10 minutes to pandas#
This is a short introduction to pandas, geared mainly for new users. You can see more complex recipes in the Cookbook.
Customarily, we import as follows:
In [1]: import numpy as np
In [2]: import pandas as pd
Object creation#
See the Intro to data structures section.
Creating a Series
by passing a list of values, letting pandas create
a default integer index:
In [3]: s = pd.Series([1, 3, 5, np.nan, 6, 8])
In [4]: s
Out[4]:
0 1.0
1 3.0
2 5.0
3 NaN
4 6.0
5 8.0
dtype: float64
Creating a DataFrame
by passing a NumPy array, with a datetime index using date_range()
and labeled columns:
In [5]: dates = pd.date_range("20130101", periods=6)
In [6]: dates
Out[6]:
DatetimeIndex(['2013-01-01', '2013-01-02', '2013-01-03', '2013-01-04',
'2013-01-05', '2013-01-06'],
dtype='datetime64[ns]', freq='D')
In [7]: df = pd.DataFrame(np.random.randn(6, 4), index=dates, columns=list("ABCD"))
In [8]: df
Out[8]:
A B C D
2013-01-01 0.469112 -0.282863 -1.509059 -1.135632
2013-01-02 1.212112 -0.173215 0.119209 -1.044236
2013-01-03 -0.861849 -2.104569 -0.494929 1.071804
2013-01-04 0.721555 -0.706771 -1.039575 0.271860
2013-01-05 -0.424972 0.567020 0.276232 -1.087401
2013-01-06 -0.673690 0.113648 -1.478427 0.524988
Creating a DataFrame
by passing a dictionary of objects that can be
converted into a series-like structure:
In [9]: df2 = pd.DataFrame(
...: {
...: "A": 1.0,
...: "B": pd.Timestamp("20130102"),
...: "C": pd.Series(1, index=list(range(4)), dtype="float32"),
...: "D": np.array([3] * 4, dtype="int32"),
...: "E": pd.Categorical(["test", "train", "test", "train"]),
...: "F": "foo",
...: }
...: )
...:
In [10]: df2
Out[10]:
A B C D E F
0 1.0 2013-01-02 1.0 3 test foo
1 1.0 2013-01-02 1.0 3 train foo
2 1.0 2013-01-02 1.0 3 test foo
3 1.0 2013-01-02 1.0 3 train foo
The columns of the resulting DataFrame
have different
dtypes:
In [11]: df2.dtypes
Out[11]:
A float64
B datetime64[ns]
C float32
D int32
E category
F object
dtype: object
If you’re using IPython, tab completion for column names (as well as public attributes) is automatically enabled. Here’s a subset of the attributes that will be completed:
In [12]: df2.<TAB> # noqa: E225, E999
df2.A df2.bool
df2.abs df2.boxplot
df2.add df2.C
df2.add_prefix df2.clip
df2.add_suffix df2.columns
df2.align df2.copy
df2.all df2.count
df2.any df2.combine
df2.append df2.D
df2.apply df2.describe
df2.applymap df2.diff
df2.B df2.duplicated
As you can see, the columns A
, B
, C
, and D
are automatically
tab completed. E
and F
are there as well; the rest of the attributes have been
truncated for brevity.
Viewing data#
See the Basics section.
Use DataFrame.head()
and DataFrame.tail()
to view the top and bottom rows of the frame
respectively:
In [13]: df.head()
Out[13]:
A B C D
2013-01-01 0.469112 -0.282863 -1.509059 -1.135632
2013-01-02 1.212112 -0.173215 0.119209 -1.044236
2013-01-03 -0.861849 -2.104569 -0.494929 1.071804
2013-01-04 0.721555 -0.706771 -1.039575 0.271860
2013-01-05 -0.424972 0.567020 0.276232 -1.087401
In [14]: df.tail(3)
Out[14]:
A B C D
2013-01-04 0.721555 -0.706771 -1.039575 0.271860
2013-01-05 -0.424972 0.567020 0.276232 -1.087401
2013-01-06 -0.673690 0.113648 -1.478427 0.524988
Display the DataFrame.index
or DataFrame.columns
:
In [15]: df.index
Out[15]:
DatetimeIndex(['2013-01-01', '2013-01-02', '2013-01-03', '2013-01-04',
'2013-01-05', '2013-01-06'],
dtype='datetime64[ns]', freq='D')
In [16]: df.columns
Out[16]: Index(['A', 'B', 'C', 'D'], dtype='object')
DataFrame.to_numpy()
gives a NumPy representation of the underlying data.
Note that this can be an expensive operation when your DataFrame
has
columns with different data types, which comes down to a fundamental difference
between pandas and NumPy: NumPy arrays have one dtype for the entire array,
while pandas DataFrames have one dtype per column. When you call
DataFrame.to_numpy()
, pandas will find the NumPy dtype that can hold all
of the dtypes in the DataFrame. This may end up being object
, which requires
casting every value to a Python object.
