is similarly broken.
In [1259]: rng = date_range('1/1/2000', periods=10)
In [1260]: rng
Out[1260]:
<class 'pandas.tseries.index.DatetimeIndex'>
[2000-01-01 00:00:00, ..., 2000-01-10 00:00:00]
Length: 10, Freq: D, Timezone: None
In [1261]: np.asarray(rng)
Out[1261]:
array([1970-01-11 184:00:00, 1970-01-11 208:00:00, 1970-01-11 232:00:00,
1970-01-11 00:00:00, 1970-01-11 24:00:00, 1970-01-11 48:00:00,
1970-01-11 72:00:00, 1970-01-11 96:00:00, 1970-01-11 120:00:00,
1970-01-11 144:00:00], dtype=datetime64[ns])
In [1262]: converted = np.asarray(rng, dtype=object)
In [1263]: converted[5]
Out[1263]: datetime.datetime(1970, 1, 11, 48, 0)
Trust me: don’t panic. If you are using NumPy 1.6 and restrict your interaction with datetime64 values to pandas’s API you will be just fine. There is nothing wrong with the data-type (a 64-bit integer internally); all of the important data processing happens in pandas and is heavily tested. I strongly recommend that you do not work directly with datetime64 arrays in NumPy 1.6 and only use the pandas API.
Support for non-unique indexes: In the latter case, you may have code inside a try:... catch: block that failed due to the index not being unique. In many cases it will no longer fail (some method like append still check for uniqueness unless disabled). However, all is not lost: you can inspect index.is_unique and raise an exception explicitly if it is False or go to a different code branch.
v.0.7.3 (April 12, 2012)¶
This is a minor release from 0.7.2 and fixes many minor bugs and adds a number of nice new features. There are also a couple of API changes to note; these should not affect very many users, and we are inclined to call them “bug fixes” even though they do constitute a change in behavior. See the full release notes or issue tracker on GitHub for a complete list.
New features¶
- New fixed width file reader, read_fwf
- New scatter_matrix function for making a scatter plot matrix
from pandas.tools.plotting import scatter_matrix
scatter_matrix(df, alpha=0.2)
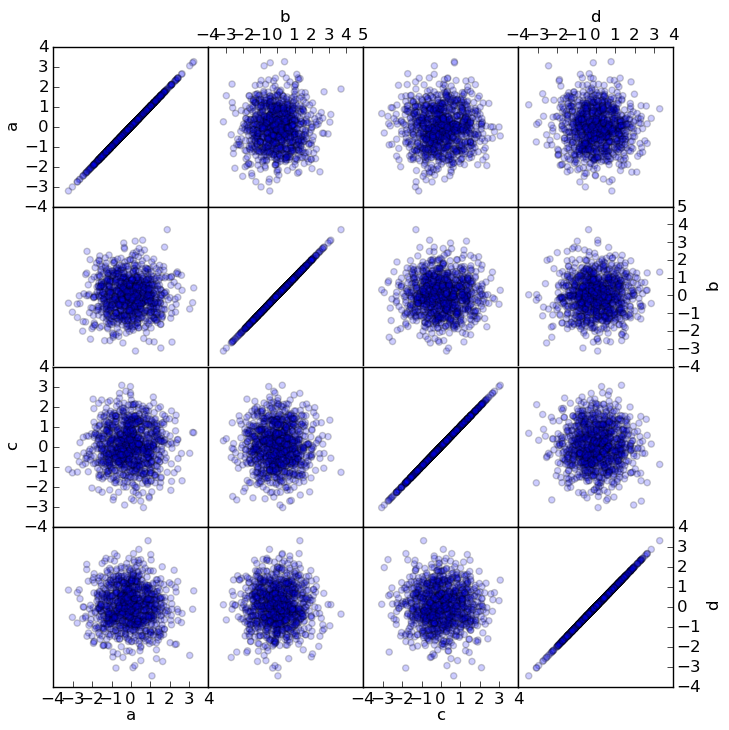
- Add stacked argument to Series and DataFrame’s plot method for stacked bar plots.