For df
, our DataFrame
of all floating-point values, and
DataFrame.to_numpy()
is fast and doesn’t require copying data:
In [17]: df.to_numpy()
Out[17]:
array([[ 0.4691, -0.2829, -1.5091, -1.1356],
[ 1.2121, -0.1732, 0.1192, -1.0442],
[-0.8618, -2.1046, -0.4949, 1.0718],
[ 0.7216, -0.7068, -1.0396, 0.2719],
[-0.425 , 0.567 , 0.2762, -1.0874],
[-0.6737, 0.1136, -1.4784, 0.525 ]])
For df2
, the DataFrame
with multiple dtypes,
DataFrame.to_numpy()
is relatively expensive:
In [18]: df2.to_numpy()
Out[18]:
array([[1.0, Timestamp('2013-01-02 00:00:00'), 1.0, 3, 'test', 'foo'],
[1.0, Timestamp('2013-01-02 00:00:00'), 1.0, 3, 'train', 'foo'],
[1.0, Timestamp('2013-01-02 00:00:00'), 1.0, 3, 'test', 'foo'],
[1.0, Timestamp('2013-01-02 00:00:00'), 1.0, 3, 'train', 'foo']],
dtype=object)
Note
DataFrame.to_numpy()
does not include the index or column
labels in the output.
describe()
shows a quick statistic summary of your data:
In [19]: df.describe()
Out[19]:
A B C D
count 6.000000 6.000000 6.000000 6.000000
mean 0.073711 -0.431125 -0.687758 -0.233103
std 0.843157 0.922818 0.779887 0.973118
min -0.861849 -2.104569 -1.509059 -1.135632
25% -0.611510 -0.600794 -1.368714 -1.076610
50% 0.022070 -0.228039 -0.767252 -0.386188
75% 0.658444 0.041933 -0.034326 0.461706
max 1.212112 0.567020 0.276232 1.071804
Transposing your data:
In [20]: df.T
Out[20]:
2013-01-01 2013-01-02 2013-01-03 2013-01-04 2013-01-05 2013-01-06
A 0.469112 1.212112 -0.861849 0.721555 -0.424972 -0.673690
B -0.282863 -0.173215 -2.104569 -0.706771 0.567020 0.113648
C -1.509059 0.119209 -0.494929 -1.039575 0.276232 -1.478427
D -1.135632 -1.044236 1.071804 0.271860 -1.087401 0.524988
DataFrame.sort_index()
sorts by an axis:
In [21]: df.sort_index(axis=1, ascending=False)
Out[21]:
D C B A
2013-01-01 -1.135632 -1.509059 -0.282863 0.469112
2013-01-02 -1.044236 0.119209 -0.173215 1.212112
2013-01-03 1.071804 -0.494929 -2.104569 -0.861849
2013-01-04 0.271860 -1.039575 -0.706771 0.721555
2013-01-05 -1.087401 0.276232 0.567020 -0.424972
2013-01-06 0.524988 -1.478427 0.113648 -0.673690
DataFrame.sort_values()
sorts by values:
In [22]: df.sort_values(by="B")
Out[22]:
A B C D
2013-01-03 -0.861849 -2.104569 -0.494929 1.071804
2013-01-04 0.721555 -0.706771 -1.039575 0.271860
2013-01-01 0.469112 -0.282863 -1.509059 -1.135632
2013-01-02 1.212112 -0.173215 0.119209 -1.044236
2013-01-06 -0.673690 0.113648 -1.478427 0.524988
2013-01-05 -0.424972 0.567020 0.276232 -1.087401
Selection#
Note
While standard Python / NumPy expressions for selecting and setting are
intuitive and come in handy for interactive work, for production code, we
recommend the optimized pandas data access methods, DataFrame.at()
, DataFrame.iat()
,
DataFrame.loc()
and DataFrame.iloc()
.
See the indexing documentation Indexing and Selecting Data and MultiIndex / Advanced Indexing.
Getting#
Selecting a single column, which yields a Series
,
equivalent to df.A
:
In [23]: df["A"]
Out[23]:
2013-01-01 0.469112
2013-01-02 1.212112
2013-01-03 -0.861849
2013-01-04 0.721555
2013-01-05 -0.424972
2013-01-06 -0.673690
Freq: D, Name: A, dtype: float64
Selecting via []
(__getitem__
), which slices the rows:
In [24]: df[0:3]
Out[24]:
A B C D
2013-01-01 0.469112 -0.282863 -1.509059 -1.135632
2013-01-02 1.212112 -0.173215 0.119209 -1.044236
2013-01-03 -0.861849 -2.104569 -0.494929 1.071804
In [25]: df["20130102":"20130104"]
Out[25]:
A B C D
2013-01-02 1.212112 -0.173215 0.119209 -1.044236
2013-01-03 -0.861849 -2.104569 -0.494929 1.071804
2013-01-04 0.721555 -0.706771 -1.039575 0.271860
Selection by label#
See more in Selection by Label using DataFrame.loc()
or DataFrame.at()
.