df.plot(kind='bar', stacked=True)
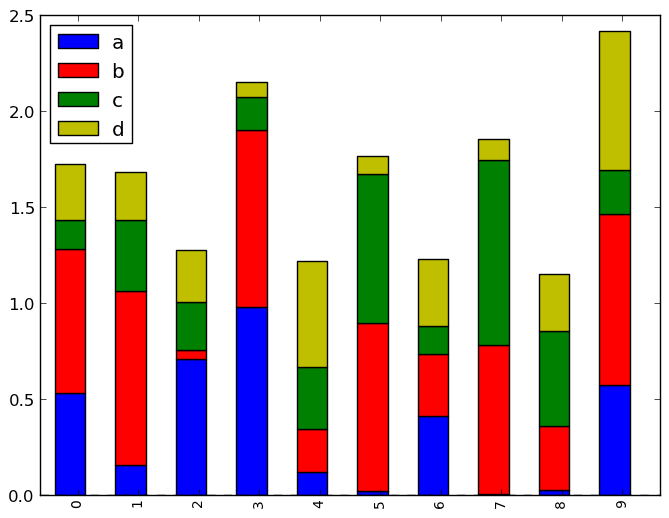
df.plot(kind='barh', stacked=True)
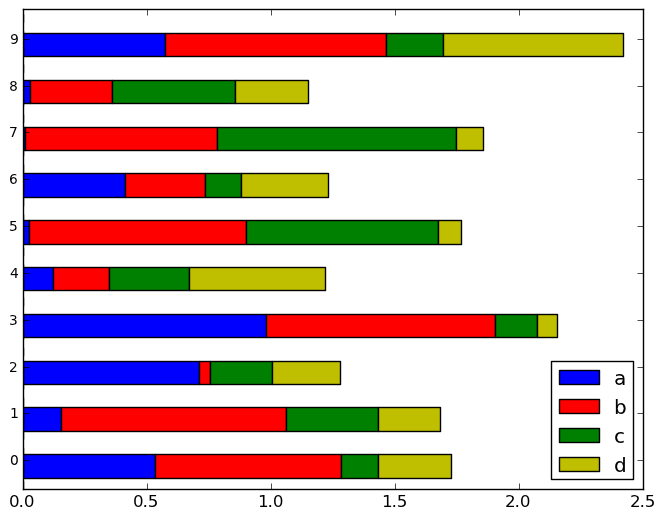
- Add log x and y scaling options to DataFrame.plot and Series.plot
- Add kurt methods to Series and DataFrame for computing kurtosis
NA Boolean Comparison API Change¶
Reverted some changes to how NA values (represented typically as NaN or None) are handled in non-numeric Series:
In [1264]: series = Series(['Steve', np.nan, 'Joe'])
In [1265]: series == 'Steve'
Out[1265]:
0 True
1 False
2 False
In [1266]: series != 'Steve'
Out[1266]:
0 False
1 True
2 True
In comparisons, NA / NaN will always come through as False except with != which is True. Be very careful with boolean arithmetic, especially negation, in the presence of NA data. You may wish to add an explicit NA filter into boolean array operations if you are worried about this:
In [1267]: mask = series == 'Steve'
In [1268]: series[mask & series.notnull()]
Out[1268]: 0 Steve
While propagating NA in comparisons may seem like the right behavior to some users (and you could argue on purely technical grounds that this is the right thing to do), the evaluation was made that propagating NA everywhere, including in numerical arrays, would cause a large amount of problems for users. Thus, a “practicality beats purity” approach was taken. This issue may be revisited at some point in the future.
Other API Changes¶
When calling apply on a grouped Series, the return value will also be a Series, to be more consistent with the groupby behavior with DataFrame:
In [1269]: df = DataFrame({'A' : ['foo', 'bar', 'foo', 'bar',
......: 'foo', 'bar', 'foo', 'foo'],
......: 'B' : ['one', 'one', 'two', 'three',
......: 'two', 'two', 'one', 'three'],
......: 'C' : np.random.randn(8), 'D' : np.random.randn(8)})
......:
In [1270]: df
Out[1270]:
A B C D
0 foo one 0.082144 -0.498406
1 bar one 0.140209 1.454595
2 foo two 0.751957 1.249438
3 bar three 0.069992 1.083574
4 foo two 0.944691 0.890245
5 bar two -1.004030 0.530267
6 foo one 1.573567 -0.033486
7 foo three 0.160244 -0.731919
In [1271]: grouped = df.groupby('A')['C']
In [1272]: grouped.describe()
Out[1272]:
A
bar count 3.000000
mean -0.264610
std 0.641318
min -1.004030
25% -0.467019
50% 0.069992
75% 0.105100
max 0.140209
foo count 5.000000
mean 0.702520
std 0.612116
min 0.082144
25% 0.160244
50% 0.751957
75% 0.944691
max 1.573567
In [1273]: grouped.apply(lambda x: x.order()[-2:]) # top 2 values
Out[1273]:
A
bar 3 0.069992
1 0.140209
foo 4 0.944691
6 1.573567
v.0.7.2 (March 16, 2012)¶
This release targets bugs in 0.7.1, and adds a few minor features.
New features¶
- Add additional tie-breaking methods in DataFrame.rank (GH874)
- Add ascending parameter to rank in Series, DataFrame (GH875)
- Add coerce_float option to DataFrame.from_records (GH893)
- Add sort_columns parameter to allow unsorted plots (GH918)
- Enable column access via attributes on GroupBy (GH882)
- Can pass dict of values to DataFrame.fillna (GH661)
- Can select multiple hierarchical groups by passing list of values in .ix (GH134)
- Add axis option to DataFrame.fillna (GH174)
- Add level keyword to drop for dropping values from a level (GH159)
v.0.7.1 (February 29, 2012)¶
This release includes a few new features and addresses over a dozen bugs in 0.7.0.
New features¶
- Add to_clipboard function to pandas namespace for writing objects to the system clipboard (GH774)
- Add itertuples method to DataFrame for iterating through the rows of a dataframe as tuples (GH818)
- Add ability to pass fill_value and method to DataFrame and Series align method (GH806, GH807)
- Add fill_value option to reindex, align methods (GH784)
- Enable concat to produce DataFrame from Series (GH787)
- Add between method to Series (GH802)
- Add HTML representation hook to DataFrame for the IPython HTML notebook (GH773)
- Support for reading Excel 2007 XML documents using openpyxl
v.0.7.0 (February 9, 2012)¶
New features¶
- New unified merge function for efficiently performing full gamut of database / relational-algebra operations. Refactored existing join methods to use the new infrastructure, resulting in substantial performance gains (GH220, GH249, GH267)
- New unified concatenation function for concatenating Series, DataFrame or Panel objects along an axis. Can form union or intersection of the other axes. Improves performance of Series.append and DataFrame.append (GH468, GH479, GH273)
- Can pass multiple DataFrames to DataFrame.append to concatenate (stack) and multiple Series to Series.append too
- Can pass list of dicts (e.g., a list of JSON objects) to DataFrame constructor (GH526)
- You can now set multiple columns in a DataFrame via __getitem__, useful for transformation (GH342)
- Handle differently-indexed output values in DataFrame.apply (GH498)
In [1274]: df = DataFrame(randn(10, 4))
In [1275]: df.apply(lambda x: x.describe())
Out[1275]:
0 1 2 3
count 10.000000 10.000000 10.000000 10.000000
mean 0.306312 0.269999 -0.067545 0.040813
std 0.700177 0.967069 1.065426 1.021549
min -1.053371 -1.066290 -2.007297 -0.945913
25% -0.106059 -0.461998 -0.511928 -0.691136
50% 0.361972 0.550510 0.022757 -0.487368
75% 0.750146 0.767581 0.250025 0.621327
max 1.451428 1.605616 1.722068 2.008174
- Add reorder_levels method to Series and DataFrame (PR534)
- Add dict-like get function to DataFrame and Panel (PR521)
- Add DataFrame.iterrows method for efficiently iterating through the rows of a DataFrame
- Add DataFrame.to_panel with code adapted from LongPanel.to_long
- Add reindex_axis method added to DataFrame
- Add level option to binary arithmetic functions on DataFrame and Series
- Add level option to the reindex and align methods on Series and DataFrame for broadcasting values across a level (GH542, PR552, others)
- Add attribute-based item access to Panel and add IPython completion (PR563)
- Add logy option to Series.plot for log-scaling on the Y axis
- Add index and header options to DataFrame.to_string
- Can pass multiple DataFrames to DataFrame.join to join on index (GH115)
- Can pass multiple Panels to Panel.join (GH115)
- Added justify argument to DataFrame.to_string to allow different alignment of column headers
- Add sort option to GroupBy to allow disabling sorting of the group keys for potential speedups (GH595)
- Can pass MaskedArray to Series constructor (PR563)
- Add Panel item access via attributes and IPython completion (GH554)
- Implement DataFrame.lookup, fancy-indexing analogue for retrieving values given a sequence of row and column labels (GH338)
- Can pass a list of functions to aggregate with groupby on a DataFrame, yielding an aggregated result with hierarchical columns (GH166)
- Can call cummin and cummax on Series and DataFrame to get cumulative minimum and maximum, respectively (GH647)
- value_range added as utility function to get min and max of a dataframe (GH288)
- Added encoding argument to read_csv, read_table, to_csv and from_csv for non-ascii text (GH717)
- Added abs method to pandas objects
- Added crosstab function for easily computing frequency tables
- Added isin method to index objects
- Added level argument to xs method of DataFrame.