For getting a cross section using a label:
In [26]: df.loc[dates[0]]
Out[26]:
A 0.469112
B -0.282863
C -1.509059
D -1.135632
Name: 2013-01-01 00:00:00, dtype: float64
Selecting on a multi-axis by label:
In [27]: df.loc[:, ["A", "B"]]
Out[27]:
A B
2013-01-01 0.469112 -0.282863
2013-01-02 1.212112 -0.173215
2013-01-03 -0.861849 -2.104569
2013-01-04 0.721555 -0.706771
2013-01-05 -0.424972 0.567020
2013-01-06 -0.673690 0.113648
Showing label slicing, both endpoints are included:
In [28]: df.loc["20130102":"20130104", ["A", "B"]]
Out[28]:
A B
2013-01-02 1.212112 -0.173215
2013-01-03 -0.861849 -2.104569
2013-01-04 0.721555 -0.706771
Reduction in the dimensions of the returned object:
In [29]: df.loc["20130102", ["A", "B"]]
Out[29]:
A 1.212112
B -0.173215
Name: 2013-01-02 00:00:00, dtype: float64
For getting a scalar value:
In [30]: df.loc[dates[0], "A"]
Out[30]: 0.4691122999071863
For getting fast access to a scalar (equivalent to the prior method):
In [31]: df.at[dates[0], "A"]
Out[31]: 0.4691122999071863
Selection by position#
See more in Selection by Position using DataFrame.iloc()
or DataFrame.at()
.
Select via the position of the passed integers:
In [32]: df.iloc[3]
Out[32]:
A 0.721555
B -0.706771
C -1.039575
D 0.271860
Name: 2013-01-04 00:00:00, dtype: float64
By integer slices, acting similar to NumPy/Python:
In [33]: df.iloc[3:5, 0:2]
Out[33]:
A B
2013-01-04 0.721555 -0.706771
2013-01-05 -0.424972 0.567020
By lists of integer position locations, similar to the NumPy/Python style:
In [34]: df.iloc[[1, 2, 4], [0, 2]]
Out[34]:
A C
2013-01-02 1.212112 0.119209
2013-01-03 -0.861849 -0.494929
2013-01-05 -0.424972 0.276232
For slicing rows explicitly:
In [35]: df.iloc[1:3, :]
Out[35]:
A B C D
2013-01-02 1.212112 -0.173215 0.119209 -1.044236
2013-01-03 -0.861849 -2.104569 -0.494929 1.071804
For slicing columns explicitly:
In [36]: df.iloc[:, 1:3]
Out[36]:
B C
2013-01-01 -0.282863 -1.509059
2013-01-02 -0.173215 0.119209
2013-01-03 -2.104569 -0.494929
2013-01-04 -0.706771 -1.039575
2013-01-05 0.567020 0.276232
2013-01-06 0.113648 -1.478427
For getting a value explicitly:
In [37]: df.iloc[1, 1]
Out[37]: -0.17321464905330858
For getting fast access to a scalar (equivalent to the prior method):
In [38]: df.iat[1, 1]
Out[38]: -0.17321464905330858
Boolean indexing#
Using a single column’s values to select data:
In [39]: df[df["A"] > 0]
Out[39]:
A B C D
2013-01-01 0.469112 -0.282863 -1.509059 -1.135632
2013-01-02 1.212112 -0.173215 0.119209 -1.044236
2013-01-04 0.721555 -0.706771 -1.039575 0.271860
Selecting values from a DataFrame where a boolean condition is met:
In [40]: df[df > 0]
Out[40]:
A B C D
2013-01-01 0.469112 NaN NaN NaN
2013-01-02 1.212112 NaN 0.119209 NaN
2013-01-03 NaN NaN NaN 1.071804
2013-01-04 0.721555 NaN NaN 0.271860
2013-01-05 NaN 0.567020 0.276232 NaN
2013-01-06 NaN 0.113648 NaN 0.524988
Using the isin()
method for filtering:
In [41]: df2 = df.copy()
In [42]: df2["E"] = ["one", "one", "two", "three", "four", "three"]
In [43]: df2
Out[43]:
A B C D E
2013-01-01 0.469112 -0.282863 -1.509059 -1.135632 one
2013-01-02 1.212112 -0.173215 0.119209 -1.044236 one
2013-01-03 -0.861849 -2.104569 -0.494929 1.071804 two
2013-01-04 0.721555 -0.706771 -1.039575 0.271860 three
2013-01-05 -0.424972 0.567020 0.276232 -1.087401 four
2013-01-06 -0.673690 0.113648 -1.478427 0.524988 three
In [44]: df2[df2["E"].isin(["two", "four"])]
Out[44]:
A B C D E
2013-01-03 -0.861849 -2.104569 -0.494929 1.071804 two
2013-01-05 -0.424972 0.567020 0.276232 -1.087401 four
Setting#
Setting a new column automatically aligns the data by the indexes:
In [45]: s1 = pd.Series([1, 2, 3, 4, 5, 6], index=pd.date_range("20130102", periods=6))
In [46]: s1
Out[46]:
2013-01-02 1
2013-01-03 2
2013-01-04 3
2013-01-05 4
2013-01-06 5
2013-01-07 6
Freq: D, dtype: int64
In [47]: df["F"] = s1
Setting values by label:
In [48]: df.at[dates[0], "A"] = 0
Setting values by position:
In [49]: df.iat[0, 1] = 0
Setting by assigning with a NumPy array:
In [50]: df.loc[:, "D"] = np.array([5] * len(df))
The result of the prior setting operations:
In [51]: df
Out[51]:
A B C D F
2013-01-01 0.000000 0.000000 -1.509059 5 NaN
2013-01-02 1.212112 -0.173215 0.119209 5 1.0
2013-01-03 -0.861849 -2.104569 -0.494929 5 2.0
2013-01-04 0.721555 -0.706771 -1.039575 5 3.0
2013-01-05 -0.424972 0.567020 0.276232 5 4.0
2013-01-06 -0.673690 0.113648 -1.478427 5 5.0
A where
operation with setting:
In [52]: df2 = df.copy()
In [53]: df2[df2 > 0] = -df2
In [54]: df2
Out[54]:
A B C D F
2013-01-01 0.000000 0.000000 -1.509059 -5 NaN
2013-01-02 -1.212112 -0.173215 -0.119209 -5 -1.0
2013-01-03 -0.861849 -2.104569 -0.494929 -5 -2.0
2013-01-04 -0.721555 -0.706771 -1.039575 -5 -3.0
2013-01-05 -0.424972 -0.567020 -0.276232 -5 -4.0
2013-01-06 -0.673690 -0.113648 -1.478427 -5 -5.0
Missing data#
pandas primarily uses the value np.nan
to represent missing data. It is by
default not included in computations. See the Missing Data section.
Reindexing allows you to change/add/delete the index on a specified axis. This returns a copy of the data:
In [55]: df1 = df.reindex(index=dates[0:4], columns=list(df.columns) + ["E"])
In [56]: df1.loc[dates[0] : dates[1], "E"] = 1
In [57]: df1
Out[57]:
A B C D F E
2013-01-01 0.000000 0.000000 -1.509059 5 NaN 1.0
2013-01-02 1.212112 -0.173215 0.119209 5 1.0 1.0
2013-01-03 -0.861849 -2.104569 -0.494929 5 2.0 NaN
2013-01-04 0.721555 -0.706771 -1.039575 5 3.0 NaN
DataFrame.dropna()
drops any rows that have missing data:
In [58]: df1.dropna(how="any")
Out[58]:
A B C D F E
2013-01-02 1.212112 -0.173215 0.119209 5 1.0 1.0
DataFrame.fillna()
fills missing data:
In [59]: df1.fillna(value=5)
Out[59]:
A B C D F E
2013-01-01 0.000000 0.000000 -1.509059 5 5.0 1.0
2013-01-02 1.212112 -0.173215 0.119209 5 1.0 1.0
2013-01-03 -0.861849 -2.104569 -0.494929 5 2.0 5.0
2013-01-04 0.721555 -0.706771 -1.039575 5 3.0 5.0
isna()
gets the boolean mask where values are nan
:
In [60]: pd.isna(df1)
Out[60]:
A B C D F E
2013-01-01 False False False False True False
2013-01-02 False False False False False False
2013-01-03 False False False False False True
2013-01-04 False False False False False True
Operations#
See the Basic section on Binary Ops.
Stats#
Operations in general exclude missing data.
Performing a descriptive statistic:
In [61]: df.mean()
Out[61]:
A -0.004474
B -0.383981
C -0.687758
D 5.000000
F 3.000000
dtype: float64
Same operation on the other axis:
In [62]: df.mean(1)
Out[62]:
2013-01-01 0.872735
2013-01-02 1.431621
2013-01-03 0.707731
2013-01-04 1.395042
2013-01-05 1.883656
2013-01-06 1.592306
Freq: D, dtype: float64
Operating with objects that have different dimensionality and need alignment. In addition, pandas automatically broadcasts along the specified dimension:
In [63]: s = pd.Series([1, 3, 5, np.nan, 6, 8], index=dates).shift(2)
In [64]: s
Out[64]:
2013-01-01 NaN
2013-01-02 NaN
2013-01-03 1.0
2013-01-04 3.0
2013-01-05 5.0
2013-01-06 NaN
Freq: D, dtype: float64
In [65]: df.sub(s, axis="index")
Out[65]:
A B C D F
2013-01-01 NaN NaN NaN NaN NaN
2013-01-02 NaN NaN NaN NaN NaN
2013-01-03 -1.861849 -3.104569 -1.494929 4.0 1.0
2013-01-04 -2.278445 -3.706771 -4.039575 2.0 0.0
2013-01-05 -5.424972 -4.432980 -4.723768 0.0 -1.0
2013-01-06 NaN NaN NaN NaN NaN
Apply#
DataFrame.apply()
applies a user defined function to the data:
In [66]: df.apply(np.cumsum)
Out[66]:
A B C D F
2013-01-01 0.000000 0.000000 -1.509059 5 NaN
2013-01-02 1.212112 -0.173215 -1.389850 10 1.0
2013-01-03 0.350263 -2.277784 -1.884779 15 3.0
2013-01-04 1.071818 -2.984555 -2.924354 20 6.0
2013-01-05 0.646846 -2.417535 -2.648122 25 10.0
2013-01-06 -0.026844 -2.303886 -4.126549 30 15.0
In [67]: df.apply(lambda x: x.max() - x.min())
Out[67]:
A 2.073961
B 2.671590
C 1.785291
D 0.000000
F 4.000000
dtype: float64
Histogramming#
See more at Histogramming and Discretization.
In [68]: s = pd.Series(np.random.randint(0, 7, size=10))
In [69]: s
Out[69]:
0 4
1 2
2 1
3 2
4 6
5 4
6 4
7 6
8 4
9 4
dtype: int64
In [70]: s.value_counts()
Out[70]:
4 5
2 2
6 2
1 1
dtype: int64
String Methods#
Series is equipped with a set of string processing methods in the str
attribute that make it easy to operate on each element of the array, as in the
code snippet below. Note that pattern-matching in str
generally uses regular
expressions by default (and in
some cases always uses them). See more at Vectorized String Methods.