API Changes to integer indexing¶
One of the potentially riskiest API changes in 0.7.0, but also one of the most important, was a complete review of how integer indexes are handled with regard to label-based indexing. Here is an example:
In [1276]: s = Series(randn(10), index=range(0, 20, 2))
In [1277]: s
Out[1277]:
0 1.325029
2 0.914218
4 0.026404
6 1.020839
8 -0.992183
10 1.277345
12 -0.984925
14 0.376436
16 -0.545691
18 -1.961682
In [1278]: s[0]
Out[1278]: 1.3250288185283847
In [1279]: s[2]
Out[1279]: 0.91421801177592665
In [1280]: s[4]
Out[1280]: 0.026404326158588228
This is all exactly identical to the behavior before. However, if you ask for a key not contained in the Series, in versions 0.6.1 and prior, Series would fall back on a location-based lookup. This now raises a KeyError:
In [2]: s[1]
KeyError: 1
This change also has the same impact on DataFrame:
In [3]: df = DataFrame(randn(8, 4), index=range(0, 16, 2))
In [4]: df
0 1 2 3
0 0.88427 0.3363 -0.1787 0.03162
2 0.14451 -0.1415 0.2504 0.58374
4 -1.44779 -0.9186 -1.4996 0.27163
6 -0.26598 -2.4184 -0.2658 0.11503
8 -0.58776 0.3144 -0.8566 0.61941
10 0.10940 -0.7175 -1.0108 0.47990
12 -1.16919 -0.3087 -0.6049 -0.43544
14 -0.07337 0.3410 0.0424 -0.16037
In [5]: df.ix[3]
KeyError: 3
In order to support purely integer-based indexing, the following methods have been added:
Method | Description |
---|---|
Series.iget_value(i) | Retrieve value stored at location i |
Series.iget(i) | Alias for iget_value |
DataFrame.irow(i) | Retrieve the i-th row |
DataFrame.icol(j) | Retrieve the j-th column |
DataFrame.iget_value(i, j) | Retrieve the value at row i and column j |
API tweaks regarding label-based slicing¶
Label-based slicing using ix now requires that the index be sorted (monotonic) unless both the start and endpoint are contained in the index:
In [1281]: s = Series(randn(6), index=list('gmkaec'))
In [1282]: s
Out[1282]:
g 1.062704
m -1.479631
k -0.569105
a 2.011439
e 0.451906
c -0.142689
Then this is OK:
In [1283]: s.ix['k':'e']
Out[1283]:
k -0.569105
a 2.011439
e 0.451906
But this is not:
In [12]: s.ix['b':'h']
KeyError 'b'
If the index had been sorted, the “range selection” would have been possible:
In [1284]: s2 = s.sort_index()
In [1285]: s2
Out[1285]:
a 2.011439
c -0.142689
e 0.451906
g 1.062704
k -0.569105
m -1.479631
In [1286]: s2.ix['b':'h']
Out[1286]:
c -0.142689
e 0.451906
g 1.062704
Changes to Series [] operator¶
As as notational convenience, you can pass a sequence of labels or a label slice to a Series when getting and setting values via [] (i.e. the __getitem__ and __setitem__ methods). The behavior will be the same as passing similar input to ix except in the case of integer indexing:
In [1287]: s = Series(randn(6), index=list('acegkm'))
In [1288]: s
Out[1288]:
a 0.207864
c 2.287787
e -0.386070
g -0.343892
k 0.845395
m 0.364465
In [1289]: s[['m', 'a', 'c', 'e']]
Out[1289]:
m 0.364465
a 0.207864
c 2.287787
e -0.386070
In [1290]: s['b':'l']
Out[1290]:
c 2.287787
e -0.386070