In [71]: s = pd.Series(["A", "B", "C", "Aaba", "Baca", np.nan, "CABA", "dog", "cat"])
In [72]: s.str.lower()
Out[72]:
0 a
1 b
2 c
3 aaba
4 baca
5 NaN
6 caba
7 dog
8 cat
dtype: object
Merge#
Concat#
pandas provides various facilities for easily combining together Series and DataFrame objects with various kinds of set logic for the indexes and relational algebra functionality in the case of join / merge-type operations.
See the Merging section.
Concatenating pandas objects together along an axis with concat()
:
In [73]: df = pd.DataFrame(np.random.randn(10, 4))
In [74]: df
Out[74]:
0 1 2 3
0 -0.548702 1.467327 -1.015962 -0.483075
1 1.637550 -1.217659 -0.291519 -1.745505
2 -0.263952 0.991460 -0.919069 0.266046
3 -0.709661 1.669052 1.037882 -1.705775
4 -0.919854 -0.042379 1.247642 -0.009920
5 0.290213 0.495767 0.362949 1.548106
6 -1.131345 -0.089329 0.337863 -0.945867
7 -0.932132 1.956030 0.017587 -0.016692
8 -0.575247 0.254161 -1.143704 0.215897
9 1.193555 -0.077118 -0.408530 -0.862495
# break it into pieces
In [75]: pieces = [df[:3], df[3:7], df[7:]]
In [76]: pd.concat(pieces)
Out[76]:
0 1 2 3
0 -0.548702 1.467327 -1.015962 -0.483075
1 1.637550 -1.217659 -0.291519 -1.745505
2 -0.263952 0.991460 -0.919069 0.266046
3 -0.709661 1.669052 1.037882 -1.705775
4 -0.919854 -0.042379 1.247642 -0.009920
5 0.290213 0.495767 0.362949 1.548106
6 -1.131345 -0.089329 0.337863 -0.945867
7 -0.932132 1.956030 0.017587 -0.016692
8 -0.575247 0.254161 -1.143704 0.215897
9 1.193555 -0.077118 -0.408530 -0.862495
Join#
merge()
enables SQL style join types along specific columns. See the Database style joining section.
In [77]: left = pd.DataFrame({"key": ["foo", "foo"], "lval": [1, 2]})
In [78]: right = pd.DataFrame({"key": ["foo", "foo"], "rval": [4, 5]})
In [79]: left
Out[79]:
key lval
0 foo 1
1 foo 2
In [80]: right
Out[80]:
key rval
0 foo 4
1 foo 5
In [81]: pd.merge(left, right, on="key")
Out[81]:
key lval rval
0 foo 1 4
1 foo 1 5
2 foo 2 4
3 foo 2 5
Another example that can be given is:
In [82]: left = pd.DataFrame({"key": ["foo", "bar"], "lval": [1, 2]})
In [83]: right = pd.DataFrame({"key": ["foo", "bar"], "rval": [4, 5]})
In [84]: left
Out[84]:
key lval
0 foo 1
1 bar 2
In [85]: right
Out[85]:
key rval
0 foo 4
1 bar 5
In [86]: pd.merge(left, right, on="key")
Out[86]:
key lval rval
0 foo 1 4
1 bar 2 5
Grouping#
By “group by” we are referring to a process involving one or more of the following steps:
Splitting the data into groups based on some criteria
Applying a function to each group independently
Combining the results into a data structure
See the Grouping section.
In [87]: df = pd.DataFrame(
....: {
....: "A": ["foo", "bar", "foo", "bar", "foo", "bar", "foo", "foo"],
....: "B": ["one", "one", "two", "three", "two", "two", "one", "three"],
....: "C": np.random.randn(8),
....: "D": np.random.randn(8),
....: }
....: )
....:
In [88]: df
Out[88]:
A B C D
0 foo one 1.346061 -1.577585
1 bar one 1.511763 0.396823
2 foo two 1.627081 -0.105381
3 bar three -0.990582 -0.532532
4 foo two -0.441652 1.453749
5 bar two 1.211526 1.208843
6 foo one 0.268520 -0.080952
7 foo three 0.024580 -0.264610
Grouping and then applying the sum()
function to the resulting
groups:
In [89]: df.groupby("A")[["C", "D"]].sum()
Out[89]:
C D
A
bar 1.732707 1.073134
foo 2.824590 -0.574779
Grouping by multiple columns forms a hierarchical index, and again we can
apply the sum()
function:
In [90]: df.groupby(["A", "B"]).sum()
Out[90]:
C D
A B
bar one 1.511763 0.396823
three -0.990582 -0.532532
two 1.211526 1.208843
foo one 1.614581 -1.658537
three 0.024580 -0.264610
two 1.185429 1.348368
Reshaping#
See the sections on Hierarchical Indexing and Reshaping.