g -0.343892
k 0.845395
In [1291]: s['c':'k']
Out[1291]:
c 2.287787
e -0.386070
g -0.343892
k 0.845395
In the case of integer indexes, the behavior will be exactly as before (shadowing ndarray):
In [1292]: s = Series(randn(6), index=range(0, 12, 2))
In [1293]: s[[4, 0, 2]]
Out[1293]:
4 0.336739
0 -0.205923
2 -1.310550
In [1294]: s[1:5]
Out[1294]:
2 -1.310550
4 0.336739
6 0.440363
8 0.271244
If you wish to do indexing with sequences and slicing on an integer index with label semantics, use ix.
Other API Changes¶
- The deprecated LongPanel class has been completely removed
- If Series.sort is called on a column of a DataFrame, an exception will now be raised. Before it was possible to accidentally mutate a DataFrame’s column by doing df[col].sort() instead of the side-effect free method df[col].order() (GH316)
- Miscellaneous renames and deprecations which will (harmlessly) raise FutureWarning
- drop added as an optional parameter to DataFrame.reset_index (GH699)
Performance improvements¶
- Cythonized GroupBy aggregations no longer presort the data, thus achieving a significant speedup (GH93). GroupBy aggregations with Python functions significantly sped up by clever manipulation of the ndarray data type in Cython (GH496).
- Better error message in DataFrame constructor when passed column labels don’t match data (GH497)
- Substantially improve performance of multi-GroupBy aggregation when a Python function is passed, reuse ndarray object in Cython (GH496)
- Can store objects indexed by tuples and floats in HDFStore (GH492)
- Don’t print length by default in Series.to_string, add length option (GH489)
- Improve Cython code for multi-groupby to aggregate without having to sort the data (GH93)
- Improve MultiIndex reindexing speed by storing tuples in the MultiIndex, test for backwards unpickling compatibility
- Improve column reindexing performance by using specialized Cython take function
- Further performance tweaking of Series.__getitem__ for standard use cases
- Avoid Index dict creation in some cases (i.e. when getting slices, etc.), regression from prior versions
- Friendlier error message in setup.py if NumPy not installed
- Use common set of NA-handling operations (sum, mean, etc.) in Panel class also (GH536)
- Default name assignment when calling reset_index on DataFrame with a regular (non-hierarchical) index (GH476)
- Use Cythonized groupers when possible in Series/DataFrame stat ops with level parameter passed (GH545)
- Ported skiplist data structure to C to speed up rolling_median by about 5-10x in most typical use cases (GH374)
v.0.6.1 (December 13, 2011)¶
New features¶
- Can append single rows (as Series) to a DataFrame
- Add Spearman and Kendall rank correlation options to Series.corr and DataFrame.corr (GH428)
- Added get_value and set_value methods to Series, DataFrame, and Panel for very low-overhead access (>2x faster in many cases) to scalar elements (GH437, GH438). set_value is capable of producing an enlarged object.
- Add PyQt table widget to sandbox (PR435)
- DataFrame.align can accept Series arguments and an axis option (GH461)
- Implement new SparseArray and SparseList data structures. SparseSeries now derives from SparseArray (GH463)
- Better console printing options (PR453)
- Implement fast data ranking for Series and DataFrame, fast versions of scipy.stats.rankdata (GH428)
- Implement DataFrame.from_items alternate constructor (GH444)
- DataFrame.convert_objects method for inferring better dtypes for object columns (GH302)
- Add rolling_corr_pairwise function for computing Panel of correlation matrices (GH189)
- Add margins option to pivot_table for computing subgroup aggregates (GH114)
- Add Series.from_csv function (PR482)
- Can pass DataFrame/DataFrame and DataFrame/Series to rolling_corr/rolling_cov (GH #462)
- MultiIndex.get_level_values can accept the level name
Performance improvements¶
- Improve memory usage of DataFrame.describe (do not copy data unnecessarily) (PR #425)
- Optimize scalar value lookups in the general case by 25% or more in Series and DataFrame
- Fix performance regression in cross-sectional count in DataFrame, affecting DataFrame.dropna speed
- Column deletion in DataFrame copies no data (computes views on blocks) (GH #158)
v.0.6.0 (November 25, 2011)¶
New Features¶
- Added melt function to pandas.core.reshape
- Added level parameter to group by level in Series and DataFrame descriptive statistics (PR313)
- Added head and tail methods to Series, analogous to to DataFrame (PR296)
- Added Series.isin function which checks if each value is contained in a passed sequence (GH289)
- Added float_format option to Series.to_string
- Added skip_footer (GH291) and converters (GH343) options to read_csv and read_table
- Added drop_duplicates and duplicated functions for removing duplicate DataFrame rows and checking for duplicate rows, respectively (GH319)
- Implemented operators ‘&’, ‘|’, ‘^’, ‘-‘ on DataFrame (GH347)
- Added Series.mad, mean absolute deviation
- Added QuarterEnd DateOffset (PR321)
- Added dot to DataFrame (GH65)
- Added orient option to Panel.from_dict (GH359, GH301)
- Added orient option to DataFrame.from_dict
- Added passing list of tuples or list of lists to DataFrame.from_records (GH357)
- Added multiple levels to groupby (GH103)
- Allow multiple columns in by argument of DataFrame.sort_index (GH92, PR362)
- Added fast get_value and put_value methods to DataFrame (GH360)
- Added cov instance methods to Series and DataFrame (GH194, PR362)
- Added kind='bar' option to DataFrame.plot (PR348)
- Added idxmin and idxmax to Series and DataFrame (PR286)
- Added read_clipboard function to parse DataFrame from clipboard (GH300)
- Added nunique function to Series for counting unique elements (GH297)
- Made DataFrame constructor use Series name if no columns passed (GH373)
- Support regular expressions in read_table/read_csv (GH364)
- Added DataFrame.to_html for writing DataFrame to HTML (PR387)
- Added support for MaskedArray data in DataFrame, masked values converted to NaN (PR396)
- Added DataFrame.boxplot function (GH368)
- Can pass extra args, kwds to DataFrame.apply (GH376)
- Implement DataFrame.join with vector on argument (GH312)
- Added legend boolean flag to DataFrame.plot (GH324)
- Can pass multiple levels to stack and unstack (GH370)
- Can pass multiple values columns to pivot_table (GH381)
- Use Series name in GroupBy for result index (GH363)
- Added raw option to DataFrame.apply for performance if only need ndarray (GH309)
- Added proper, tested weighted least squares to standard and panel OLS (GH303)
Performance Enhancements¶
- VBENCH Cythonized cache_readonly, resulting in substantial micro-performance enhancements throughout the codebase (GH361)
- VBENCH Special Cython matrix iterator for applying arbitrary reduction operations with 3-5x better performance than np.apply_along_axis (GH309)
- VBENCH Improved performance of MultiIndex.from_tuples
- VBENCH Special Cython matrix iterator for applying arbitrary reduction operations
- VBENCH + DOCUMENT Add raw option to DataFrame.apply for getting better performance when
- VBENCH Faster cythonized count by level in Series and DataFrame (GH341)
- VBENCH? Significant GroupBy performance enhancement with multiple keys with many “empty” combinations
- VBENCH New Cython vectorized function map_infer speeds up Series.apply and Series.map significantly when passed elementwise Python function, motivated by (PR355)
- VBENCH Significantly improved performance of Series.order, which also makes np.unique called on a Series faster (GH327)
- VBENCH Vastly improved performance of GroupBy on axes with a MultiIndex (GH299)
v.0.5.0 (October 24, 2011)¶
New Features¶
- Added DataFrame.align method with standard join options
- Added parse_dates option to read_csv and read_table methods to optionally try to parse dates in the index columns
- Added nrows, chunksize, and iterator arguments to read_csv and read_table. The last two return a new TextParser class capable of lazily iterating through chunks of a flat file (GH242)
- Added ability to join on multiple columns in DataFrame.join (GH214)
- Added private _get_duplicates function to Index for identifying duplicate values more easily (ENH5c)
- Added column attribute access to DataFrame.