Stack#
In [91]: tuples = list(
....: zip(
....: ["bar", "bar", "baz", "baz", "foo", "foo", "qux", "qux"],
....: ["one", "two", "one", "two", "one", "two", "one", "two"],
....: )
....: )
....:
In [92]: index = pd.MultiIndex.from_tuples(tuples, names=["first", "second"])
In [93]: df = pd.DataFrame(np.random.randn(8, 2), index=index, columns=["A", "B"])
In [94]: df2 = df[:4]
In [95]: df2
Out[95]:
A B
first second
bar one -0.727965 -0.589346
two 0.339969 -0.693205
baz one -0.339355 0.593616
two 0.884345 1.591431
The stack()
method “compresses” a level in the DataFrame’s
columns:
In [96]: stacked = df2.stack()
In [97]: stacked
Out[97]:
first second
bar one A -0.727965
B -0.589346
two A 0.339969
B -0.693205
baz one A -0.339355
B 0.593616
two A 0.884345
B 1.591431
dtype: float64
With a “stacked” DataFrame or Series (having a MultiIndex
as the
index
), the inverse operation of stack()
is
unstack()
, which by default unstacks the last level:
In [98]: stacked.unstack()
Out[98]:
A B
first second
bar one -0.727965 -0.589346
two 0.339969 -0.693205
baz one -0.339355 0.593616
two 0.884345 1.591431
In [99]: stacked.unstack(1)
Out[99]:
second one two
first
bar A -0.727965 0.339969
B -0.589346 -0.693205
baz A -0.339355 0.884345
B 0.593616 1.591431
In [100]: stacked.unstack(0)
Out[100]:
first bar baz
second
one A -0.727965 -0.339355
B -0.589346 0.593616
two A 0.339969 0.884345
B -0.693205 1.591431
Pivot tables#
See the section on Pivot Tables.
In [101]: df = pd.DataFrame(
.....: {
.....: "A": ["one", "one", "two", "three"] * 3,
.....: "B": ["A", "B", "C"] * 4,
.....: "C": ["foo", "foo", "foo", "bar", "bar", "bar"] * 2,
.....: "D": np.random.randn(12),
.....: "E": np.random.randn(12),
.....: }
.....: )
.....:
In [102]: df
Out[102]:
A B C D E
0 one A foo -1.202872 0.047609
1 one B foo -1.814470 -0.136473
2 two C foo 1.018601 -0.561757
3 three A bar -0.595447 -1.623033
4 one B bar 1.395433 0.029399
5 one C bar -0.392670 -0.542108
6 two A foo 0.007207 0.282696
7 three B foo 1.928123 -0.087302
8 one C foo -0.055224 -1.575170
9 one A bar 2.395985 1.771208
10 two B bar 1.552825 0.816482
11 three C bar 0.166599 1.100230
pivot_table()
pivots a DataFrame
specifying the values
, index
and columns
In [103]: pd.pivot_table(df, values="D", index=["A", "B"], columns=["C"])
Out[103]:
C bar foo
A B
one A 2.395985 -1.202872
B 1.395433 -1.814470
C -0.392670 -0.055224
three A -0.595447 NaN
B NaN 1.928123
C 0.166599 NaN
two A NaN 0.007207
B 1.552825 NaN
C NaN 1.018601
Time series#
pandas has simple, powerful, and efficient functionality for performing resampling operations during frequency conversion (e.g., converting secondly data into 5-minutely data). This is extremely common in, but not limited to, financial applications. See the Time Series section.
In [104]: rng = pd.date_range("1/1/2012", periods=100, freq="S")
In [105]: ts = pd.Series(np.random.randint(0, 500, len(rng)), index=rng)
In [106]: ts.resample("5Min").sum()
Out[106]:
2012-01-01 24182
Freq: 5T, dtype: int64
Series.tz_localize()
localizes a time series to a time zone:
In [107]: rng = pd.date_range("3/6/2012 00:00", periods=5, freq="D")
In [108]: ts = pd.Series(np.random.randn(len(rng)), rng)
In [109]: ts
Out[109]:
2012-03-06 1.857704
2012-03-07 -1.193545
2012-03-08 0.677510
2012-03-09 -0.153931
2012-03-10 0.520091
Freq: D, dtype: float64
In [110]: ts_utc = ts.tz_localize("UTC")
In [111]: ts_utc
Out[111]:
2012-03-06 00:00:00+00:00 1.857704
2012-03-07 00:00:00+00:00 -1.193545
2012-03-08 00:00:00+00:00 0.677510
2012-03-09 00:00:00+00:00 -0.153931
2012-03-10 00:00:00+00:00 0.520091
Freq: D, dtype: float64
Series.tz_convert()
converts a timezones aware time series to another time zone:
In [112]: ts_utc.tz_convert("US/Eastern")
Out[112]:
2012-03-05 19:00:00-05:00 1.857704
2012-03-06 19:00:00-05:00 -1.193545
2012-03-07 19:00:00-05:00 0.677510
2012-03-08 19:00:00-05:00 -0.153931
2012-03-09 19:00:00-05:00 0.520091
Freq: D, dtype: float64
Converting between time span representations:
In [113]: rng = pd.date_range("1/1/2012", periods=5, freq="M")
In [114]: ts = pd.Series(np.random.randn(len(rng)), index=rng)
In [115]: ts
Out[115]:
2012-01-31 -1.475051
2012-02-29 0.722570
2012-03-31 -0.322646
2012-04-30 -1.601631
2012-05-31 0.778033
Freq: M, dtype: float64
In [116]: ps = ts.to_period()
In [117]: ps
Out[117]:
2012-01 -1.475051
2012-02 0.722570
2012-03 -0.322646
2012-04 -1.601631
2012-05 0.778033
Freq: M, dtype: float64
In [118]: ps.to_timestamp()
Out[118]:
2012-01-01 -1.475051
2012-02-01 0.722570
2012-03-01 -0.322646
2012-04-01 -1.601631
2012-05-01 0.778033
Freq: MS, dtype: float64
Converting between period and timestamp enables some convenient arithmetic functions to be used. In the following example, we convert a quarterly frequency with year ending in November to 9am of the end of the month following the quarter end:
In [119]: prng = pd.period_range("1990Q1", "2000Q4", freq="Q-NOV")
In [120]: ts = pd.Series(np.random.randn(len(prng)), prng)
In [121]: ts.index = (prng.asfreq("M", "e") + 1).asfreq("H", "s") + 9
In [122]: ts.head()
Out[122]:
1990-03-01 09:00 -0.289342
1990-06-01 09:00 0.233141
1990-09-01 09:00 -0.223540
1990-12-01 09:00 0.542054
1991-03-01 09:00 -0.688585
Freq: H, dtype: float64
Categoricals#
pandas can include categorical data in a DataFrame
. For full docs, see the
categorical introduction and the API documentation.
In [123]: df = pd.DataFrame(
.....: {"id": [1, 2, 3, 4, 5, 6], "raw_grade": ["a", "b", "b", "a", "a", "e"]}
.....: )
.....:
Converting the raw grades to a categorical data type:
In [124]: df["grade"] = df["raw_grade"].astype("category")
In [125]: df["grade"]
Out[125]:
0 a
1 b
2 b
3 a
4 a
5 e
Name: grade, dtype: category
Categories (3, object): ['a', 'b', 'e']
Rename the categories to more meaningful names:
In [126]: new_categories = ["very good", "good", "very bad"]
In [127]: df["grade"] = df["grade"].cat.rename_categories(new_categories)
Reorder the categories and simultaneously add the missing categories (methods under Series.cat()
return a new Series
by default):
In [128]: df["grade"] = df["grade"].cat.set_categories(
.....: ["very bad", "bad", "medium", "good", "very good"]
.....: )
.....:
In [129]: df["grade"]
Out[129]:
0 very good
1 good
2 good
3 very good
4 very good
5 very bad
Name: grade, dtype: category
Categories (5, object): ['very bad', 'bad', 'medium', 'good', 'very good']
Sorting is per order in the categories, not lexical order:
In [130]: df.sort_values(by="grade")
Out[130]:
id raw_grade grade
5 6 e very bad
1 2 b good
2 3 b good
0 1 a very good
3 4 a very good
4 5 a very good
Grouping by a categorical column also shows empty categories:
In [131]: df.groupby("grade").size()
Out[131]:
grade
very bad 1
bad 0
medium 0
good 2
very good 3
dtype: int64
Plotting#
See the Plotting docs.
We use the standard convention for referencing the matplotlib API:
In [132]: import matplotlib.pyplot as plt
In [133]: plt.close("all")
The plt.close
method is used to close a figure window:
In [134]: ts = pd.Series(np.random.randn(1000), index=pd.date_range("1/1/2000", periods=1000))
In [135]: ts = ts.cumsum()
In [136]: ts.plot();
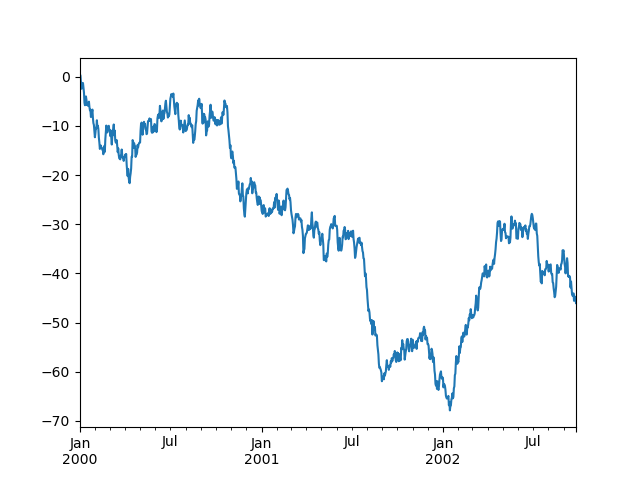
If running under Jupyter Notebook, the plot will appear on plot()
. Otherwise use
matplotlib.pyplot.show to show it or
matplotlib.pyplot.savefig to write it to a file.