- Added Python tab completion hook for DataFrame columns. (PR233, GH230)
- Implemented Series.describe for Series containing objects (PR241)
- Added inner join option to DataFrame.join when joining on key(s) (GH248)
- Implemented selecting DataFrame columns by passing a list to __getitem__ (GH253)
- Implemented & and | to intersect / union Index objects, respectively (GH261)
- Added pivot_table convenience function to pandas namespace (GH234)
- Implemented Panel.rename_axis function (GH243)
- DataFrame will show index level names in console output (PR334)
- Implemented Panel.take
- Added set_eng_float_format for alternate DataFrame floating point string formatting (ENH61)
- Added convenience set_index function for creating a DataFrame index from its existing columns
- Implemented groupby hierarchical index level name (GH223)
- Added support for different delimiters in DataFrame.to_csv (PR244)
- TODO: DOCS ABOUT TAKE METHODS
Performance Enhancements¶
- VBENCH Major performance improvements in file parsing functions read_csv and read_table
- VBENCH Added Cython function for converting tuples to ndarray very fast. Speeds up many MultiIndex-related operations
- VBENCH Refactored merging / joining code into a tidy class and disabled unnecessary computations in the float/object case, thus getting about 10% better performance (GH211)
- VBENCH Improved speed of DataFrame.xs on mixed-type DataFrame objects by about 5x, regression from 0.3.0 (GH215)
- VBENCH With new DataFrame.align method, speeding up binary operations between differently-indexed DataFrame objects by 10-25%.
- VBENCH Significantly sped up conversion of nested dict into DataFrame (GH212)
- VBENCH Significantly speed up DataFrame __repr__ and count on large mixed-type DataFrame objects
v.0.4.3 through v0.4.1 (September 25 - October 9, 2011)¶
New Features¶
- Added Python 3 support using 2to3 (PR200)
- Added name attribute to Series, now prints as part of Series.__repr__
- Added instance methods isnull and notnull to Series (PR209, GH203)
- Added Series.align method for aligning two series with choice of join method (ENH56)
- Added method get_level_values to MultiIndex (IS188)
- Set values in mixed-type DataFrame objects via .ix indexing attribute (GH135)
- Added new DataFrame methods get_dtype_counts and property dtypes (ENHdc)
- Added ignore_index option to DataFrame.append to stack DataFrames (ENH1b)
- read_csv tries to sniff delimiters using csv.Sniffer (PR146)
- read_csv can read multiple columns into a MultiIndex; DataFrame’s to_csv method writes out a corresponding MultiIndex (PR151)
- DataFrame.rename has a new copy parameter to rename a DataFrame in place (ENHed)
- Enable unstacking by name (PR142)
- Enable sortlevel to work by level (PR141)
Performance Enhancements¶
- Altered binary operations on differently-indexed SparseSeries objects to use the integer-based (dense) alignment logic which is faster with a larger number of blocks (GH205)
- Wrote faster Cython data alignment / merging routines resulting in substantial speed increases
- Improved performance of isnull and notnull, a regression from v0.3.0 (GH187)
- Refactored code related to DataFrame.join so that intermediate aligned copies of the data in each DataFrame argument do not need to be created. Substantial performance increases result (GH176)
- Substantially improved performance of generic Index.intersection and Index.union
- Implemented BlockManager.take resulting in significantly faster take performance on mixed-type DataFrame objects (GH104)
- Improved performance of Series.sort_index
- Significant groupby performance enhancement: removed unnecessary integrity checks in DataFrame internals that were slowing down slicing operations to retrieve groups
- Optimized _ensure_index function resulting in performance savings in type-checking Index objects
- Wrote fast time series merging / joining methods in Cython. Will be integrated later into DataFrame.join and related functions