In [137]: plt.show();
On a DataFrame, the plot()
method is a convenience to plot all
of the columns with labels:
In [138]: df = pd.DataFrame(
.....: np.random.randn(1000, 4), index=ts.index, columns=["A", "B", "C", "D"]
.....: )
.....:
In [139]: df = df.cumsum()
In [140]: plt.figure();
In [141]: df.plot();
In [142]: plt.legend(loc='best');
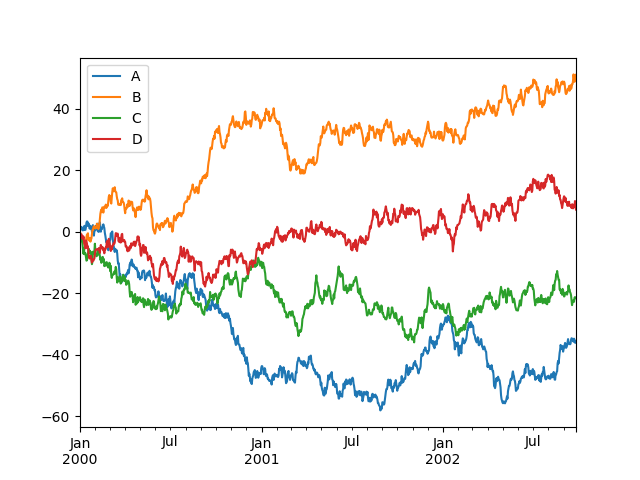
Importing and exporting data#
CSV#
Writing to a csv file: using DataFrame.to_csv()
In [143]: df.to_csv("foo.csv")
Reading from a csv file: using read_csv()
In [144]: pd.read_csv("foo.csv")
Out[144]:
Unnamed: 0 A B C D
0 2000-01-01 0.350262 0.843315 1.798556 0.782234
1 2000-01-02 -0.586873 0.034907 1.923792 -0.562651
2 2000-01-03 -1.245477 -0.963406 2.269575 -1.612566
3 2000-01-04 -0.252830 -0.498066 3.176886 -1.275581
4 2000-01-05 -1.044057 0.118042 2.768571 0.386039
.. ... ... ... ... ...
995 2002-09-22 -48.017654 31.474551 69.146374 -47.541670
996 2002-09-23 -47.207912 32.627390 68.505254 -48.828331
997 2002-09-24 -48.907133 31.990402 67.310924 -49.391051
998 2002-09-25 -50.146062 33.716770 67.717434 -49.037577
999 2002-09-26 -49.724318 33.479952 68.108014 -48.822030
[1000 rows x 5 columns]
HDF5#
Reading and writing to HDFStores.
Writing to a HDF5 Store using DataFrame.to_hdf()
:
In [145]: df.to_hdf("foo.h5", "df")
Reading from a HDF5 Store using read_hdf()
:
In [146]: pd.read_hdf("foo.h5", "df")
Out[146]:
A B C D
2000-01-01 0.350262 0.843315 1.798556 0.782234
2000-01-02 -0.586873 0.034907 1.923792 -0.562651
2000-01-03 -1.245477 -0.963406 2.269575 -1.612566
2000-01-04 -0.252830 -0.498066 3.176886 -1.275581
2000-01-05 -1.044057 0.118042 2.768571 0.386039
... ... ... ... ...
2002-09-22 -48.017654 31.474551 69.146374 -47.541670
2002-09-23 -47.207912 32.627390 68.505254 -48.828331
2002-09-24 -48.907133 31.990402 67.310924 -49.391051
2002-09-25 -50.146062 33.716770 67.717434 -49.037577
2002-09-26 -49.724318 33.479952 68.108014 -48.822030
[1000 rows x 4 columns]
Excel#
Reading and writing to Excel.
Writing to an excel file using DataFrame.to_excel()
:
In [147]: df.to_excel("foo.xlsx", sheet_name="Sheet1")
Reading from an excel file using read_excel()
:
In [148]: pd.read_excel("foo.xlsx", "Sheet1", index_col=None, na_values=["NA"])
Out[148]:
Unnamed: 0 A B C D
0 2000-01-01 0.350262 0.843315 1.798556 0.782234
1 2000-01-02 -0.586873 0.034907 1.923792 -0.562651
2 2000-01-03 -1.245477 -0.963406 2.269575 -1.612566
3 2000-01-04 -0.252830 -0.498066 3.176886 -1.275581
4 2000-01-05 -1.044057 0.118042 2.768571 0.386039
.. ... ... ... ... ...
995 2002-09-22 -48.017654 31.474551 69.146374 -47.541670
996 2002-09-23 -47.207912 32.627390 68.505254 -48.828331
997 2002-09-24 -48.907133 31.990402 67.310924 -49.391051
998 2002-09-25 -50.146062 33.716770 67.717434 -49.037577
999 2002-09-26 -49.724318 33.479952 68.108014 -48.822030
[1000 rows x 5 columns]
Gotchas#
If you are attempting to perform a boolean operation on a Series
or DataFrame
you might see an exception like:
In [149]: if pd.Series([False, True, False]):
.....: print("I was true")
.....:
---------------------------------------------------------------------------
ValueError Traceback (most recent call last)
Cell In[149], line 1
----> 1 if pd.Series([False, True, False]):
2 print("I was true")
File ~/work/pandas/pandas/pandas/core/generic.py:1527, in NDFrame.__nonzero__(self)
1525 @final
1526 def __nonzero__(self) -> NoReturn:
-> 1527 raise ValueError(
1528 f"The truth value of a {type(self).__name__} is ambiguous. "
1529 "Use a.empty, a.bool(), a.item(), a.any() or a.all()."
1530 )
ValueError: The truth value of a Series is ambiguous. Use a.empty, a.bool(), a.item(), a.any() or a.all().
See Comparisons and Gotchas for an explanation and what to do.